What are the biggest risks of AI data theft and how to mitigate them? This question is paramount in today’s data-driven world, where artificial intelligence (AI) systems are increasingly reliant on vast amounts of sensitive information. From unauthorized access to sophisticated malware attacks, the potential for data breaches is significant, impacting not only financial stability but also an organization’s reputation and competitive edge.
Understanding these risks and implementing robust mitigation strategies is crucial for businesses and organizations of all sizes operating in the AI landscape.
The theft of AI data can manifest in various forms, from the pilfering of training datasets to the compromise of model parameters or even intellectual property. This stolen data can be used to create malicious AI systems, replicate existing capabilities, or even provide competitors with an unfair advantage. The financial consequences can be devastating, including loss of revenue, legal battles, and a damaged reputation that erodes customer trust.
Therefore, proactive measures are essential to protect valuable AI assets and maintain a secure operational environment.
Types of AI Data Theft
AI data theft poses a significant threat to organizations leveraging artificial intelligence. The value of the data used to train and operate AI models, coupled with the increasing sophistication of cyberattacks, creates a landscape ripe for exploitation. Understanding the various methods and vulnerabilities is crucial for effective mitigation strategies.
AI data theft can manifest in several ways, impacting different aspects of AI systems. These methods range from direct attacks on databases to more subtle insider threats. The consequences can be severe, leading to financial losses, reputational damage, and even competitive disadvantages. The following table Artikels common methods, their impacts, and typical targets.
Methods and Impacts of AI Data Theft
Method | Description | Impact | Common Targets |
---|---|---|---|
Unauthorized Access | Gaining access to AI data repositories through exploiting vulnerabilities in security systems (e.g., weak passwords, unpatched software) or through phishing attacks. | Data breach, model compromise, intellectual property theft, financial losses, regulatory fines. | Cloud storage, on-premise servers, databases containing training data, model parameters, or intellectual property. |
Insider Threats | Malicious or negligent actions by employees or contractors with access to sensitive AI data. This can involve stealing data, deliberately corrupting models, or leaking information. | Data breach, model compromise, reputational damage, loss of competitive advantage, legal repercussions. | All aspects of AI development and deployment, including training data, models, algorithms, and intellectual property. |
Malware Attacks | Using malicious software to infiltrate systems and steal AI data. This can include ransomware attacks that encrypt data and demand a ransom for its release, or data exfiltration tools that silently copy data. | Data loss or corruption, model compromise, system disruption, financial losses, reputational damage. | Servers, databases, and endpoints used in AI development and deployment. Generative models are particularly vulnerable due to their large datasets. |
Supply Chain Attacks | Compromising third-party vendors or suppliers involved in the AI ecosystem, gaining access to data indirectly. | Data breach, model compromise, disruption of AI operations, reputational damage. | Data providers, cloud service providers, hardware manufacturers involved in AI infrastructure. |
Vulnerabilities of Different AI Models
Different AI models exhibit varying vulnerabilities to data theft. Generative models, known for their ability to produce new content (like text or images), often require vast datasets for training. This makes them prime targets, as the theft of their training data can severely impact their performance or allow for the creation of malicious imitations. Machine learning models, while not always requiring datasets as large as generative models, still rely on sensitive data for training and are vulnerable to attacks that compromise their accuracy or functionality.
Deep learning models, a subset of machine learning, can be particularly susceptible due to their complex architectures and the large amounts of data they process. The theft of model parameters – the internal weights and biases that define a model’s behavior – can allow attackers to replicate or manipulate the model’s functionality without access to the original training data.
Risks of Stealing Training Data vs. Model Parameters
Stealing training data represents a significant risk because it allows attackers to potentially re-train a model, creating a copy or even a superior version of the original. This could lead to the loss of competitive advantage, especially in fields where AI models provide a unique edge. Conversely, stealing model parameters allows attackers to deploy a functional copy of the model without needing the original training data.
This can be less resource-intensive than re-training but still allows for malicious use or competitive advantage. However, the effectiveness of a stolen model may depend on the quality and accessibility of the original training data, which is not available to the attacker in this scenario. The theft of intellectual property, such as the underlying algorithms or architecture of the AI model, is also a serious risk, potentially leading to the development of competing products or services.
The impact of each type of theft varies depending on the specific AI model and its application.
Impact of AI Data Theft: What Are The Biggest Risks Of AI Data Theft And How To Mitigate Them?
The theft of AI data carries significant and far-reaching consequences for organizations, extending beyond the immediate loss of information. The impact reverberates through financial performance, legal battles, and public perception, potentially crippling a company’s ability to compete and thrive. Understanding these repercussions is crucial for developing effective mitigation strategies.The financial ramifications of AI data theft are substantial and multifaceted.
Direct losses can include the cost of investigating the breach, notifying affected parties, and implementing remedial measures. These costs can quickly escalate into the millions, depending on the scale of the theft and the sensitivity of the data. Beyond these direct costs, organizations face potential loss of revenue due to disrupted operations, compromised services, and damage to customer trust.
Legal fees associated with regulatory fines, lawsuits from affected customers, and potential intellectual property litigation can further exacerbate the financial burden. Reputational damage, leading to decreased customer loyalty and investor confidence, can result in long-term financial instability.
Financial Consequences of AI Data Theft
The financial impact of AI data theft is not limited to immediate expenses. Lost revenue stemming from service disruptions, diminished customer trust, and the costs associated with rebuilding damaged systems can significantly impact profitability. For example, a company relying on AI for fraud detection that suffers a data breach could experience a surge in fraudulent transactions, resulting in substantial financial losses.
Furthermore, legal fees related to data breach notifications, regulatory investigations, and potential lawsuits can quickly drain resources. The reputational damage, which can take years to repair, often translates into decreased investor confidence and reduced market valuation. The cumulative effect of these financial impacts can be devastating, potentially leading to bankruptcy in severe cases. Consider the hypothetical case of a fintech company whose proprietary AI trading algorithms are stolen; the resulting loss of competitive advantage and potential for market manipulation could result in millions of dollars in lost revenue and significant legal repercussions.
Intellectual Property Theft and Competitive Advantage
AI models are often the result of significant investment in research and development, representing valuable intellectual property. Theft of this data grants competitors an unfair advantage, allowing them to replicate or improve upon existing technologies without incurring the same development costs. This shortcut can significantly disrupt the market, undermining years of innovation and potentially leading to the demise of the victimized company.
For instance, a pharmaceutical company’s stolen AI model used for drug discovery could enable a competitor to rapidly develop similar medications, capturing market share and reducing the original company’s profits drastically. The stolen data might also reveal critical insights into the victim’s research strategies and future development plans, providing the competitor with a significant head start in the race for innovation.
Creation of Malicious AI Systems
Stolen AI data can be repurposed to create malicious AI systems with potentially devastating consequences. The capabilities of AI are such that this stolen data can be used to build sophisticated tools for fraud, disinformation, and even warfare.
- Deepfakes: Stolen biometric data (facial images, voice recordings) can be used to create highly realistic deepfakes for identity theft, blackmail, or political manipulation. Imagine a deepfake video of a CEO announcing a fraudulent merger, causing significant stock market fluctuations and financial losses.
- Autonomous Weapons: Stolen data related to AI-powered weaponry could be used to enhance the capabilities of existing systems or develop new ones, potentially leading to more lethal and autonomous weapons systems.
- Targeted Malware: Stolen data about user behavior and system vulnerabilities can be used to create highly targeted malware that is more difficult to detect and mitigate.
Mitigation Strategies
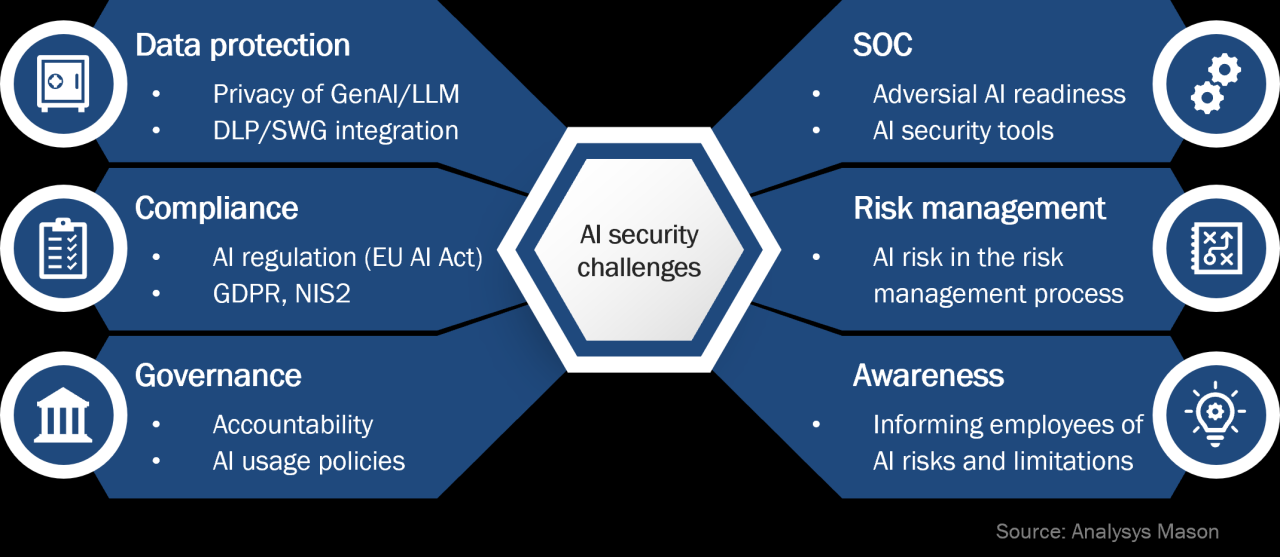
Protecting AI systems and data from theft requires a multi-layered approach encompassing robust security measures, proactive incident response planning, and a culture of security awareness. Effective mitigation hinges on understanding the vulnerabilities within the AI lifecycle, from data acquisition to model deployment, and implementing appropriate safeguards at each stage. Failing to adequately secure AI data can lead to significant financial losses, reputational damage, and legal repercussions.
A comprehensive data security plan is the cornerstone of any effective AI data protection strategy. This plan should be meticulously designed, regularly reviewed, and adapted to address emerging threats and vulnerabilities. It should not be a static document but a living entity that evolves with the changing landscape of cyber threats and the specific needs of the organization.
Data Security Plan Design and Implementation, What are the biggest risks of AI data theft and how to mitigate them?
A robust data security plan incorporates several key elements. Access control measures, such as role-based access control (RBAC), limit access to sensitive data based on an individual’s role and responsibilities. This principle of least privilege ensures that only authorized personnel can access specific data sets and functionalities. Encryption, both at rest and in transit, safeguards data from unauthorized access even if a breach occurs.
This involves using strong encryption algorithms and regularly updating encryption keys. Regular security audits, both internal and external, provide an independent assessment of the effectiveness of the security measures in place, identifying vulnerabilities and areas for improvement. These audits should include penetration testing to simulate real-world attacks and assess the system’s resilience. For example, a financial institution using AI for fraud detection might employ multi-factor authentication, encrypt all transaction data, and conduct quarterly security audits by an independent cybersecurity firm.
Securing AI Model Parameters and Training Data
Protecting AI model parameters and training data throughout the development and deployment lifecycle is critical. During development, version control systems should be used to track changes to models and data, enabling rollback to previous versions in case of compromise. Secure repositories, with access control lists, should be used to store model parameters and training data. Differential privacy techniques can be employed during training to reduce the risk of identifying individuals within the training dataset.
During deployment, model parameters should be protected through encryption and access control mechanisms. Regular monitoring for anomalies in model behavior can also help detect potential compromises. For instance, a company deploying an AI-powered image recognition system might use a secure cloud storage service with encryption at rest and in transit, implement access control based on roles, and continuously monitor the model’s performance for any unexpected deviations.
Data Breach Incident Response Procedure
A well-defined incident response plan is essential for minimizing the impact of a data breach. This plan should Artikel a step-by-step procedure for containing the breach, investigating its cause, and notifying affected parties. The containment phase involves isolating affected systems to prevent further data exfiltration. The investigation phase focuses on identifying the root cause of the breach, the extent of the damage, and the responsible parties.
The notification phase involves informing affected individuals, regulatory bodies, and other relevant stakeholders according to legal and regulatory requirements. For example, a healthcare provider experiencing a breach of patient data would immediately isolate affected servers, launch a forensic investigation to determine the source and scope of the breach, and then notify patients, the relevant authorities, and potentially the media, depending on the severity and scope of the data loss.
This procedure must be tested regularly through simulations to ensure its effectiveness.
Mitigation Strategies
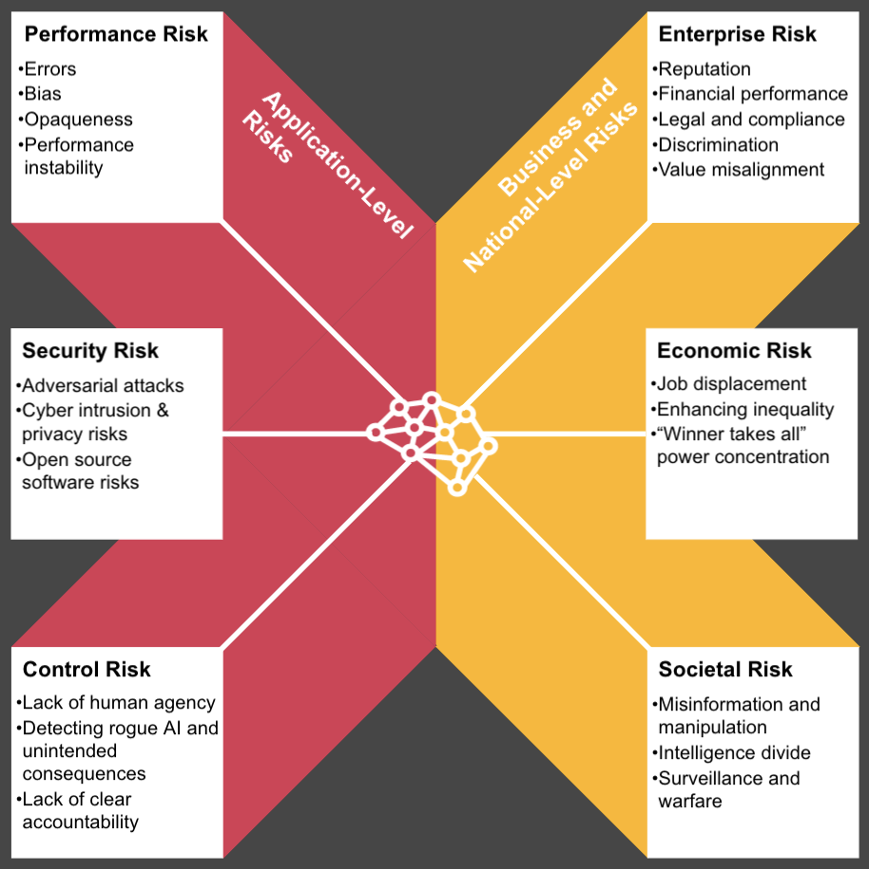
Protecting AI systems and data from theft requires a multi-faceted approach encompassing robust technological safeguards and equally strong personnel and process controls. Neglecting the human element significantly weakens even the most sophisticated security measures. A strong security culture, fostered through comprehensive training and stringent access controls, is crucial for minimizing the risk of data breaches originating from within an organization.Effective mitigation strategies must address human vulnerabilities and procedural weaknesses.
This includes rigorous background checks, comprehensive training programs, and clearly defined access control policies. By focusing on these critical areas, organizations can significantly reduce the likelihood of successful AI data theft attempts.
Employee Training on Data Security Best Practices
A comprehensive training program is essential to equip employees with the knowledge and skills necessary to identify and avoid data security threats. This program should encompass various aspects of data security, with a particular emphasis on the most common attack vectors. For example, employees need to understand the characteristics of phishing emails and social engineering tactics, enabling them to recognize and report suspicious activity.
Training should also cover the importance of strong password management and the risks associated with using unsecured Wi-Fi networks or personal devices for accessing company data. Regular refresher training and simulated phishing exercises can reinforce learning and maintain a high level of vigilance. Furthermore, the training should clearly Artikel the consequences of data breaches and the company’s disciplinary procedures for violations of security protocols.
Background Checks and Security Clearances
Individuals with access to sensitive AI data should undergo thorough background checks and, depending on the sensitivity of the data, security clearances. This process aims to identify any potential risks associated with granting access to sensitive information. Background checks may include criminal history checks, credit checks, and verification of educational credentials and employment history. Security clearances, typically employed in government and highly regulated industries, involve more extensive investigations and vetting processes.
The level of scrutiny should be commensurate with the sensitivity of the AI data and the potential impact of a breach. For example, an employee working on a project involving military-grade AI would require a much higher level of security clearance than an employee working on a less sensitive project.
Best Practices for Managing Access Control to AI Systems and Data
Effective access control is paramount in preventing unauthorized access to AI systems and data. A well-defined access control policy should be implemented and rigorously enforced. This includes the principle of least privilege, granting individuals only the minimum level of access required to perform their duties. Regular audits of access permissions should be conducted to identify and revoke unnecessary access rights.
- Implement multi-factor authentication (MFA) for all access points to AI systems and data.
- Utilize role-based access control (RBAC) to manage user permissions based on their roles and responsibilities.
- Regularly review and update access control lists (ACLs) to ensure they accurately reflect current roles and responsibilities.
- Employ strong password policies, including password complexity requirements and regular password changes.
- Monitor system logs for suspicious activity and promptly investigate any anomalies.
- Segment networks to isolate sensitive AI data from other systems.
- Establish a clear incident response plan to address security breaches effectively.
Mitigation Strategies
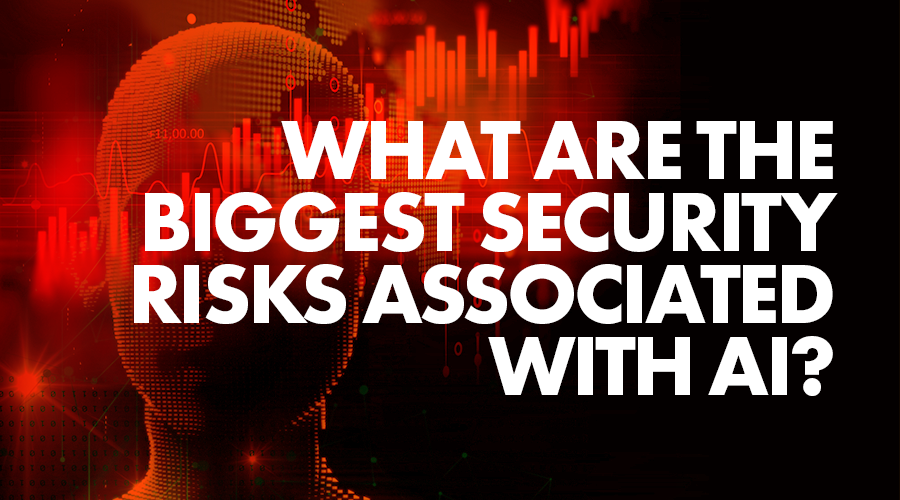
Protecting AI data from theft requires a multi-layered approach encompassing robust security technologies, diligent monitoring, and proactive responses to adversarial attacks. Effective mitigation strategies are crucial not only for safeguarding valuable intellectual property but also for maintaining the integrity and trustworthiness of AI systems. The following sections detail technological solutions and implementation strategies for enhanced AI data security.
Technological Mitigation Strategies
Several technologies can significantly enhance the security of AI data. The choice of technology depends on the specific needs and context of the AI system, including the sensitivity of the data, the scale of the operation, and the level of threat. The following table summarizes key technologies and their attributes.
Technology | Function | Advantages | Disadvantages |
---|---|---|---|
Intrusion Detection Systems (IDS) | Monitor network traffic and system activity for malicious behavior, identifying potential data breaches. | Early detection of threats, reduced impact of successful attacks, improved overall system security. | Can generate false positives, requires skilled personnel for analysis and response, may not detect all types of attacks. |
Data Loss Prevention (DLP) Tools | Prevent sensitive AI data from leaving the controlled environment, monitoring data transfers and blocking unauthorized access. | Reduces the risk of data exfiltration, enforces data security policies, provides granular control over data access. | Can be complex to implement and manage, may interfere with legitimate data transfers, requires careful configuration to avoid hindering productivity. |
Blockchain Technology | Provides a secure, transparent, and tamper-proof record of data access and modifications, enhancing data provenance and accountability. | Increased data integrity, improved auditability, enhanced trust and transparency. | Can be computationally expensive, requires specialized expertise to implement, scalability challenges for large datasets. |
Homomorphic Encryption | Allows computations to be performed on encrypted data without decryption, protecting data confidentiality during processing. | Enhanced data privacy during model training and inference, reduces the risk of data breaches during computation. | Computationally intensive, limited functionality compared to unencrypted computations, ongoing research to improve efficiency and practicality. |
Federated Learning | Trains AI models on decentralized data sources without exchanging the raw data, improving data privacy and security. | Enhanced data privacy, reduced risk of data breaches, enables collaboration on sensitive data without compromising confidentiality. | Slower training process compared to centralized learning, requires careful coordination between participating parties, potential challenges in data heterogeneity. |
Robust Monitoring and Logging
Implementing robust monitoring and logging systems is critical for detecting suspicious activity related to AI data. This involves continuous monitoring of system logs, network traffic, and user activity for anomalies indicative of malicious behavior. Real-time alerts can be configured to notify security personnel of potential threats. Detailed logs should record all data access attempts, modifications, and transfers, providing valuable forensic evidence in case of a breach.
For example, a sudden surge in data access requests from an unusual IP address could indicate a potential intrusion attempt. Furthermore, regular log analysis can identify trends and patterns that may indicate emerging threats.
Detecting and Responding to Adversarial Attacks
Adversarial attacks aim to manipulate AI models by introducing subtly altered inputs that cause the model to produce incorrect or unintended outputs. Detecting these attacks requires sophisticated techniques, including anomaly detection, model robustness testing, and adversarial training. Anomaly detection algorithms can identify unusual patterns in model inputs or outputs that may indicate an attack. Robustness testing involves evaluating the model’s performance under various adversarial conditions, identifying vulnerabilities and improving resilience.
Adversarial training involves exposing the model to adversarial examples during training, enhancing its ability to withstand attacks. A robust incident response plan is crucial for effectively handling adversarial attacks, including containment, eradication, and recovery measures. For example, if an adversarial attack is detected, the affected AI model may need to be temporarily disabled, the source of the attack identified and neutralized, and the model retrained or updated with improved defenses.
Legal and Regulatory Considerations
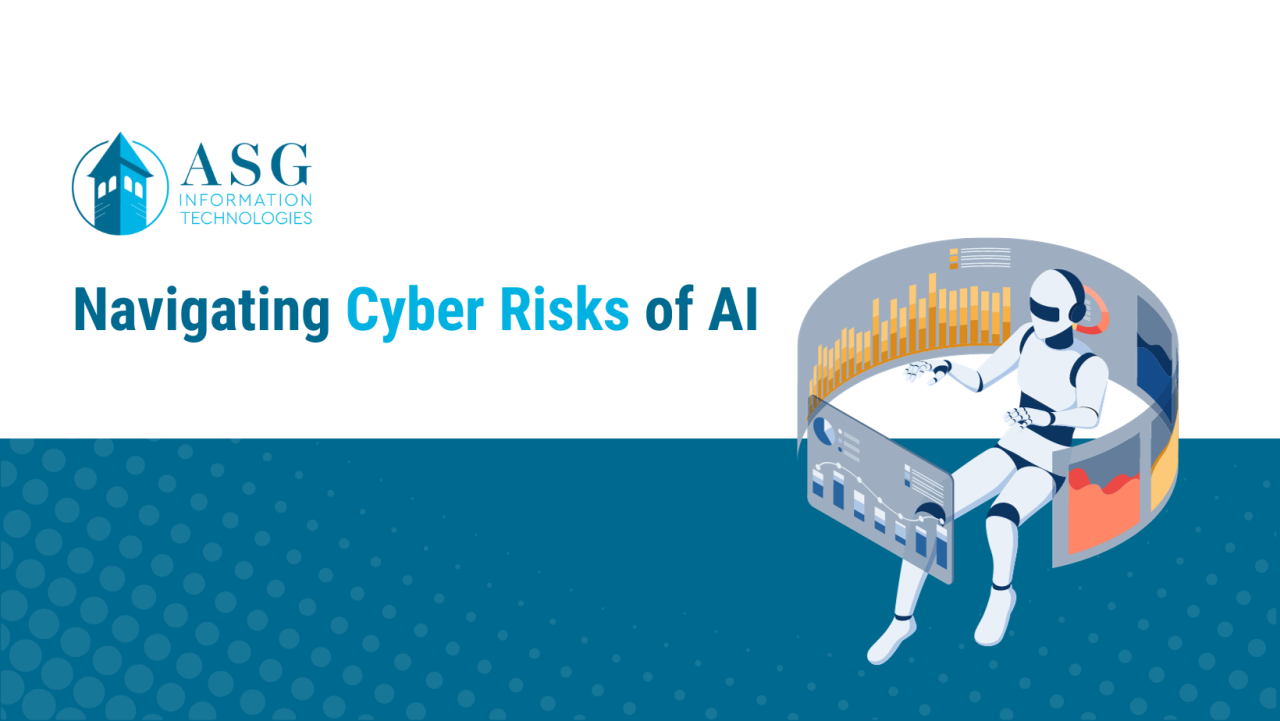
The theft of AI data carries significant legal and financial ramifications, demanding a thorough understanding of applicable regulations and potential liabilities. Navigating this complex landscape requires proactive measures to ensure compliance and minimize exposure to legal action. Failure to comply can result in substantial fines, reputational damage, and loss of customer trust.The legal landscape surrounding AI data is constantly evolving, but several key regulations already impact organizations handling sensitive information used for AI development and deployment.
Understanding these regulations and their implications is crucial for responsible AI development and deployment.
Data Privacy Regulations and Laws
Numerous international and national laws govern the collection, use, and protection of personal data. The General Data Protection Regulation (GDPR) in Europe and the California Consumer Privacy Act (CCPA) in the United States are prominent examples. GDPR imposes stringent requirements on organizations processing personal data of EU residents, including the right to access, rectification, erasure, and data portability.
Similarly, CCPA grants California residents specific rights concerning their personal information, including the right to know what data is collected, the right to delete data, and the right to opt-out of the sale of personal data. Other regional and national laws, such as the Brazilian LGPD, also impose significant obligations regarding data protection. Compliance with these regulations is paramount to avoid legal penalties and maintain ethical data handling practices.
Organizations must implement robust data governance frameworks and conduct thorough data protection impact assessments to ensure compliance.
Legal Liabilities Associated with AI Data Breaches
AI data breaches can lead to a range of legal liabilities, including hefty fines for non-compliance with data protection regulations like GDPR and CCPA. Beyond regulatory penalties, organizations face potential lawsuits from individuals whose data has been compromised. These lawsuits could involve claims for damages resulting from identity theft, financial losses, reputational harm, or emotional distress. Furthermore, organizations might face class-action lawsuits if a breach affects a large number of individuals.
The legal consequences can be severe, extending beyond financial penalties to include reputational damage and loss of business. Proactive risk management, including robust security measures and incident response plans, is essential to mitigate these liabilities.
The Role of Insurance in Mitigating Financial Risks
Cyber insurance plays a crucial role in mitigating the financial risks associated with AI data theft. Comprehensive cyber insurance policies can cover various expenses related to a data breach, including legal fees, regulatory fines, notification costs, credit monitoring services for affected individuals, and potential compensation for data subjects. Selecting the appropriate coverage level is critical, taking into account the organization’s size, the sensitivity of the data it handles, and the potential impact of a breach.
It is important to carefully review policy terms and conditions to ensure adequate coverage for AI-specific risks. A well-structured cyber insurance policy can significantly reduce the financial burden associated with data theft and facilitate a faster recovery process. The insurance policy should also cover the costs associated with investigating and responding to a breach, including forensic analysis and public relations management.
Future Trends and Challenges
The landscape of AI data security is constantly evolving, presenting both new opportunities and significant challenges. As AI systems become more sophisticated and interconnected, the potential for data breaches and exploitation increases exponentially. Emerging technologies and attack vectors demand a proactive and adaptive approach to safeguarding valuable AI datasets.The future of AI data security will be defined by a complex interplay of technological advancements, evolving regulatory landscapes, and the ingenuity of both attackers and defenders.
Predicting the exact trajectory is difficult, but several key trends are already emerging that will significantly shape this domain.
Quantum Computing’s Threat to AI Data Security
Quantum computing, while still in its nascent stages, poses a significant long-term threat to current cryptographic methods used to protect AI data. Quantum computers possess the potential to break widely used encryption algorithms, such as RSA and ECC, rendering current security measures ineffective. This would allow malicious actors to decrypt sensitive AI training data, intellectual property embedded within AI models, and even compromise the functionality of AI systems themselves.
For instance, a quantum computer could potentially break the encryption protecting a self-driving car’s sensor data, leading to significant safety risks. The development of quantum-resistant cryptography is crucial to mitigating this future threat. Research into post-quantum cryptography algorithms, such as lattice-based cryptography and code-based cryptography, is vital for ensuring the long-term security of AI data.
Advanced AI Attacks on AI Systems
The use of AI to attack AI systems is a rapidly growing concern. Sophisticated adversarial attacks can manipulate AI models by introducing carefully crafted inputs that cause them to produce incorrect or malicious outputs. These attacks can target various aspects of AI systems, from manipulating training data to compromising the model’s inference process. For example, a malicious actor could use an adversarial attack to inject false data into a facial recognition system, causing it to misidentify individuals.
Similarly, an autonomous vehicle’s navigation system could be compromised by an adversarial attack on its image recognition capabilities. Developing robust defense mechanisms against these advanced AI attacks is critical to ensuring the reliability and security of AI systems. This involves techniques such as adversarial training, which involves exposing AI models to adversarial examples during training to improve their robustness.
AI-Enhanced AI Data Security
Paradoxically, advancements in AI itself can be leveraged to enhance AI data security. AI can be employed to detect anomalies, predict potential threats, and automate security responses. For example, AI-powered intrusion detection systems can analyze network traffic and identify suspicious activity in real-time. Similarly, AI can be used to monitor AI models for signs of compromise or manipulation, providing early warning of potential attacks.
Furthermore, AI can be utilized to automate the process of patching vulnerabilities and implementing security updates, improving the overall security posture of AI systems. The development and deployment of these AI-powered security solutions are crucial for staying ahead of evolving threats. Companies like Google and Microsoft are already actively investing in this area, developing sophisticated AI-driven security tools to protect their own AI infrastructure and the data it handles.
Final Wrap-Up
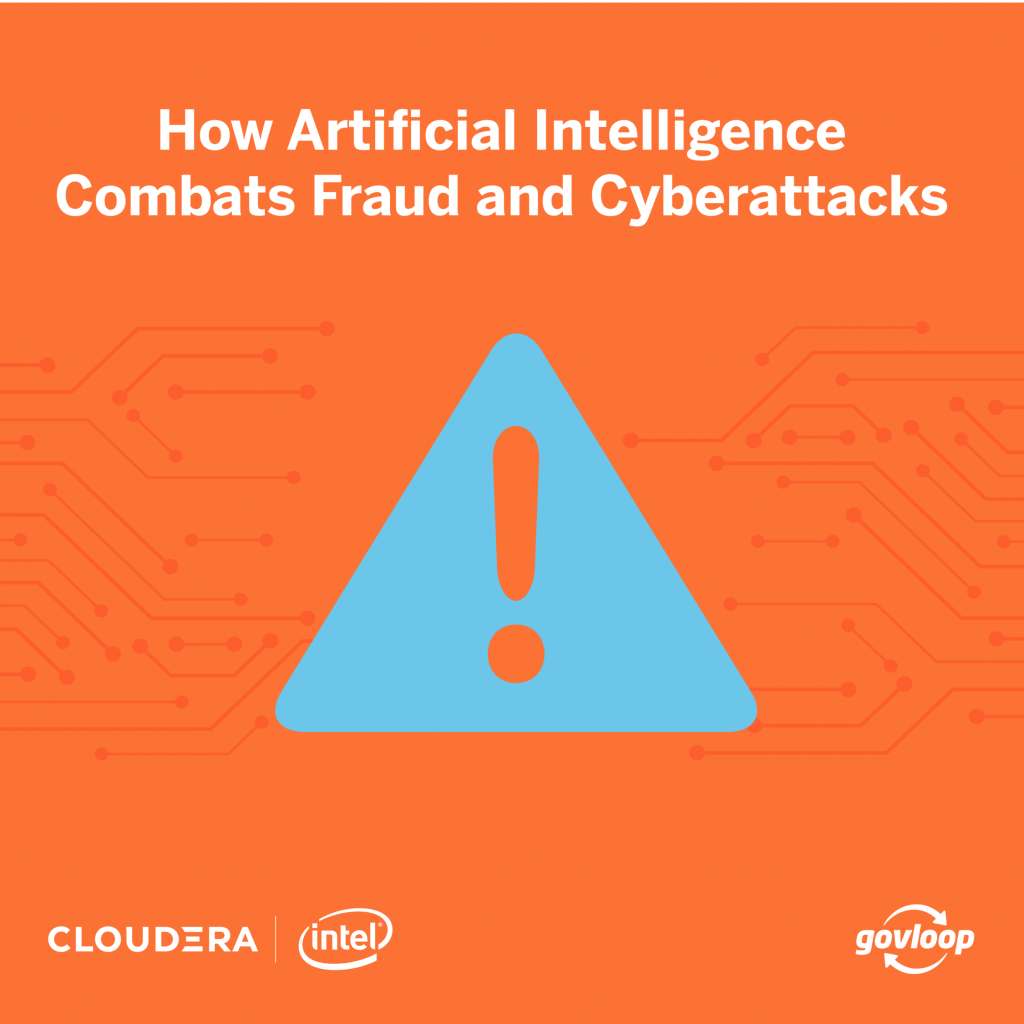
Securing AI data requires a multi-faceted approach encompassing robust data security protocols, rigorous personnel training, advanced security technologies, and a thorough understanding of relevant legal and regulatory frameworks. While the evolving landscape of AI presents new challenges, such as quantum computing threats and increasingly sophisticated adversarial attacks, advancements in AI itself can also be leveraged to bolster security measures.
By proactively addressing the risks and implementing comprehensive mitigation strategies, organizations can safeguard their AI investments, maintain a competitive advantage, and build trust with stakeholders in this ever-evolving technological landscape.