AI-driven tools for remote worker security and data protection are revolutionizing how businesses safeguard their data and employees. The rise of remote work has exponentially increased the attack surface, leaving organizations vulnerable to sophisticated cyber threats. Traditional security measures struggle to keep pace with these evolving threats, but AI offers a powerful solution. By leveraging machine learning and advanced analytics, AI-powered tools can proactively identify and mitigate risks, enhancing security posture without sacrificing productivity.
This comprehensive guide explores the diverse applications of AI in securing remote workforces, covering everything from endpoint protection and data loss prevention to user behavior analytics and threat intelligence. We’ll examine various AI-driven solutions, compare their effectiveness, and discuss the crucial considerations for successful implementation. We’ll also delve into the future of AI in remote worker security, highlighting emerging trends and technologies that promise even greater protection.
Introduction to AI-Driven Security for Remote Workers
The rise of remote work has dramatically expanded the attack surface for organizations. Traditional security perimeters, designed for on-premise networks, are rendered ineffective when employees access company data and systems from diverse, often unsecured, locations. This increased vulnerability exposes sensitive information to a wider range of threats, including phishing attacks, malware infections, and data breaches. The challenge lies in maintaining robust security while accommodating the flexibility and accessibility demanded by a remote workforce.AI-driven security tools offer a significant advancement over traditional methods by leveraging machine learning and advanced analytics to proactively identify and mitigate these threats.
Unlike rule-based systems that rely on pre-defined signatures, AI solutions adapt to evolving threats in real-time, learning from past incidents to anticipate and prevent future attacks. This adaptive nature is crucial in the dynamic landscape of cyber threats, where attackers constantly refine their techniques. Furthermore, AI can automate many security tasks, reducing the workload on IT teams and allowing them to focus on more strategic initiatives.
AI’s Enhanced Threat Detection Capabilities
AI algorithms excel at identifying anomalies in user behavior and network traffic that might indicate malicious activity. For instance, an AI system can detect unusual login attempts from unfamiliar locations or devices, flagging potential unauthorized access. It can also analyze network traffic patterns to identify suspicious communications or data exfiltration attempts that might go unnoticed by traditional intrusion detection systems.
This proactive approach allows for immediate intervention, minimizing the impact of potential breaches. Real-world examples include AI-powered solutions detecting and blocking sophisticated phishing attacks that bypass traditional email filters, as well as identifying and isolating compromised endpoints before they can spread malware across the network.
AI-Powered Data Loss Prevention (DLP)
AI significantly enhances data loss prevention (DLP) capabilities for remote workers. Traditional DLP solutions often rely on filtering and rule-based systems, which are easily circumvented by sophisticated attackers. AI-powered DLP, however, can analyze the content of documents and communications, identifying sensitive information regardless of its format or s. This includes identifying personally identifiable information (PII), financial data, and intellectual property.
AI can also learn the typical data usage patterns of individual employees and flag deviations from the norm, indicating potential data leaks. For example, an AI-powered DLP system might detect an employee attempting to transfer a large amount of sensitive data to an unauthorized external server, prompting an immediate alert and potential intervention.
AI-Driven Access Control and Authentication
AI enhances security by providing more sophisticated access control and authentication mechanisms. Beyond traditional passwords and multi-factor authentication, AI can leverage behavioral biometrics to verify user identity based on typing patterns, mouse movements, and other unique characteristics. This adds an extra layer of security, making it harder for attackers to gain unauthorized access even if they have obtained valid credentials.
Furthermore, AI can dynamically adjust access privileges based on user context and risk level, ensuring that only authorized personnel have access to sensitive information. For example, an AI system might restrict access to certain data based on the employee’s location or the device they are using. This context-aware access control further minimizes the risk of data breaches.
AI-Powered Endpoint Security
Securing remote worker endpoints is paramount in today’s distributed work environment. The traditional perimeter-based security model is insufficient to protect against the ever-evolving threats faced by remote employees. AI-powered endpoint security solutions offer a more proactive and adaptive approach, leveraging machine learning and advanced analytics to identify and neutralize threats before they can cause significant damage. This sophisticated technology significantly enhances the security posture of organizations with remote workforces.AI-Powered Endpoint Security Solutions for Remote WorkersSeveral AI-powered solutions are available to enhance endpoint security for remote workers.
These solutions go beyond traditional antivirus software by incorporating advanced threat detection capabilities, behavioral analysis, and automated response mechanisms. Examples include endpoint detection and response (EDR) solutions, cloud-based security platforms, and next-generation antivirus (NGAV) products that integrate AI-driven threat intelligence. These tools provide a multi-layered defense against malware, ransomware, phishing attacks, and other cyber threats targeting remote endpoints.AI Algorithms in Endpoint Security: A ComparisonVarious AI algorithms power endpoint security solutions, each with strengths and weaknesses.
Machine learning algorithms, such as supervised learning (using labeled data to train models) and unsupervised learning (identifying patterns in unlabeled data), are commonly used for threat detection. Deep learning, a subset of machine learning using artificial neural networks, can analyze complex patterns and identify subtle anomalies indicative of malicious activity. Supervised learning excels at known threat detection, while unsupervised learning is better at identifying zero-day exploits and novel attacks.
The effectiveness of each algorithm depends on the specific application and the quality of the training data. Deep learning, while powerful, often requires significant computational resources and expertise to implement effectively.Hypothetical Scenario: AI-Powered Endpoint Response to MalwareImagine a remote worker receives a phishing email containing a malicious attachment. Upon opening the attachment, an AI-powered endpoint security system immediately flags suspicious activity.
The system’s machine learning algorithms detect unusual file behavior, such as attempts to access sensitive data or communicate with external command-and-control servers. The system automatically quarantines the malicious file, preventing further damage. Simultaneously, the system generates an alert, notifying the security operations center (SOC) of the incident. The SOC analysts can then investigate the incident further, analyze the threat, and take appropriate remediation steps, such as patching vulnerabilities or deploying updated security policies.
The entire process is automated and significantly faster than traditional methods, minimizing the impact of the attack.Comparison of AI-Powered Endpoint Security Solutions
Solution | Key Features | Pricing | Target Audience |
---|---|---|---|
CrowdStrike Falcon | EDR, threat intelligence, vulnerability management, endpoint protection | Subscription-based, varying by features and number of endpoints | Large enterprises, mid-sized businesses |
SentinelOne | EDR, AI-powered threat detection, autonomous response, vulnerability management | Subscription-based, varying by features and number of endpoints | Large enterprises, mid-sized businesses, managed service providers |
Carbon Black Cloud | EDR, threat prevention, incident response, endpoint protection | Subscription-based, varying by features and number of endpoints | Large enterprises, mid-sized businesses |
Sophos Intercept X | NGAV, EDR, exploit prevention, ransomware protection | Subscription-based, varying by features and number of endpoints | Small businesses, mid-sized businesses, large enterprises |
Data Loss Prevention (DLP) with AI
Data Loss Prevention (DLP) is crucial for organizations, especially those with remote workforces, where sensitive data is constantly at risk. AI significantly enhances DLP capabilities by automating the identification and prevention of data breaches, going beyond traditional rule-based systems to offer a more proactive and adaptive security posture. This advanced approach allows for the detection of subtle anomalies and patterns that might otherwise go unnoticed.AI algorithms play a pivotal role in modern DLP systems by analyzing vast amounts of data to identify sensitive information and predict potential data leaks.
This analysis extends beyond simple matching, incorporating contextual understanding and machine learning to adapt to evolving threats and data usage patterns.
AI-Driven DLP Mechanisms
AI-powered DLP solutions employ several techniques to identify and prevent data loss. These include machine learning algorithms that analyze data patterns to detect anomalies, such as unusual access times or file transfers involving sensitive data. Natural language processing (NLP) is used to understand the context of communications, identifying potentially sensitive information within emails, chat messages, and other forms of communication.
Furthermore, these systems leverage behavioral biometrics to detect suspicious user activity, such as unusual login attempts or rapid data deletion. The combination of these techniques provides a comprehensive and layered approach to data protection.
Examples of AI-Driven DLP Tools
Several vendors offer AI-driven DLP tools with varying functionalities. For instance, some solutions focus on email security, using AI to scan emails for sensitive information and block attempts to send such data outside the organization. Others provide endpoint protection, monitoring file activity and preventing unauthorized access or transfer of sensitive files. Advanced solutions offer a combination of these functionalities, integrating with various platforms and providing centralized management.
These tools often incorporate threat intelligence feeds to stay ahead of emerging threats and adapt their detection capabilities accordingly. A specific example could be a hypothetical tool, “SecureFlow AI,” which uses machine learning to identify patterns in user behavior related to sensitive data access, flagging suspicious activity and automatically blocking unauthorized transfers. Another example could be a hypothetical solution like “DataShield AI” which employs NLP to analyze communication channels, identifying and flagging potentially sensitive information embedded within informal communications.
Best Practices for Implementing AI-Driven DLP Strategies
Implementing an effective AI-driven DLP strategy within a remote work environment requires a multi-faceted approach.
The following best practices should be considered:
- Comprehensive Data Discovery and Classification: Begin by identifying and classifying all sensitive data assets within the organization. This involves understanding the types of data, its location, and its sensitivity level.
- AI-Powered Monitoring and Alerting: Implement AI-driven monitoring tools that can detect anomalies and potential data breaches in real-time, providing timely alerts to security teams.
- Regularly Update and Train AI Models: AI models need to be regularly updated with new data and trained to adapt to evolving threats and data usage patterns. This ensures the system remains effective in identifying and preventing data loss.
- Integration with Existing Security Tools: Integrate AI-driven DLP solutions with existing security tools, such as SIEM (Security Information and Event Management) systems, to provide a holistic security posture.
- Employee Training and Awareness: Educate employees about data security best practices and the importance of adhering to company policies. This includes training on how to identify and report potential data breaches.
- Regular Security Audits and Assessments: Conduct regular security audits and assessments to identify vulnerabilities and ensure the effectiveness of DLP strategies. This should include testing the AI-driven systems to validate their accuracy and effectiveness.
AI in User and Entity Behavior Analytics (UEBA) for Remote Access
The rise of remote work has significantly expanded the attack surface for organizations, making robust security measures paramount. AI-powered User and Entity Behavior Analytics (UEBA) offers a powerful solution for monitoring remote worker activity and detecting insider threats, significantly improving overall security posture. By analyzing vast amounts of data and identifying anomalies in user and system behavior, UEBA systems provide proactive threat detection capabilities that are difficult to achieve with traditional security tools.AI enhances UEBA by automating the analysis of massive datasets, identifying subtle patterns indicative of malicious activity that might be missed by human analysts.
This automation frees up security teams to focus on investigating high-priority alerts and responding to threats effectively. Furthermore, AI’s ability to learn and adapt over time ensures that the system continuously improves its accuracy in identifying threats, even as attacker techniques evolve.
Establishing Baselines for Normal User Behavior and Identifying Deviations
AI-powered UEBA systems establish baselines of normal user behavior by analyzing historical data such as login times, access patterns, data accessed, and system interactions. This baseline represents the typical activity of a user or entity. Machine learning algorithms then continuously monitor real-time activity, comparing it to the established baseline. Significant deviations from this baseline, such as unusual login times from unfamiliar locations, unusually high data access volumes, or access to sensitive data outside of normal work hours, trigger alerts, signaling potential malicious activity.
The system’s ability to learn and adapt allows it to adjust baselines as user behavior naturally changes over time, maintaining accuracy and minimizing false positives.
Detecting Suspicious Login Attempts or Data Exfiltration Attempts
Consider a hypothetical scenario: Sarah, a remote employee in the marketing department, typically logs in to the company network between 9 AM and 5 PM from her home IP address. An AI-powered UEBA system would establish this as her normal behavior. One evening, at 11 PM, a login attempt is detected from an unfamiliar IP address in a different country, attempting to access Sarah’s account.
The system immediately flags this as a significant deviation from her established baseline. Further investigation might reveal a phishing attack or credential compromise. Similarly, if Sarah suddenly begins downloading unusually large volumes of data to an external cloud storage service, the UEBA system would detect this as anomalous behavior, potentially indicating data exfiltration. The system would then generate an alert, allowing security personnel to investigate and prevent further data loss.
This proactive detection significantly reduces the window of opportunity for attackers and minimizes potential damage.
Secure Access Service Edge (SASE) and AI
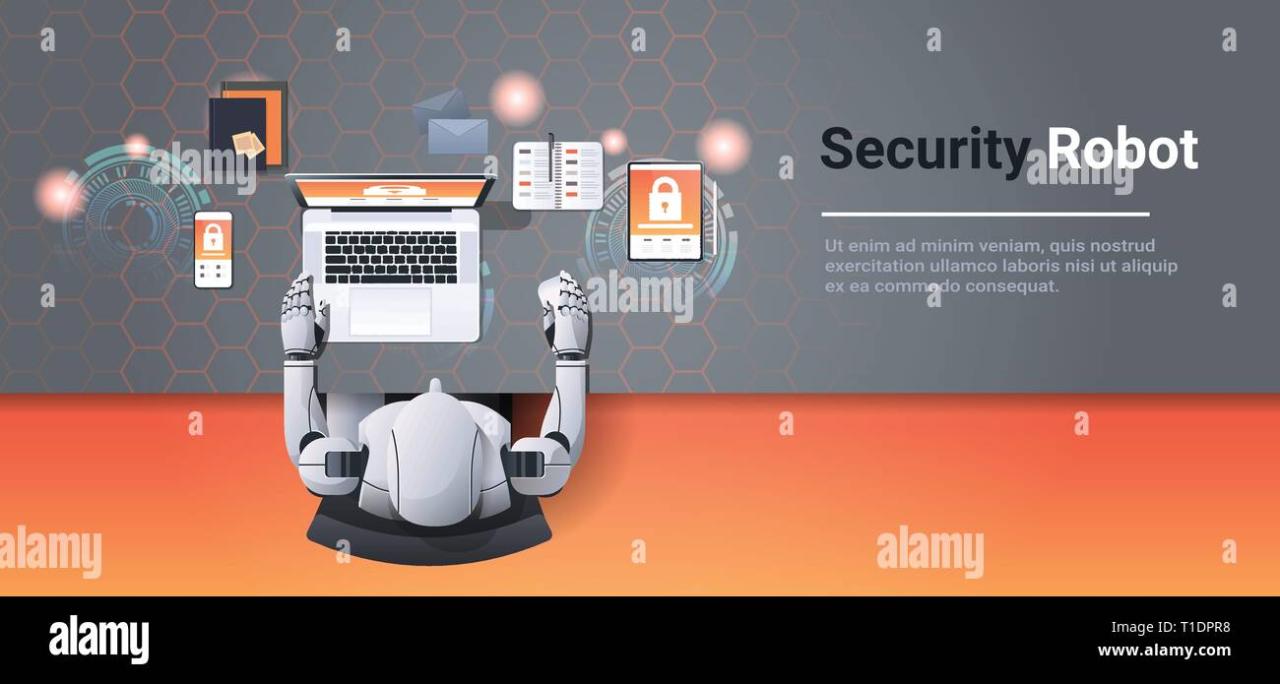
Secure Access Service Edge (SASE) architectures are transforming how organizations secure remote worker access to applications and data. The integration of Artificial Intelligence (AI) significantly enhances SASE’s capabilities, providing a more robust, efficient, and user-friendly security posture. AI’s ability to analyze vast amounts of data in real-time allows for proactive threat detection and response, adaptive security policy enforcement, and a more seamless user experience.AI enhances several key security features within SASE architectures for remote workers.
By analyzing network traffic patterns, user behavior, and device characteristics, AI algorithms can identify and mitigate threats far more effectively than traditional rule-based systems. This proactive approach reduces the risk of successful cyberattacks and data breaches, ensuring consistent security regardless of location or device. Furthermore, AI facilitates the automation of security tasks, reducing the burden on IT administrators and enabling faster responses to emerging threats.
AI Optimization of Network Traffic Routing and Security Policy Enforcement
AI plays a crucial role in optimizing network traffic routing within the SASE framework. By analyzing real-time network conditions and user requirements, AI algorithms can dynamically route traffic to the optimal path, minimizing latency and ensuring optimal application performance. This intelligent routing also contributes to improved security by directing traffic away from compromised or congested network segments. Simultaneously, AI enhances security policy enforcement by adapting policies based on real-time risk assessments.
For instance, if an unusual access pattern is detected from a specific user or device, AI can automatically trigger stricter authentication measures or block access altogether. This dynamic adaptation of security policies ensures that security measures are always relevant and effective, adapting to changing threats and user behavior. Consider a scenario where a remote worker attempts to access sensitive data from a high-risk location.
AI, analyzing the geolocation data and user behavior, could instantly enforce multi-factor authentication or even temporarily restrict access, preventing potential breaches.
AI Improvement of User Experience in a SASE Environment, AI-driven tools for remote worker security and data protection
While maintaining robust security is paramount, AI also contributes to a significantly improved user experience within a SASE environment. AI-powered features such as automated provisioning, intelligent access control, and seamless application delivery enhance user productivity and reduce IT support requests. For example, AI can automatically provision access to specific applications based on a user’s role and location, eliminating the need for manual configuration and reducing wait times.
Similarly, AI-driven anomaly detection systems can proactively identify and address performance issues, ensuring a consistent and reliable user experience. In a practical example, if an application becomes slow or unresponsive for a specific user, AI can analyze the network traffic and identify the bottleneck, automatically rerouting traffic or taking other corrective actions to restore optimal performance without user intervention.
This proactive approach minimizes user frustration and maintains productivity.
AI-Driven Threat Intelligence and Response for Remote Teams
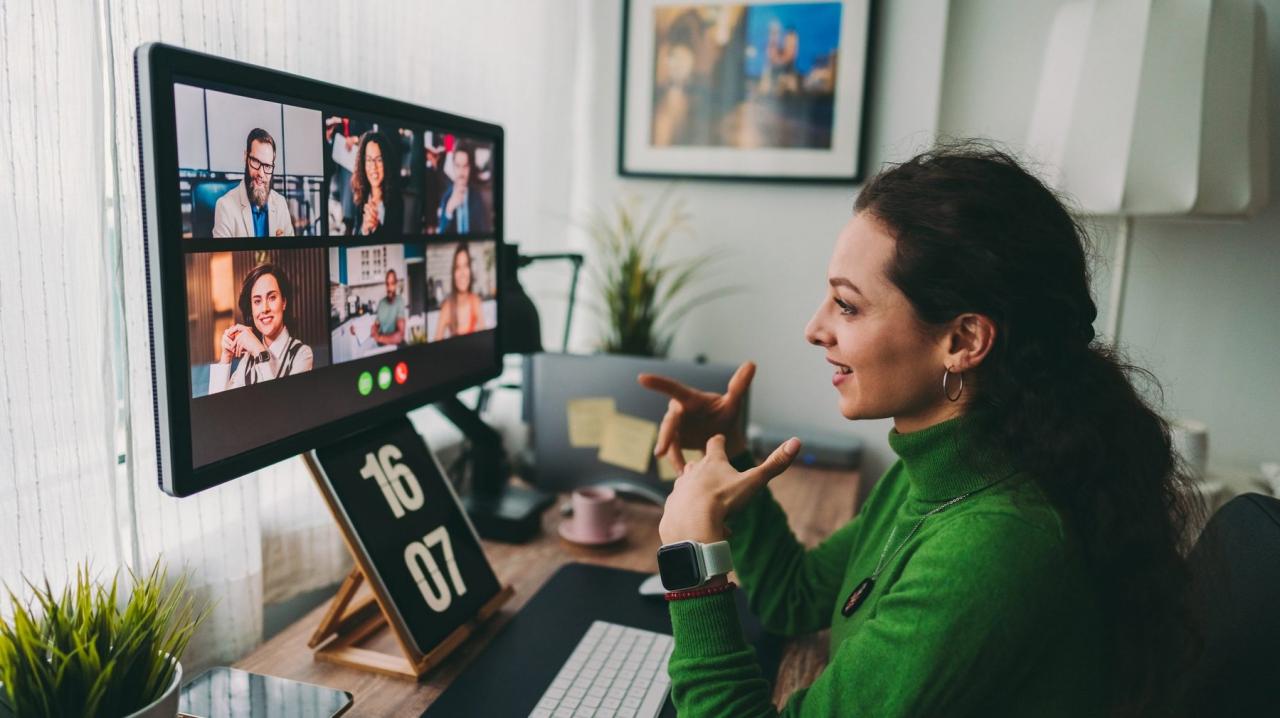
AI significantly enhances security for remote workers by proactively identifying and mitigating threats. By analyzing vast datasets and identifying patterns indicative of malicious activity, AI-driven threat intelligence platforms offer a level of protection impossible to achieve through manual processes alone. This proactive approach minimizes the impact of breaches and reduces the overall security risk profile for organizations with distributed workforces.AI processes threat intelligence by correlating data from various sources, including internal security logs, external threat feeds, and employee activity data.
This multi-faceted approach allows for the identification of sophisticated attacks that might otherwise go undetected. Machine learning algorithms identify anomalies in network traffic, user behavior, and endpoint activity, flagging potentially malicious actions in real-time. This enables rapid response to emerging threats and the proactive blocking of known attack vectors before they can compromise systems. For instance, an AI system might detect unusual login attempts from unfamiliar geographical locations, immediately triggering an alert and potentially blocking access.
AI-Driven Threat Identification and Mitigation
AI systems analyze threat intelligence feeds, identifying known malware signatures, phishing campaigns, and other emerging threats. This information is then used to create profiles of potential risks specific to remote workers. For example, an AI system could identify a surge in phishing attempts targeting a specific industry or using a particular social engineering tactic. This allows security teams to proactively warn remote employees about the specific threats they are most likely to encounter.
The system can also automatically apply protective measures, such as blocking access to known malicious websites or filtering suspicious emails. This proactive approach significantly reduces the likelihood of successful attacks.
AI-Automated Incident Response
AI automates many aspects of incident response, significantly reducing the time it takes to contain and remediate security breaches. When a threat is detected, AI can automatically isolate affected systems, block malicious actors, and initiate a rollback of compromised data. This automated response minimizes the impact of the breach and prevents further damage. For example, if an AI system detects a ransomware attack on a remote employee’s computer, it can automatically quarantine the infected machine from the network, preventing the malware from spreading to other systems.
Simultaneously, the system can initiate a recovery process from a known good backup. This automated response significantly reduces the time and resources required to recover from a security incident.
Preventing Phishing Attacks with AI-Driven Threat Intelligence
A step-by-step procedure demonstrating how AI-driven threat intelligence can prevent a phishing attack targeting remote workers:
- Threat Intelligence Gathering: The AI system continuously monitors threat intelligence feeds for emerging phishing campaigns, identifying common tactics, URLs, and email subject lines.
- Pattern Recognition: Machine learning algorithms analyze incoming emails and websites, identifying patterns consistent with known phishing attacks. This includes analyzing email headers, links, and content for suspicious characteristics.
- Real-time Analysis: As emails arrive, the AI system analyzes them in real-time, comparing them against the known phishing patterns and threat intelligence database.
- Automated Filtering: Suspicious emails are automatically filtered and quarantined before reaching the user’s inbox. The AI system may also block access to malicious websites identified in the phishing emails.
- User Notification: Users are notified about the blocked emails and provided with information on why they were flagged as suspicious. This educates users and reinforces security awareness.
- Continuous Learning: The AI system continuously learns from new phishing attacks and updates its threat intelligence database, improving its accuracy and effectiveness over time.
Challenges and Considerations of AI in Remote Worker Security: AI-driven Tools For Remote Worker Security And Data Protection
Implementing AI-driven security solutions for remote workers offers significant advantages, but it also presents unique challenges and ethical considerations that require careful attention. A balanced approach, acknowledging both the potential and limitations, is crucial for effective and responsible deployment. Ignoring these challenges can lead to vulnerabilities and unintended consequences.
AI Limitations in Remote Worker Security
AI-powered security systems, while powerful, are not infallible. Their effectiveness depends heavily on the quality and quantity of data used for training. Insufficient or biased training data can lead to inaccurate threat detection, resulting in false positives or, more critically, missed threats. Furthermore, sophisticated attackers are constantly evolving their techniques, potentially outpacing the ability of AI systems to adapt and respond effectively.
This arms race necessitates continuous updates and retraining of AI models to maintain their efficacy. Another limitation stems from the reliance on network connectivity. If a remote worker operates in an area with poor or intermittent internet access, the effectiveness of AI-based monitoring and protection is significantly reduced. Finally, the complexity of AI algorithms can make it difficult to understand their decision-making processes, hindering troubleshooting and incident response.
Ethical Implications of AI-Powered Surveillance
The use of AI-powered surveillance tools for remote employees raises significant ethical concerns. The potential for misuse and abuse is substantial. Constant monitoring of employee activity, even if anonymized, can create a climate of distrust and anxiety, impacting morale and productivity. Concerns regarding data privacy and potential biases embedded within AI algorithms are paramount. For example, an AI system trained on data reflecting existing societal biases could disproportionately flag certain employee behaviors or demographics as suspicious, leading to unfair or discriminatory outcomes.
Transparency and accountability in the deployment and use of such systems are crucial to mitigate these risks. Clear guidelines and policies outlining the permissible scope of surveillance, data handling procedures, and mechanisms for redress are essential.
Mitigating Risks of AI-Driven Security Solutions
Effective risk mitigation requires a multi-faceted approach. Firstly, rigorous testing and validation of AI security solutions are essential before deployment. This includes evaluating their accuracy, robustness, and potential for bias. Secondly, a robust data governance framework is crucial, ensuring data used for training and operation is accurate, complete, and ethically sourced. Regular audits and independent reviews of AI systems can help identify and address potential biases or vulnerabilities.
Thirdly, transparency and employee awareness are key. Employees should be informed about the types of AI-driven security measures in place and how their data is being used. This fosters trust and allows for open communication about potential concerns. Finally, human oversight remains crucial. AI should be viewed as a tool to augment, not replace, human expertise in security management.
Maintaining a balance between automated security and human intervention ensures a robust and ethical security posture.
Future Trends in AI-Driven Remote Worker Security
The landscape of remote work security is constantly evolving, driven by advancements in artificial intelligence and the ever-increasing sophistication of cyber threats. Predicting the future with certainty is impossible, but by analyzing current trends and emerging technologies, we can anticipate significant developments in AI-driven tools for securing remote workforces in the coming years. This will involve a shift towards more proactive, predictive, and automated security measures, reducing reliance on reactive responses to breaches.AI-driven security solutions are already proving their worth in identifying and mitigating threats in real-time.
However, the future will see an even more profound integration of AI, leading to more sophisticated and nuanced security capabilities. This will encompass improved threat detection, automated incident response, and a more personalized security posture tailored to individual remote workers and their specific risk profiles.
Enhanced Threat Detection and Prevention
AI algorithms will become increasingly adept at identifying subtle anomalies indicative of malicious activity. This includes advanced techniques like behavioral biometrics, which analyze user interaction patterns to detect deviations from established baselines, even mimicking the complexity of human decision-making processes. Furthermore, expect to see AI systems that can proactively predict potential threats based on real-time threat intelligence and historical data, allowing for preemptive mitigation strategies.
For instance, an AI system might identify a newly emerging malware variant based on its code signature and automatically update endpoint protection software across all remote devices before any damage occurs. This proactive approach will significantly reduce the window of vulnerability.
Autonomous Incident Response
The future will see a significant increase in the automation of incident response. AI-powered systems will be capable of automatically isolating compromised devices, containing malware outbreaks, and initiating remediation actions without human intervention. This automated response will significantly reduce the time it takes to contain a security incident, minimizing potential damage and disruption. Imagine a scenario where an AI system detects a phishing attack targeting a remote worker.
The system automatically blocks the malicious email, quarantines the affected device, and initiates a password reset, all within seconds, without any need for human intervention. This level of automation will be critical in managing the complexities of securing a distributed workforce.
AI-Driven Zero Trust Security
The concept of Zero Trust will be significantly enhanced by AI. AI will play a crucial role in verifying user identities and device trustworthiness continuously, adapting security policies based on real-time risk assessments. This dynamic approach will ensure that access is granted only to authorized users and devices, regardless of their location or network. For example, AI could analyze a user’s device posture, location, and behavior to determine the appropriate level of access to sensitive data, adapting access controls dynamically based on the risk profile.
This granular control will be critical in mitigating the risks associated with remote access to corporate resources.
Predictive Security Posture Management
AI will allow for a more proactive and predictive approach to security posture management. Instead of simply reacting to security incidents, organizations will be able to anticipate potential vulnerabilities and proactively address them. This predictive capability will be crucial in maintaining a strong security posture in the face of constantly evolving threats. For example, an AI system could analyze the security configurations of all remote devices and identify potential weaknesses before they are exploited by attackers.
This would allow organizations to proactively patch vulnerabilities and implement appropriate security controls, reducing their overall attack surface.
Visual Representation of the AI-Driven Remote Worker Security Landscape in 5 Years
Imagine a dynamic, interconnected network. At the center is a powerful AI security brain, constantly analyzing data from various sources – endpoint devices, network traffic, user behavior, and threat intelligence feeds. This AI brain acts as a central nervous system, dynamically adapting security policies based on real-time risk assessments. Surrounding this central AI are numerous remote worker endpoints, each represented by a node with varying levels of security strength, indicated by color-coding (green for strong, yellow for moderate, red for weak).
Lines connecting the nodes to the central AI represent the constant flow of data and security updates. The network is constantly shifting and adapting, with the AI proactively identifying and mitigating threats, automatically adjusting security controls based on individual user risk profiles and real-time threat intelligence. This illustrates a proactive, self-healing security system, adapting to the dynamic nature of remote work.
Final Thoughts
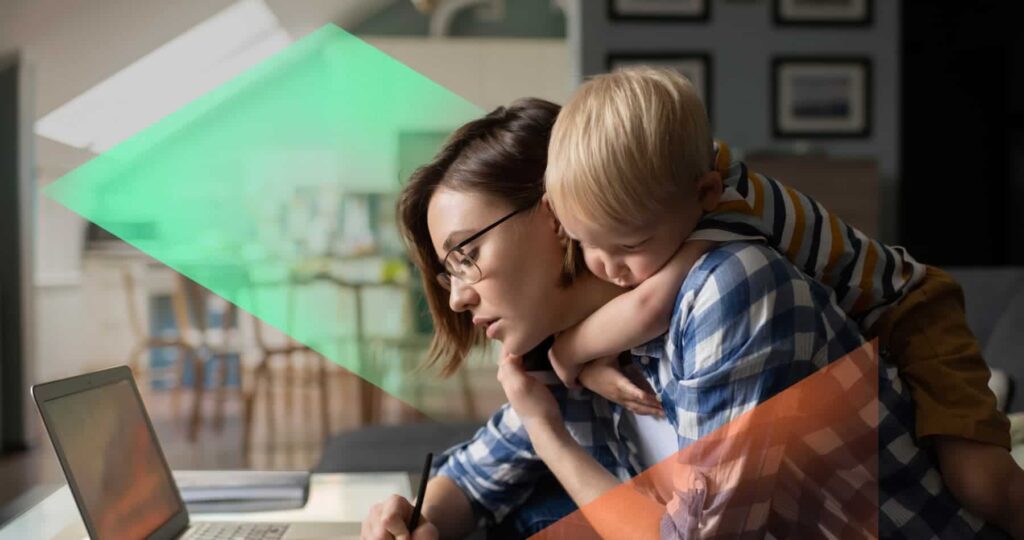
Securing remote workers in today’s digital landscape requires a proactive, intelligent approach. AI-driven tools are no longer a luxury but a necessity for organizations committed to robust data protection and employee safety. By adopting these advanced solutions and understanding their limitations, businesses can significantly reduce their risk profile and maintain a competitive edge in a constantly evolving threat environment.
The future of remote work security lies in the continuous evolution of AI, promising more sophisticated, adaptable, and user-friendly security measures.