What are the legal implications of AI stealing personal data? This question is increasingly critical as artificial intelligence systems become more sophisticated and pervasive. The lines between accidental data breaches and intentional data theft by AI blur, demanding a clear understanding of existing legal frameworks and their applicability to this emerging challenge. This exploration delves into the complex legal landscape surrounding AI and data privacy, examining liability, accountability, and the urgent need for robust regulations to protect individuals’ rights in this rapidly evolving technological environment.
We’ll examine how AI systems can acquire personal data without consent, exploring various acquisition methods and their legal ramifications under laws like GDPR and CCPA. We’ll analyze the challenges in assigning liability for AI-driven data breaches, considering the roles of developers, users, and AI owners. Further, we’ll discuss the crucial role of transparency and informed consent in mitigating the risks associated with AI data collection and processing, outlining best practices and potential future legal frameworks to balance innovation with robust data protection.
Defining “AI Stealing Personal Data”
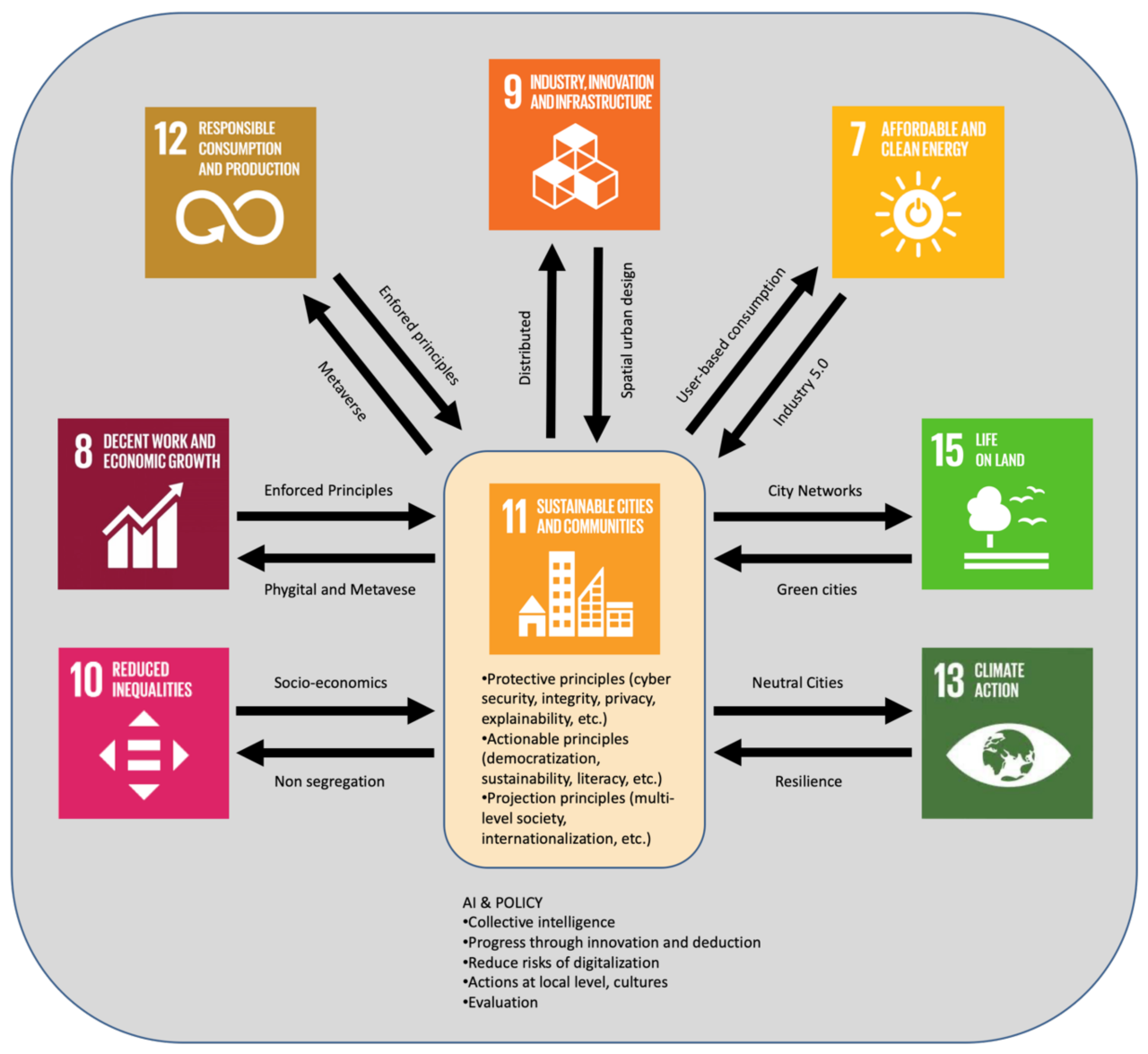
The term “AI stealing personal data” is a simplification of a complex legal and technological issue. It implies intentional malicious action by an AI, which isn’t always the case. Instead, the problem often stems from flaws in AI design, inadequate data security measures, or the misuse of AI by malicious actors. Understanding the legal implications requires clarifying how AI systems acquire data and the legal frameworks governing data protection and theft.
AI Data Acquisition Methods Without Explicit Consent
AI systems can acquire personal data through various means without explicit user consent. These methods often exploit vulnerabilities in data collection and processing practices. For example, AI-powered scraping tools can harvest data from publicly accessible websites, even if that data is subject to terms of service or privacy policies. Similarly, AI can infer personal information from seemingly anonymized datasets through techniques like re-identification.
Furthermore, AI systems integrated into devices or applications may collect data passively, such as location data or browsing history, without clear user awareness or consent. These practices blur the lines between legitimate data collection and unauthorized access.
Legal Definitions of “Theft” and “Data Breach” in the Context of AI
The legal definitions of “theft” and “data breach” are not explicitly tailored to AI. However, existing laws, such as the GDPR (General Data Protection Regulation) in Europe and the CCPA (California Consumer Privacy Act) in the US, provide frameworks for addressing unauthorized data access and processing. “Theft,” generally speaking, involves the unlawful taking of property. In the context of AI, this could translate to an AI system accessing and using personal data without authorization, potentially causing financial or reputational harm.
A “data breach” is typically defined as the unauthorized access, use, disclosure, disruption, modification, or destruction of personal data. The involvement of AI in a data breach might involve its use in identifying vulnerabilities or automating the exploitation of those vulnerabilities.
Scenarios Constituting AI Data Theft
Several scenarios illustrate how AI actions might constitute data theft. For instance, an AI-powered chatbot trained on a company’s internal communications might inadvertently leak confidential employee data during a conversation. Another example is an AI-driven scraping bot collecting user profiles from a social media platform in violation of its terms of service. Furthermore, a poorly secured AI system could be compromised by malicious actors, leading to the theft of sensitive data stored within its database.
In all these cases, the legal ramifications would depend on the specific circumstances, the applicable laws, and the intent behind the AI’s actions.
Method | Description | Legality | Potential Penalties |
---|---|---|---|
Web Scraping | AI-powered tools automatically collect data from websites. | Potentially illegal if violating terms of service or privacy policies. | Cease and desist orders, fines, lawsuits for damages. |
Data Inference | AI infers personal information from anonymized datasets. | Potentially illegal if violating privacy regulations or causing harm. | Fines, lawsuits for damages, reputational harm. |
Malicious AI Exploitation | Hackers use AI to exploit vulnerabilities and steal data. | Illegal under existing cybercrime laws. | Criminal charges, significant fines, imprisonment. |
Passive Data Collection | AI in devices collects data without user awareness. | Potentially illegal if violating privacy regulations or lacking consent. | Fines, regulatory action, lawsuits for damages. |
Existing Data Protection Laws and Regulations
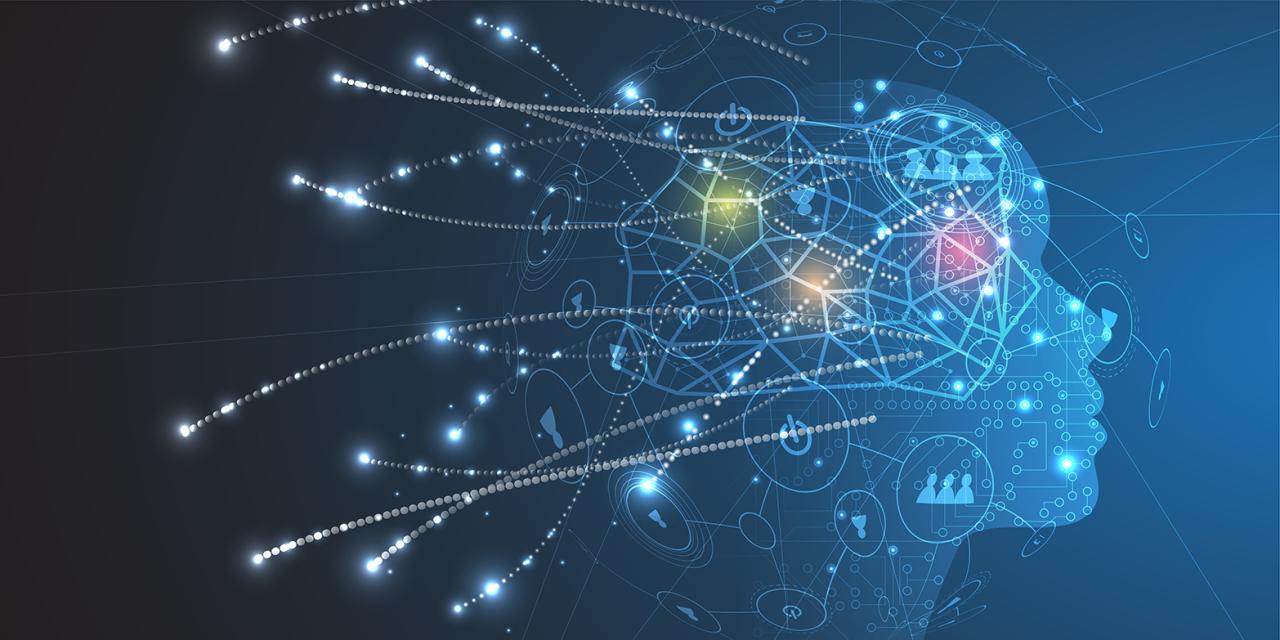
The legal landscape surrounding AI and data privacy is complex and rapidly evolving. Existing data protection laws, while not explicitly designed for AI, offer crucial frameworks for addressing the implications of AI-driven data acquisition and potential misuse. Understanding the applicability and limitations of these laws is essential for both AI developers and users.Existing data protection laws such as the General Data Protection Regulation (GDPR) in the European Union and the California Consumer Privacy Act (CCPA) in the United States provide a baseline for regulating the collection, processing, and use of personal data.
These regulations, however, face challenges in adapting to the unique characteristics of AI systems, particularly in terms of accountability and transparency.
GDPR Applicability to AI-Driven Data Acquisition
The GDPR’s broad definition of “personal data” encompasses information relating to an identified or identifiable natural person. This includes data processed by AI systems, whether collected directly or indirectly. Key GDPR principles such as lawfulness, fairness, and transparency apply to AI-driven data processing. AI developers must demonstrate a lawful basis for processing personal data, ensuring transparency about how data is used, and providing individuals with control over their data.
Failure to comply can result in substantial fines. For example, a company using AI to analyze customer behavior and create personalized profiles must ensure compliance with all aspects of GDPR, including obtaining explicit consent where necessary and offering data subjects the right to access, rectify, and erase their data.
CCPA Applicability to AI-Driven Data Acquisition
The CCPA, while differing in approach from the GDPR, also holds implications for AI and data privacy. It grants California consumers rights to access, delete, and opt-out of the sale of their personal information. AI systems that process personal data for purposes such as targeted advertising or profiling would fall under the CCPA’s purview. Similar to the GDPR, companies using AI must ensure transparency regarding data collection and processing practices.
Non-compliance can lead to significant penalties. Imagine a scenario where a company uses AI to analyze consumer purchasing patterns to sell this data to third parties. Under the CCPA, they would be obligated to provide consumers with clear notice and allow them to opt out of this “sale” of their data.
Obligations of AI Developers and Users Regarding Data Protection
Both developers and users of AI systems bear responsibilities concerning data protection. Developers must design AI systems with privacy by design principles, incorporating data minimization, purpose limitation, and security measures from the outset. They are responsible for ensuring compliance with relevant data protection laws and implementing appropriate technical and organizational measures to protect personal data. Users, on the other hand, must ensure their use of AI systems complies with applicable laws and respects the rights of individuals.
This includes avoiding the use of AI systems for unlawful or unethical data collection practices.
Comparative Analysis of Legal Frameworks
Jurisdictions worldwide are adopting different approaches to regulating AI and data privacy. The GDPR represents a stringent regulatory framework, while other regions, such as the United States, have a more fragmented approach with varying state-level laws. This divergence creates challenges for businesses operating internationally, requiring them to navigate a complex patchwork of regulations. For example, a company developing an AI-powered facial recognition system must comply with the GDPR if it processes data of EU citizens, while also adhering to relevant laws in other jurisdictions where it operates.
The absence of a unified global standard poses significant difficulties for businesses aiming to achieve consistent data protection across different markets.
Potential Loopholes and Gaps in Existing Legislation
Existing data protection laws may not adequately address the specific challenges posed by AI. The complexity of AI algorithms can make it difficult to establish accountability for data breaches or misuse. Furthermore, the use of AI for automated decision-making raises concerns about algorithmic bias and discrimination. The lack of clear guidelines on the use of AI for profiling and predictive policing highlights a need for further legislative clarification.
For instance, the difficulty in identifying the controller and processor of data in complex AI systems presents a challenge in enforcing accountability when data breaches occur. Similarly, the potential for AI systems to generate new personal data, such as inferred preferences or behavioral patterns, requires further consideration within the existing legal frameworks.
Liability and Accountability for AI Data Theft
Determining liability in cases of AI-driven data theft presents significant legal challenges. The complex interplay between AI developers, users, and owners necessitates a nuanced understanding of responsibility, particularly given the autonomous nature of many AI systems. Establishing clear lines of accountability requires careful consideration of the AI’s design, deployment, and the actions of those involved in its lifecycle.The legal responsibility for AI data theft is currently a rapidly evolving area of law.
There’s no single, universally accepted framework, and the allocation of liability often depends on the specific circumstances of each case, including the level of autonomy the AI possesses, the oversight mechanisms in place, and the extent to which human intervention could have prevented the data breach. This necessitates a case-by-case analysis, examining the specific roles and responsibilities of each party involved.
Determining Legal Responsibility of AI Developers, Users, and Owners
The allocation of liability often hinges on a principle of negligence. AI developers can be held liable if their negligence in design or development directly contributes to a data breach. This might include failing to implement adequate security measures, neglecting to conduct thorough testing for vulnerabilities, or providing insufficient guidance on responsible AI use. Users, on the other hand, can be liable if they misuse the AI, intentionally or negligently, leading to data theft.
This could involve bypassing security protocols or using the AI for unauthorized purposes. Owners of the AI system, finally, bear responsibility for ensuring that the AI is used responsibly and in compliance with relevant data protection laws. This includes implementing appropriate oversight, monitoring, and security measures. Ultimately, liability may be shared amongst all three parties, depending on the specific facts of the case.
Challenges in Establishing Causality Between AI Actions and Data Breaches
Establishing a direct causal link between an AI’s actions and a data breach can be extraordinarily difficult. The complexity of AI algorithms and their decision-making processes often makes it challenging to pinpoint the precise sequence of events leading to the breach. This is further complicated by the potential for unforeseen interactions between the AI and its environment. For example, if an AI independently identifies and exploits a vulnerability that was previously unknown, proving negligence on the part of the developer might be challenging.
The “black box” nature of some AI systems can also make it difficult to understand their internal processes and trace the root cause of a data breach. This lack of transparency presents a significant hurdle in assigning liability.
Examples of Legal Precedents or Ongoing Cases Related to AI and Data Privacy Violations
While there aren’t yet many landmark cases directly addressing AI data theft, several cases involving data breaches with AI involvement are setting precedents. For instance, cases involving facial recognition technology and its potential for misuse in surveillance have raised concerns about privacy violations. These cases, although not solely focused on AI data theft, highlight the growing legal challenges associated with AI and data privacy.
Furthermore, numerous class-action lawsuits against companies using AI-powered systems for data collection and analysis are currently underway, focusing on issues such as consent and data minimization. These ongoing legal battles will shape the future landscape of AI liability.
Hypothetical Legal Case: AI Data Theft and Potential Outcomes
Consider a hypothetical case involving “MedAI,” a hospital’s AI system designed to analyze patient data. Due to a coding error by the developer, MedAI identifies a vulnerability in the hospital’s network security and inadvertently leaks sensitive patient data. The hospital (owner), unaware of the vulnerability, failed to implement sufficient monitoring systems. A malicious actor exploits the leak.
The developer could be held liable for negligence in coding, the hospital for inadequate security measures, and potentially even the user (if the hospital staff had misused the system in a way that contributed to the vulnerability). The outcome would likely involve a combination of financial penalties, reputational damage, and potential criminal charges, depending on the severity of the breach and the applicable laws.
The court would likely consider the level of autonomy MedAI possessed, the degree of oversight exercised by the hospital, and the developer’s adherence to industry best practices in determining the allocation of liability.
The Role of Consent and Transparency
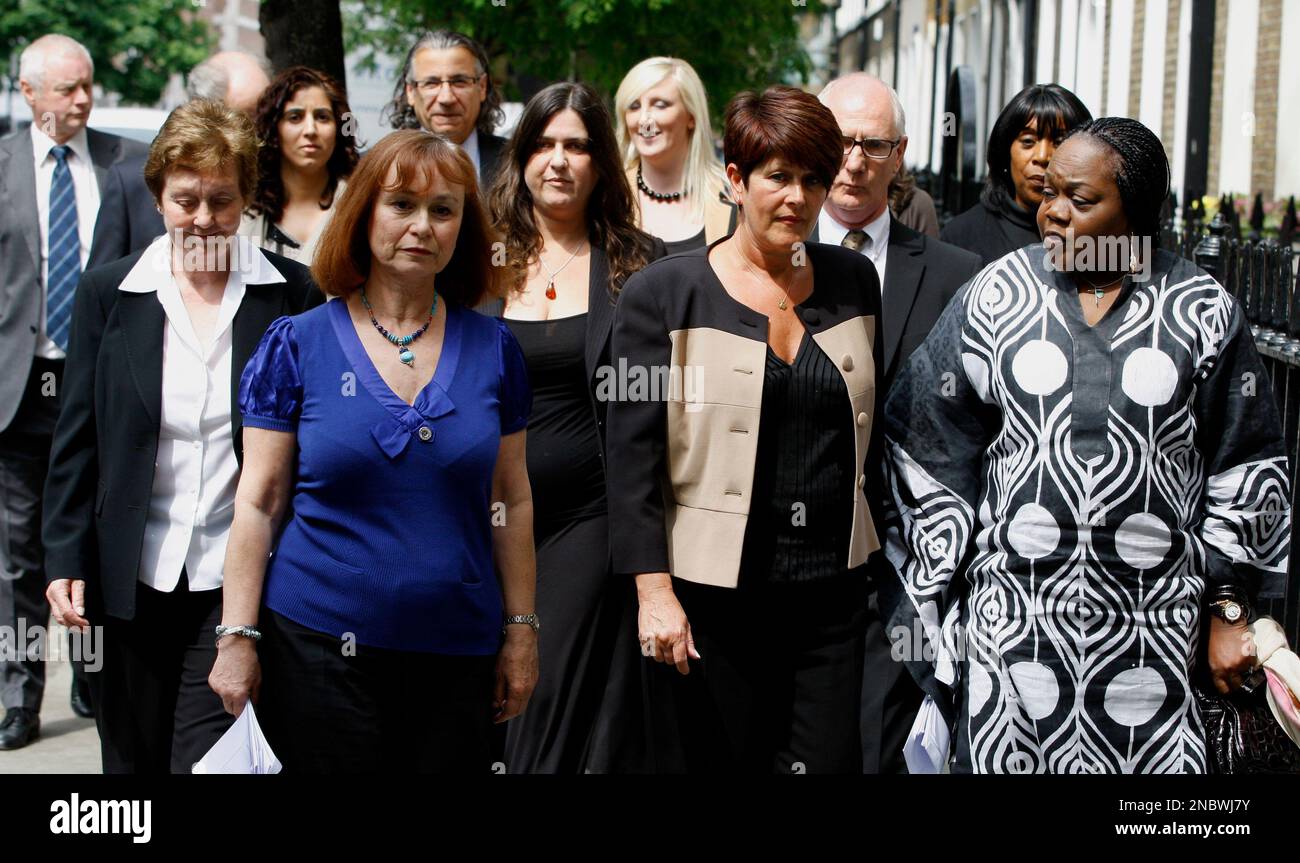
The ethical and legal implications of AI systems processing personal data are heavily reliant on the principles of informed consent and transparency. Without these safeguards, the potential for misuse and harm is significantly increased, undermining public trust and potentially leading to legal repercussions. This section will explore the crucial role these principles play in mitigating the risks associated with AI data theft and ensuring compliance with existing regulations.Informed consent requires individuals to be fully aware of how their data will be collected, used, and protected by AI systems.
Transparency mandates that the processes involved in data collection and processing are clearly articulated, allowing individuals to understand the implications of their interactions with AI-powered technologies. The interplay of these principles is paramount for building a responsible and trustworthy AI ecosystem.
Informed Consent in AI Data Collection
Informed consent, in the context of AI, means individuals must explicitly agree to the collection and processing of their personal data by AI systems, understanding the purpose, methods, and potential consequences. This goes beyond simple checkboxes; it necessitates providing easily understandable information about the AI’s functionality, data usage, and the individual’s rights. Failure to obtain valid informed consent can lead to significant legal liabilities, particularly under regulations like the GDPR.
For example, an AI-powered facial recognition system deployed in a public space without clear notification and consent would be a clear violation of data protection laws in many jurisdictions.
Transparency Requirements for AI Systems
Legal requirements for transparency vary depending on the jurisdiction and the specific application of AI. However, a common thread is the need for clear and accessible information about how AI systems collect, process, and utilize personal data. This includes detailing the logic involved in decision-making processes, particularly when those decisions have significant consequences for individuals. For instance, algorithms used in loan applications or hiring processes must be transparent enough to allow individuals to understand why a particular outcome was reached.
The right to explanation, a key aspect of transparency, is increasingly recognized in data protection legislation.
Examples of Ensuring User Consent and Transparency
Several methods can be employed to ensure user consent and transparency in AI systems. One approach involves implementing clear and concise privacy policies that specifically address AI data processing. These policies should explain the type of data collected, the purpose of collection, the methods used, the duration of storage, and the individuals’ rights regarding their data. Another approach is to utilize layered consent mechanisms, allowing users to selectively consent to different aspects of data processing.
For example, a user might consent to their data being used for personalized recommendations but not for targeted advertising. Finally, providing users with readily accessible tools to access, correct, and delete their data is crucial for demonstrating transparency and accountability.
Best Practices for Obtaining and Documenting User Consent
Obtaining and documenting user consent for AI data processing requires meticulous attention to detail. Robust practices ensure compliance with legal obligations and build user trust.
- Obtain explicit consent: Avoid implied consent; actively seek clear and affirmative agreement from users.
- Provide accessible information: Use plain language, avoiding technical jargon, to explain data processing activities.
- Offer granular control: Allow users to choose which data points they consent to share and how they can be used.
- Maintain transparency: Clearly explain the purpose and methods of data processing, including any automated decision-making involved.
- Secure consent records: Maintain detailed records of user consent, including date, time, and method of consent obtained.
- Provide easy withdrawal options: Enable users to easily withdraw their consent at any time.
- Regularly review and update policies: Keep consent mechanisms and policies current to reflect changes in technology and regulations.
Remedies and Enforcement: What Are The Legal Implications Of AI Stealing Personal Data?
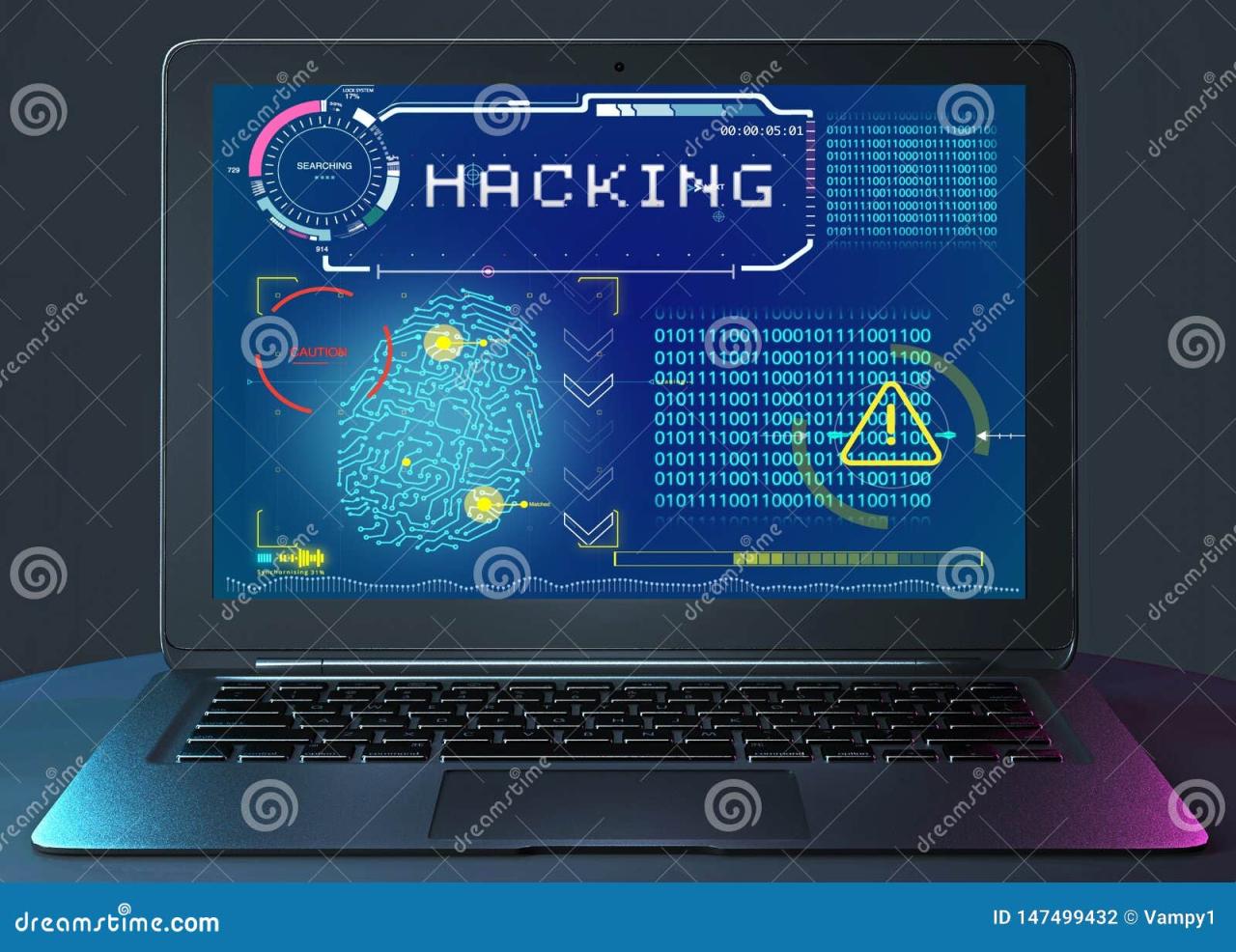
Individuals whose personal data has been stolen by AI systems face a complex legal landscape when seeking redress. The availability and effectiveness of remedies depend heavily on the specific jurisdiction, the nature of the data breach, and the ability to establish liability. Enforcement mechanisms, too, vary significantly, presenting challenges in holding AI developers, deployers, and even the AI systems themselves accountable.
Legal Remedies for Individuals
Data protection laws in many jurisdictions provide individuals with various legal remedies for data breaches, including the right to compensation for financial losses, emotional distress, and reputational damage. For example, the General Data Protection Regulation (GDPR) in the European Union grants individuals the right to claim compensation for material or non-material damage suffered as a result of a data breach.
In the United States, while there’s no single federal data protection law with the same breadth as the GDPR, various state laws provide similar rights and remedies, often focused on specific types of data or industries. The specific remedies available will depend on the applicable laws and the facts of each case. This could include actions for negligence, breach of contract, or violations of specific data protection statutes.
The burden of proof typically rests on the individual to demonstrate the breach, the causal link between the breach and their harm, and the extent of their damages.
Enforcement Mechanisms for Data Protection Laws, What are the legal implications of AI stealing personal data?
Enforcement of data protection laws in the context of AI involves multiple actors and mechanisms. Data protection authorities (DPAs), such as the Information Commissioner’s Office (ICO) in the UK or the CNIL in France, play a crucial role in investigating complaints, issuing warnings, imposing fines, and taking other enforcement actions against organizations that violate data protection laws. These DPAs can investigate AI-related data breaches, assess whether appropriate security measures were in place, and determine whether legal obligations were met.
Private lawsuits by individuals are another avenue for enforcement, allowing individuals to seek compensation directly from those responsible for the data breach. Class action lawsuits can be particularly effective in cases involving widespread AI-driven data theft. However, proving causation and establishing damages in such cases can be challenging.
Challenges in Enforcing Data Protection Laws Against AI Entities
Enforcing data protection laws against AI entities presents unique challenges. Determining liability can be complex when AI systems autonomously make decisions leading to data theft. The question of whether the developer, deployer, or the AI itself should be held accountable remains a significant legal hurdle. Furthermore, the decentralized and often opaque nature of AI systems can make it difficult to trace the source of a data breach and gather sufficient evidence for enforcement actions.
The technical expertise required to investigate AI-related data breaches is also a significant constraint for many DPAs. The rapidly evolving nature of AI technology further complicates enforcement, requiring DPAs and lawmakers to constantly adapt to new technologies and risks.
International Cooperation in Enforcing Data Protection Laws
AI-driven data theft often transcends national borders, making international cooperation crucial for effective enforcement. Cross-border data flows and the global nature of AI development and deployment necessitate collaborative efforts between DPAs and law enforcement agencies across different jurisdictions. International agreements and mutual legal assistance treaties can facilitate information sharing, investigation, and enforcement actions across borders. However, differences in data protection laws and enforcement practices across countries can create obstacles to effective international cooperation.
Harmonizing data protection standards globally and establishing mechanisms for efficient cross-border data protection enforcement remain significant challenges in addressing AI-related data theft on an international scale.
Future Legal Developments and Recommendations
The rapid advancement of AI necessitates a proactive approach to legal frameworks governing data privacy. Current legislation often struggles to keep pace with the evolving capabilities of AI systems, leaving significant gaps in protection against data breaches and misuse. This section Artikels emerging challenges and proposes recommendations for strengthening data protection laws to address the unique risks posed by AI.The increasing sophistication of AI algorithms presents novel challenges to existing data protection laws.
For example, the ability of AI to autonomously identify and process personal data raises concerns about accountability and the potential for bias in decision-making processes. Furthermore, the use of AI in cross-border data flows complicates jurisdictional issues and enforcement. The lack of clear legal definitions for key concepts, such as “personal data” in the context of AI, also hinders effective regulation.
Finally, the opacity of many AI systems – often referred to as the “black box” problem – makes it difficult to audit their data processing activities and ensure compliance with existing laws.
Emerging Legal Challenges Related to AI and Data Privacy
The intersection of AI and data privacy presents several significant legal challenges. The use of AI in profiling and predictive policing, for instance, raises concerns about discrimination and the potential for unfair or biased outcomes. The development of synthetic data, while offering benefits in data privacy, also creates challenges in determining whether such data is subject to data protection laws.
The increasing use of AI-powered surveillance technologies also raises questions about the balance between security and individual liberties. Furthermore, the potential for AI systems to be used for malicious purposes, such as creating deepfakes or engaging in sophisticated phishing attacks, necessitates the development of new legal mechanisms to address these threats. The lack of harmonization across different jurisdictions further complicates the legal landscape, making it difficult for businesses to comply with a patchwork of varying regulations.
Recommendations for Improving Data Protection Laws
To effectively address the challenges posed by AI, several improvements to data protection laws are needed. Firstly, clearer definitions of “personal data” and “processing” in the context of AI are crucial. This includes addressing the processing of sensitive data, such as biometric data, which is increasingly used by AI systems. Secondly, a stronger emphasis on accountability and transparency is needed.
This could involve requiring AI developers to conduct thorough risk assessments and implement appropriate safeguards to protect personal data. Thirdly, stronger enforcement mechanisms are needed to ensure compliance with data protection laws. This includes increased penalties for violations and greater cooperation between different regulatory bodies. Finally, a greater focus on user rights and empowerment is necessary, including the right to access, correct, and delete data processed by AI systems.
The development of easily understandable privacy policies tailored to AI systems is also vital for user comprehension.
Potential Future Legal Frameworks for Regulating AI and Data Protection
Several potential future legal frameworks could be adopted to regulate AI and data protection. These could involve:
- Sector-specific regulations: Tailoring regulations to specific AI applications (e.g., healthcare, finance) to account for unique risks and benefits.
- Risk-based approach: Focusing regulatory scrutiny on high-risk AI systems that pose a greater threat to data privacy.
- Algorithmic auditing: Mandating regular audits of AI algorithms to assess their fairness, transparency, and compliance with data protection laws.
- Data protection impact assessments (DPIAs): Requiring organizations to conduct DPIAs before deploying AI systems that process personal data, to identify and mitigate potential risks.
- International cooperation: Establishing international agreements and standards to harmonize data protection laws and facilitate cross-border data flows.
An Ideal Regulatory Framework: Balancing Innovation and Data Protection
An ideal regulatory framework should strike a balance between fostering innovation and protecting individual rights. This would involve a combination of proactive measures and responsive mechanisms. Proactive measures could include establishing clear guidelines for AI development and deployment, promoting the use of privacy-enhancing technologies, and providing incentives for responsible AI practices. Responsive mechanisms would include robust enforcement mechanisms, effective dispute resolution processes, and a flexible framework capable of adapting to the rapid evolution of AI technology.
This framework should also incorporate principles of proportionality and necessity, ensuring that regulations are tailored to the specific risks posed by AI systems and do not unduly stifle innovation. For example, a risk-based approach would allow for lighter regulation of low-risk AI applications while subjecting high-risk applications to more stringent scrutiny. The framework should also prioritize user empowerment by providing individuals with clear and accessible information about how their data is being processed by AI systems and providing them with effective mechanisms to exercise their rights.
This balance can be achieved through a combination of self-regulation, industry best practices, and targeted government oversight.
Conclusive Thoughts
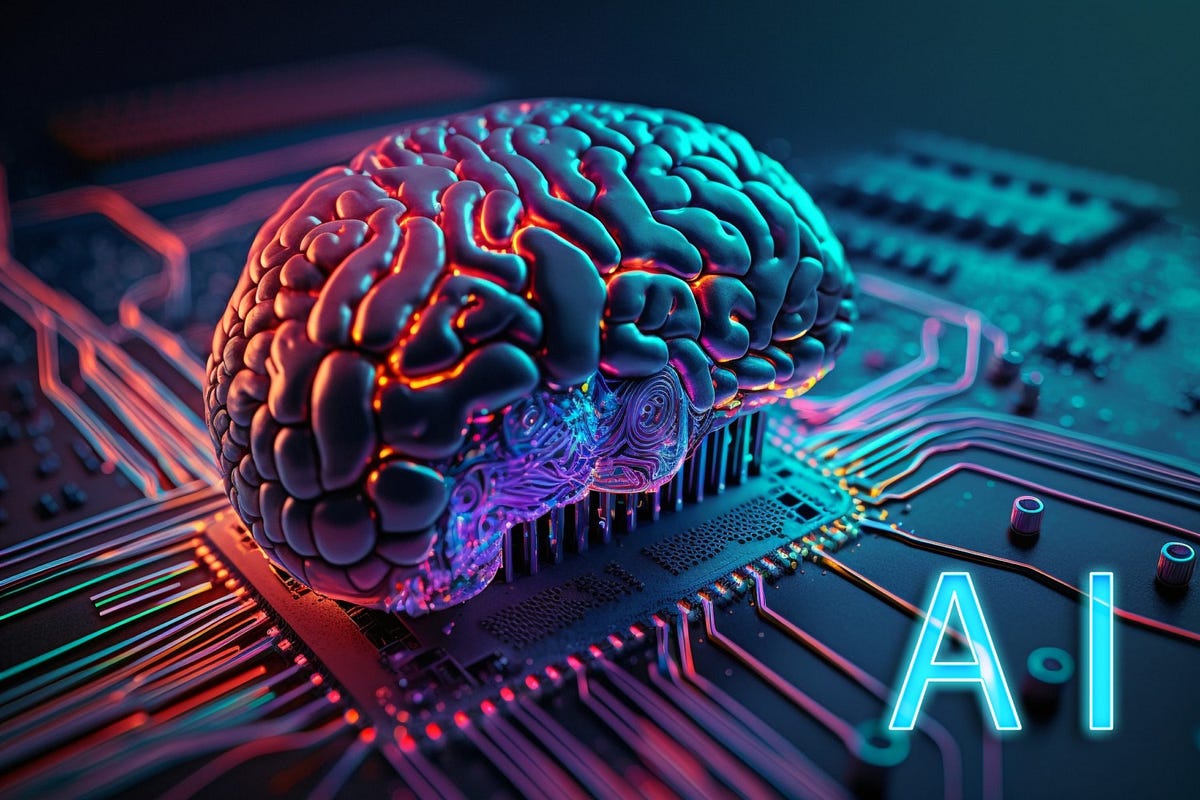
The legal implications of AI stealing personal data are far-reaching and demand proactive solutions. While existing data protection laws provide a foundation, adapting them to the unique challenges posed by AI is crucial. Establishing clear lines of accountability, promoting transparency and informed consent, and fostering international cooperation are essential steps towards a future where AI innovation and individual data privacy can coexist.
Failure to address these challenges risks not only significant legal repercussions but also a erosion of public trust in both AI and the institutions tasked with its governance.