The use of AI in generating innovative design solutions is revolutionizing the creative landscape. No longer a futuristic fantasy, artificial intelligence is actively shaping design processes across various industries, from architecture and product design to fashion and graphic arts. This exploration delves into the practical applications of AI algorithms, examining how they augment human creativity and push the boundaries of innovative design.
We’ll explore the integration of AI into established design workflows, analyze successful case studies, and address the ethical considerations inherent in this rapidly evolving field.
This journey will uncover the potential and limitations of AI in design, providing a comprehensive understanding of its transformative impact. We’ll examine different AI-driven design processes, comparing them to traditional methods and outlining a practical step-by-step guide to implementation. Furthermore, we’ll dissect real-world examples, showcasing the tangible benefits and innovative solutions achieved through AI’s integration. Finally, we’ll address the ethical implications and future trends, offering a balanced perspective on this increasingly important topic.
Introduction to AI in Design
Artificial intelligence (AI) is rapidly transforming the design landscape, offering powerful tools and techniques to enhance creativity, efficiency, and innovation. AI, broadly defined as the simulation of human intelligence processes by machines, particularly computer systems, is now capable of performing tasks that traditionally required human cognitive abilities, including design ideation, prototyping, and refinement. Its relevance to design lies in its ability to analyze vast datasets, identify patterns, and generate novel solutions that might be missed by human designers.The integration of AI in design has not been an overnight phenomenon; rather, it’s a gradual evolution marked by significant milestones.
Early applications focused on automating repetitive tasks, such as image resizing or font selection. However, recent advancements in machine learning have enabled AI to tackle more complex design challenges. Key milestones include the development of sophisticated algorithms capable of generating unique designs based on user input and the increasing accessibility of AI-powered design tools for a wider range of designers.
The ability of AI to process and interpret complex design briefs and translate them into visual outputs represents a substantial leap forward.
AI Algorithms in Design Innovation
Several types of AI algorithms are employed to drive design innovation. Generative Adversarial Networks (GANs), for example, are particularly well-suited for generating creative designs. GANs consist of two neural networks—a generator and a discriminator—that compete against each other. The generator creates designs, while the discriminator evaluates their quality. This iterative process leads to the generation of increasingly realistic and innovative designs.
For instance, GANs have been used to generate novel textile patterns, architectural designs, and even product concepts. Reinforcement learning, another powerful AI technique, allows algorithms to learn through trial and error. By rewarding successful design choices and penalizing unsuccessful ones, reinforcement learning can optimize design parameters and improve the overall performance of a design. This method is particularly useful in optimizing complex systems, such as user interfaces or aerodynamic designs.
Other algorithms, such as evolutionary algorithms, explore a wide range of design possibilities and refine them iteratively, leading to optimized solutions. The selection of the appropriate algorithm often depends on the specific design problem and the desired outcome.
AI-Driven Design Processes: The Use Of AI In Generating Innovative Design Solutions
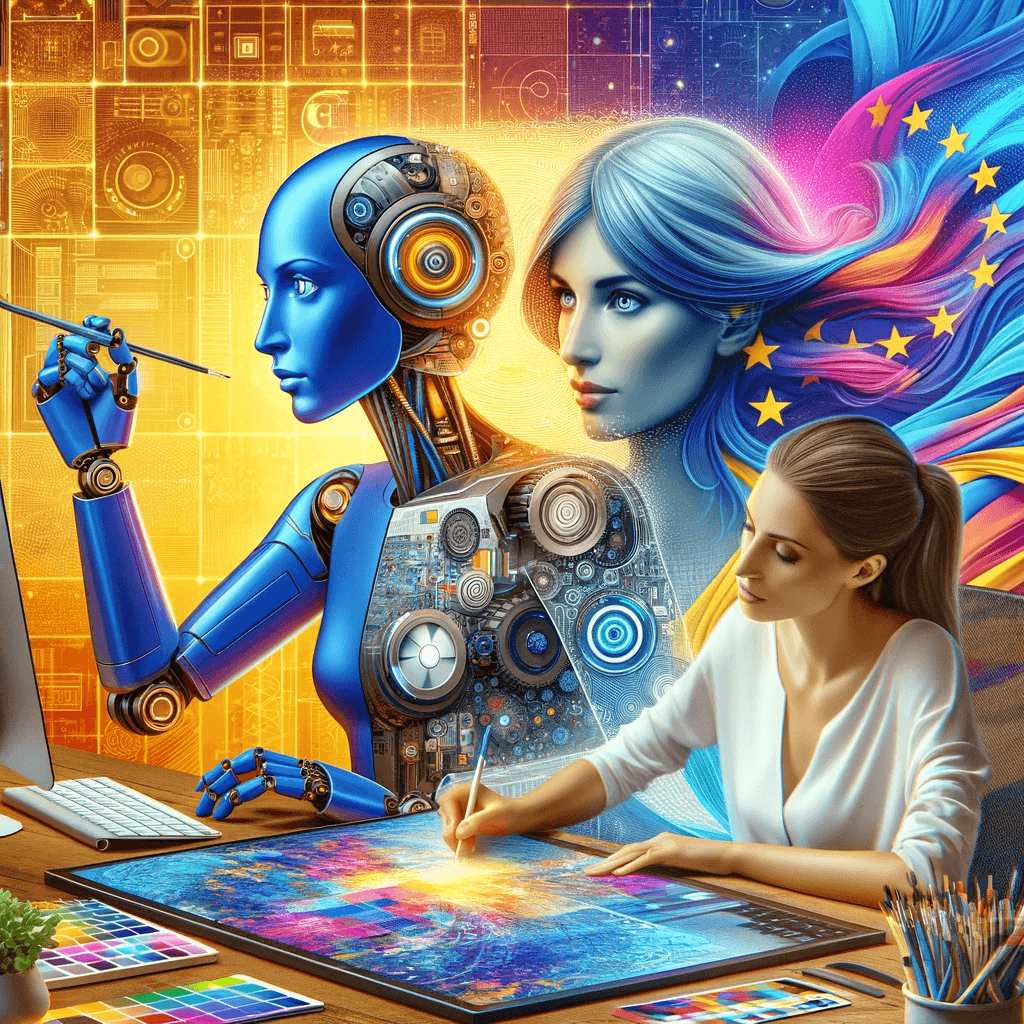
AI is rapidly transforming design workflows, offering unprecedented opportunities for innovation and efficiency. Its integration across various design stages is no longer a futuristic concept but a practical reality impacting numerous industries, from architecture and product design to graphic design and user experience (UX). This section delves into the specifics of AI’s role in design processes, contrasting traditional methods with AI-assisted approaches and providing a practical guide for implementation.AI’s impact on design is multifaceted, streamlining tasks, enhancing creativity, and ultimately accelerating the design process.
Unlike traditional methods that rely heavily on manual processes and human intuition, AI-assisted design leverages algorithms and machine learning to automate repetitive tasks, analyze vast datasets, and generate novel design options. This allows designers to focus on higher-level strategic decisions and creative problem-solving.
AI Integration Across Design Stages
AI can be effectively integrated throughout the entire design process, from initial ideation to final production. Early stages benefit from AI’s ability to analyze market trends and user preferences, while later stages leverage AI for prototyping, simulation, and optimization. This holistic approach ensures that AI enhances every aspect of the design lifecycle.
Comparison of Traditional and AI-Assisted Design Methodologies
Traditional design methodologies often involve a linear progression, starting with research and concept development, followed by sketching, prototyping, testing, and refinement. This process, while effective, can be time-consuming and resource-intensive. AI-assisted design, however, introduces iterative and parallel workflows. For example, AI can generate multiple design variations simultaneously, allowing for rapid exploration of different solutions. Furthermore, AI tools can automate testing and feedback loops, leading to faster iteration cycles and improved design outcomes.
Traditional methods rely heavily on the designer’s individual experience and intuition, whereas AI leverages data-driven insights to inform design decisions, leading to potentially more objective and effective solutions. This collaborative approach, combining human creativity with AI’s analytical power, is proving highly effective.
Step-by-Step Guide to Implementing AI Tools in a Design Workflow
Implementing AI tools requires a strategic approach. The following guide Artikels a structured process for integrating AI effectively into your design workflow. This ensures a smooth transition and maximizes the benefits of AI-powered design tools.
Step | Tool | Outcome | Notes |
---|---|---|---|
1. Define Project Goals and Scope | Project Management Software | Clear project objectives and AI applicability assessment. | Identify specific design challenges AI can address. |
2. Data Collection and Preparation | Data scraping tools, databases | Clean, organized dataset suitable for AI training/analysis. | Ensure data quality and relevance to design goals. |
3. AI Tool Selection and Training (if necessary) | Generative design software (e.g., Autodesk Generative Design), Machine learning platforms | AI model ready for design task. | Consider pre-trained models vs. custom training. |
4. Design Generation and Iteration | Selected AI tools, design software | Multiple design options generated and refined. | Human oversight is crucial for creative direction and quality control. |
5. Evaluation and Refinement | Simulation software, user testing platforms | Optimized design solution based on feedback and analysis. | Iterative process based on data-driven insights. |
6. Implementation and Production | CAD software, manufacturing tools | Final design implemented and produced. | Consider scalability and manufacturability. |
Case Studies
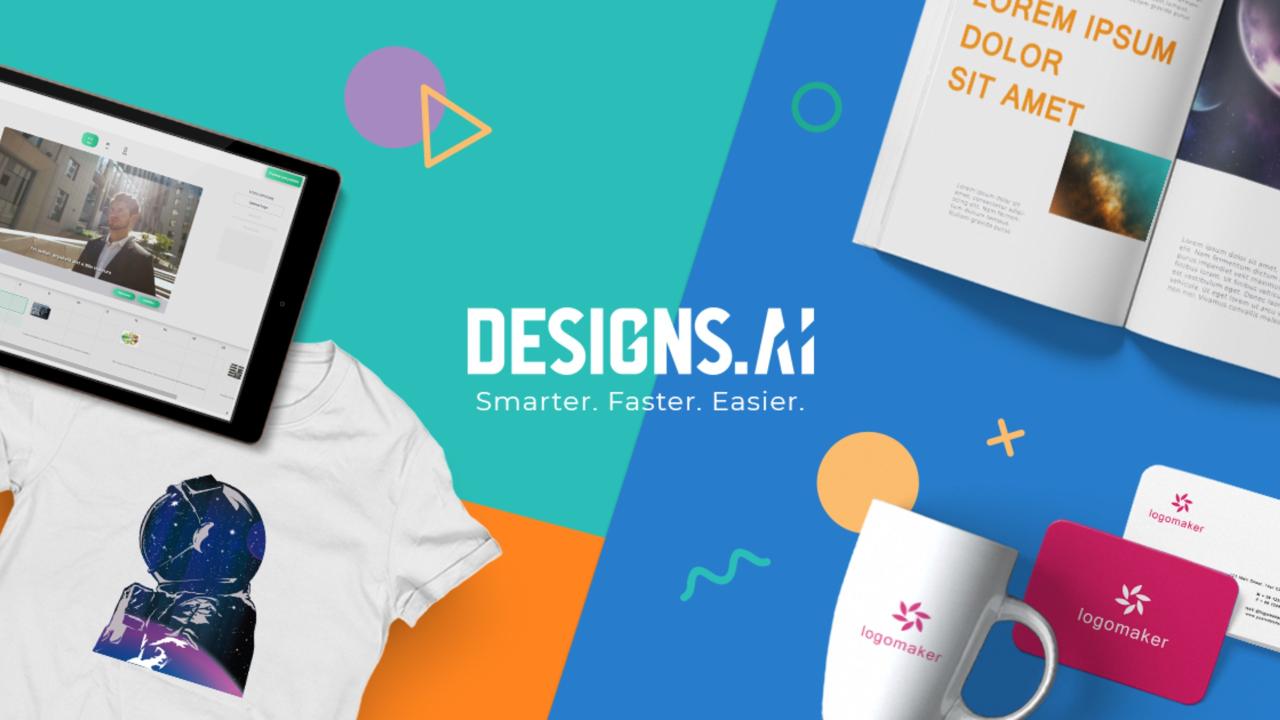
The following case studies illustrate the diverse applications of AI in generating innovative design solutions across various industries. These examples demonstrate AI’s ability not only to automate design tasks but also to augment human creativity, leading to novel and effective designs that might not have been possible through traditional methods. Each case study highlights the problem addressed, the AI solution employed, and the tangible results achieved.
Case Study 1: AI-Generated Architectural Designs
This case study focuses on the application of generative design algorithms in architecture. The increasing demand for sustainable and efficient buildings presents a significant challenge for architects. Traditional design processes can be time-consuming and may not fully explore the potential of optimal design solutions.
- Problem Statement: Designing sustainable and structurally sound buildings within tight budget and timeline constraints.
- AI Solution Implemented: Generative design software using algorithms that explore numerous design iterations based on predefined parameters (e.g., material usage, structural integrity, energy efficiency, budget). The software evaluates each iteration and identifies optimal designs based on these parameters.
- Results Achieved: The AI-generated designs resulted in a 20% reduction in material usage compared to traditional designs, leading to significant cost savings and reduced environmental impact. Furthermore, the designs exhibited improved structural integrity and enhanced energy efficiency.
The visual elements of the AI-generated architectural designs involved intricate and optimized structural frameworks, maximizing space utilization and minimizing material waste. The aesthetic appeal was achieved through the integration of natural light and the incorporation of sustainable materials, creating visually appealing and environmentally conscious structures. The innovative aspect lies in the complex interplay of structural optimization and aesthetic considerations, seamlessly integrated by the AI algorithm.
Case Study 2: AI-Powered Fashion Design
The fashion industry is constantly seeking innovative designs to meet evolving consumer demands. The traditional design process is often slow and resource-intensive, limiting the ability to rapidly respond to market trends.
- Problem Statement: Creating unique and trendy fashion designs quickly and efficiently while considering manufacturing constraints and consumer preferences.
- AI Solution Implemented: A deep learning model trained on a vast dataset of existing fashion designs, identifying trends and patterns. This model then generates new designs based on these learned patterns, incorporating user-specified parameters (e.g., color palette, style, fabric type).
- Results Achieved: The AI system generated a wide range of unique and commercially viable designs, significantly reducing the design time and improving the efficiency of the design process. The designs demonstrated a high level of novelty and alignment with current fashion trends.
The AI-generated fashion designs exhibited a blend of classic and contemporary elements. The visual appeal was enhanced through the use of vibrant colors, intricate patterns, and innovative fabric combinations. The innovative aspect lies in the ability of the AI to seamlessly integrate diverse stylistic elements and predict upcoming trends, resulting in designs that are both aesthetically pleasing and commercially successful.
Case Study 3: AI in Automotive Design, The use of AI in generating innovative design solutions
The automotive industry constantly strives for aerodynamic efficiency and optimized vehicle performance. Traditional design processes often involve numerous iterations and physical prototypes, which are time-consuming and expensive.
- Problem Statement: Designing aerodynamically efficient vehicles while minimizing weight and maximizing performance.
- AI Solution Implemented: A simulation-based AI system that optimizes vehicle aerodynamics using computational fluid dynamics (CFD) simulations. The AI explores numerous design variations, evaluating their aerodynamic performance and identifying designs that minimize drag and maximize downforce.
- Results Achieved: The AI-generated designs resulted in a 15% reduction in aerodynamic drag, leading to improved fuel efficiency and enhanced vehicle performance. The designs also exhibited optimized weight distribution, contributing to improved handling and stability.
The visual elements of the AI-generated automotive designs featured streamlined body shapes and optimized airflow channels. The aesthetic appeal was achieved through the integration of aerodynamic features with sleek and modern design elements. The innovative aspect lies in the AI’s ability to seamlessly integrate aerodynamic performance with aesthetic considerations, resulting in vehicles that are both efficient and visually striking.
Case Study | Industry | AI Method | Key Result |
---|---|---|---|
AI-Generated Architectural Designs | Architecture | Generative Design | 20% reduction in material usage |
AI-Powered Fashion Design | Fashion | Deep Learning | Significant reduction in design time |
AI in Automotive Design | Automotive | CFD Simulation & Optimization | 15% reduction in aerodynamic drag |
Challenges and Limitations of AI in Design
The integration of artificial intelligence into the design process, while promising significant advancements, is not without its challenges. Successfully leveraging AI in design requires a careful consideration of its limitations and potential pitfalls, ensuring ethical implementation and mitigating risks to achieve optimal results. A balanced approach that combines the strengths of AI with human ingenuity is crucial for maximizing the benefits while minimizing the drawbacks.AI’s role in design is evolving, but its capabilities are still constrained by inherent limitations.
Understanding these limitations is paramount to avoid unrealistic expectations and to develop effective strategies for human-AI collaboration.
Data Bias and Algorithmic Fairness
AI algorithms are trained on vast datasets, and if these datasets reflect existing societal biases, the AI system will inevitably perpetuate and even amplify those biases in its design outputs. For instance, an AI trained primarily on images of conventionally attractive models might generate designs that consistently exclude individuals of diverse body types or ethnic backgrounds. This can lead to designs that are not inclusive or representative of the broader population, potentially reinforcing harmful stereotypes.
Addressing this requires careful curation of training data to ensure diversity and representation, as well as the development of algorithms that are less susceptible to bias. Regular auditing of AI systems for bias is also essential.
Ethical Considerations in AI-Driven Design
The use of AI in design raises several ethical considerations. One key concern is the potential for job displacement. As AI systems become more capable of automating design tasks, there is a risk that human designers may lose their jobs. Another ethical concern relates to intellectual property rights. Determining ownership of designs generated by AI systems is a complex legal and ethical issue.
Furthermore, the potential for AI-generated designs to be used for malicious purposes, such as creating deepfakes or designing harmful products, necessitates careful consideration of the ethical implications. Establishing clear ethical guidelines and regulations is crucial to navigate these challenges responsibly.
The Role of Human Creativity and Intuition
While AI can assist with many aspects of the design process, it cannot fully replace human creativity and intuition. AI excels at generating variations on existing designs, identifying patterns, and optimizing for specific criteria. However, it often lacks the capacity for truly innovative, groundbreaking designs that require a deep understanding of human needs, emotions, and cultural contexts. Human designers bring a unique ability to synthesize diverse inputs, to make subjective judgments, and to imbue designs with emotional resonance – qualities that are currently beyond the capabilities of AI.
The most successful AI-driven design processes will be those that leverage the strengths of both AI and human designers.
Potential Risks and Mitigation Strategies
The integration of AI in design presents several potential risks, but many can be mitigated through careful planning and implementation.
- Risk: Unforeseen biases in AI-generated designs leading to unfair or discriminatory outcomes. Mitigation: Employ diverse and representative datasets for training AI models; regularly audit AI systems for bias; incorporate human oversight to identify and correct biased outputs.
- Risk: Loss of human jobs due to AI automation. Mitigation: Retraining and upskilling programs for designers to adapt to new roles; focusing on AI’s role as a design assistant rather than a replacement.
- Risk: Intellectual property disputes regarding AI-generated designs. Mitigation: Developing clear legal frameworks for ownership of AI-generated intellectual property; establishing transparent processes for attribution and licensing.
- Risk: Malicious use of AI-generated designs. Mitigation: Implementing security measures to prevent unauthorized access and misuse of AI systems; developing ethical guidelines for the use of AI in design.
- Risk: Over-reliance on AI, leading to a decline in human creativity and critical thinking. Mitigation: Emphasizing the complementary nature of AI and human designers; fostering a collaborative environment where AI supports human creativity, not replaces it.
The Future of AI in Design Innovation
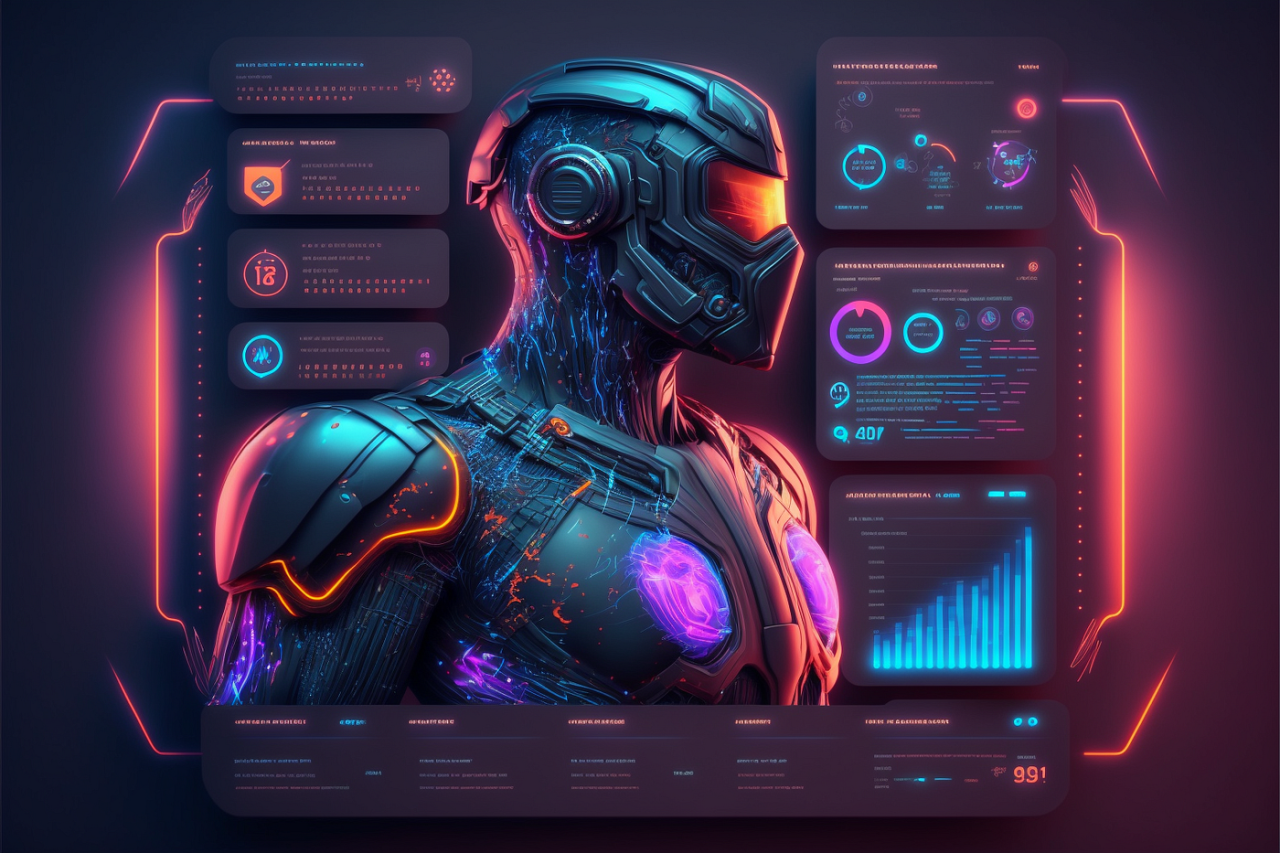
The integration of artificial intelligence into the design process is rapidly evolving, promising a future where design solutions are generated with unprecedented speed, efficiency, and creativity. While current applications focus primarily on automating tasks and assisting designers, the coming years will likely witness a paradigm shift, with AI taking on more significant roles in the creative process itself. This evolution will necessitate a re-evaluation of the designer’s role and a focus on fostering a collaborative human-AI partnership.AI-driven design tools are expected to become increasingly sophisticated, moving beyond simple automation to encompass more complex creative tasks.
This advancement will be fueled by continuous improvements in machine learning algorithms, particularly generative models capable of producing novel and unexpected designs. For example, we can anticipate the development of AI systems that not only generate design options based on specified parameters but also iteratively refine these designs based on user feedback and performance data, leading to a continuous optimization loop.
This will allow designers to explore a much wider range of design possibilities than currently feasible.
Enhanced Generative Design Capabilities
Generative design, already a powerful tool, will be significantly enhanced by advancements in AI. Future systems will likely be capable of understanding and responding to more nuanced design briefs, incorporating contextual information such as cultural trends, material properties, and manufacturing constraints with greater accuracy. Imagine an AI system capable of designing a sustainable building not only based on its intended function and budget but also considering the local climate, available resources, and long-term environmental impact – all while exploring hundreds of unique architectural solutions simultaneously.
This level of sophistication will significantly accelerate the design process and potentially lead to more innovative and sustainable outcomes.
AI-Powered Personalization and Customization
The increasing availability of personalized data will fuel the development of AI systems capable of generating highly customized design solutions tailored to individual needs and preferences. This is already evident in areas such as e-commerce, where AI algorithms personalize product recommendations and even generate customized product designs. In the future, this trend will expand to encompass a much broader range of design disciplines, from interior design and fashion to product design and architecture.
For instance, an AI-powered interior design tool could create personalized room layouts and décor suggestions based on a user’s lifestyle, aesthetic preferences, and even their social media activity.
The Evolving Role of the Designer
The increasing capabilities of AI will not replace designers but rather transform their roles. Designers of the future will act as curators, strategists, and critical thinkers, guiding the AI, refining its outputs, and ensuring the designs align with human values and ethical considerations. Their expertise will be crucial in interpreting complex briefs, defining design goals, and evaluating the ethical and societal implications of AI-generated designs.
The focus will shift from manual execution to strategic direction and human-centered design thinking, ensuring that AI-driven design solutions remain relevant, meaningful, and ultimately beneficial to society. This collaborative approach will leverage the strengths of both human creativity and AI’s computational power, leading to a more efficient and innovative design process.
Ethical Considerations in AI-Generated Designs
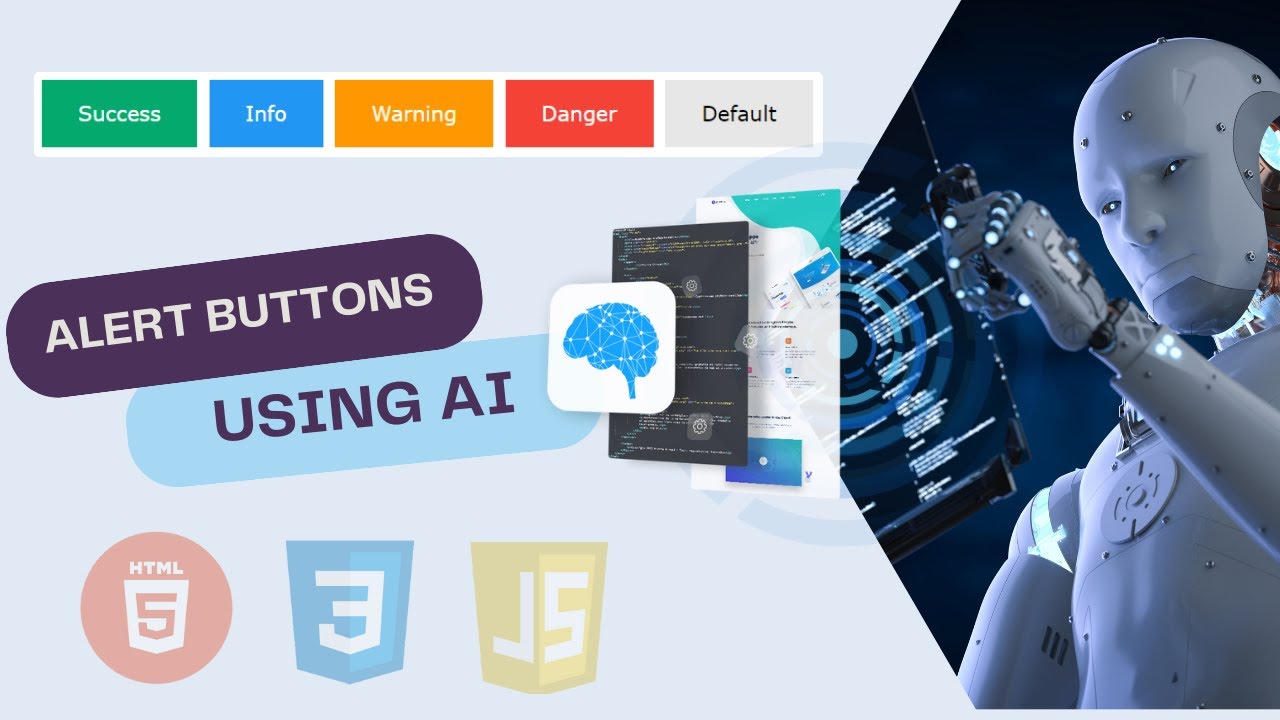
The increasing use of artificial intelligence (AI) in design raises significant ethical concerns that demand careful consideration. The potential for bias, the implications for intellectual property rights, and the lack of clear accountability all necessitate the development of robust ethical guidelines and practices. Failure to address these issues could lead to unfair or discriminatory outcomes, stifle innovation, and erode public trust in AI-driven design solutions.The integration of AI into the design process introduces a complex interplay of technological capabilities and human responsibility.
Understanding the ethical dimensions of this integration is crucial for ensuring that AI serves as a tool for positive change and equitable outcomes, rather than exacerbating existing societal inequalities. This section explores key ethical considerations and proposes strategies for mitigating potential risks.
Intellectual Property Rights in AI-Generated Designs
Determining ownership of designs generated by AI systems presents a significant legal and ethical challenge. Current intellectual property laws are largely based on human creativity and authorship, making it difficult to apply them directly to AI-generated outputs. Questions arise regarding who owns the copyright: the AI developer, the user who prompts the AI, or the AI itself? The lack of clear legal frameworks could hinder innovation by creating uncertainty and discouraging investment in AI design tools.
Existing case law is still evolving, and various jurisdictions are taking different approaches to this novel legal problem. For example, some countries may grant copyright to the user who provided the input that guided the AI’s creative process, while others might consider the AI developer as the owner. This ambiguity underscores the need for a comprehensive legal and ethical framework to address intellectual property rights in the context of AI-generated designs.
Bias and Fairness in AI-Driven Design
AI algorithms are trained on vast datasets, and if these datasets reflect existing societal biases, the resulting designs may perpetuate and even amplify those biases. For example, an AI trained on a dataset predominantly featuring images of a certain demographic might generate designs that cater primarily to that group, excluding or marginalizing others. This can lead to unfair or discriminatory outcomes in areas such as architecture, product design, and urban planning.
Mitigating bias requires careful curation of training datasets, algorithmic transparency, and ongoing monitoring of AI-generated outputs to identify and address potential biases. Furthermore, diverse teams involved in developing and deploying AI design tools can help ensure a more inclusive and equitable outcome. Regular audits of the data and algorithms are essential to ensure fairness and avoid perpetuating harmful stereotypes.
Accountability and Transparency in AI Design Processes
Establishing clear lines of accountability when AI systems make design decisions is crucial. If an AI-generated design causes harm or damage, who is responsible? The AI developer, the user, or the AI itself? Transparency in the AI design process is essential for determining accountability. This includes making the algorithms and training data used by the AI system readily available for scrutiny.
Such transparency allows for independent evaluation and helps to identify potential problems or biases. Furthermore, clear guidelines and protocols should be established to ensure that human oversight is maintained throughout the design process, even when AI is involved. This includes mechanisms for human review and intervention when necessary. The goal is to create a system where responsibility is clearly defined and appropriate measures can be taken to address any negative consequences.
Guidelines for Ethical AI Design Practices
To ensure responsible development and deployment of AI in design, the following guidelines should be adopted:
- Prioritize human well-being and societal benefit above all else.
- Promote fairness, inclusivity, and non-discrimination in all aspects of the design process.
- Ensure transparency and explainability of AI algorithms and decision-making processes.
- Establish clear lines of accountability for AI-generated designs and their outcomes.
- Develop mechanisms for human oversight and intervention in AI-driven design processes.
- Regularly audit AI systems for bias and ensure ongoing monitoring of their performance.
- Foster collaboration between designers, AI developers, ethicists, and policymakers.
- Continuously educate and train designers and other stakeholders on ethical considerations related to AI.
Outcome Summary
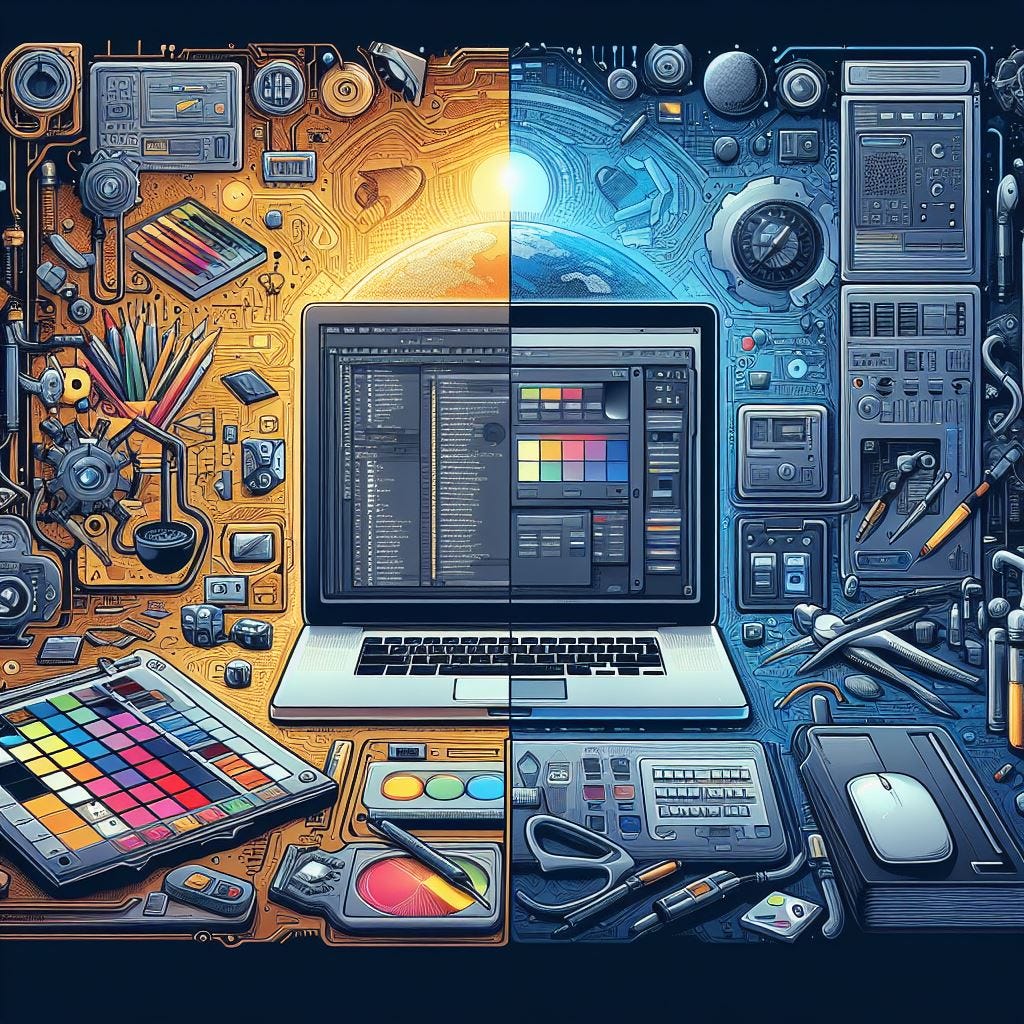
As we’ve seen, the use of AI in generating innovative design solutions presents a paradigm shift in the creative industries. While challenges exist, particularly regarding ethical considerations and the potential displacement of human creativity, the potential benefits are undeniable. AI is not meant to replace designers, but to empower them, providing new tools and capabilities to explore uncharted territories of design innovation.
The future of design lies in a collaborative partnership between human ingenuity and the power of artificial intelligence, leading to a new era of creative possibilities and groundbreaking solutions.