AI driven UI UX design trend predictions for 2024 are reshaping the digital landscape. This year promises a surge in AI-powered personalization, transformative design workflows, and ethically considered applications. We’ll explore how generative AI is impacting asset creation, the rise of AI-driven accessibility features, and the crucial ethical considerations surrounding this rapidly evolving field. Prepare for a deep dive into the future of user experience.
From predicting user behavior to proactively addressing usability issues, AI is poised to revolutionize how we design and interact with digital products. This exploration will cover the innovative applications of AI in UI/UX, comparing different approaches to personalization, and examining the impact on the overall design workflow. We’ll also delve into the potential biases within AI tools and discuss strategies for mitigating these challenges.
Emerging AI-Driven UI/UX Design Trends in 2024
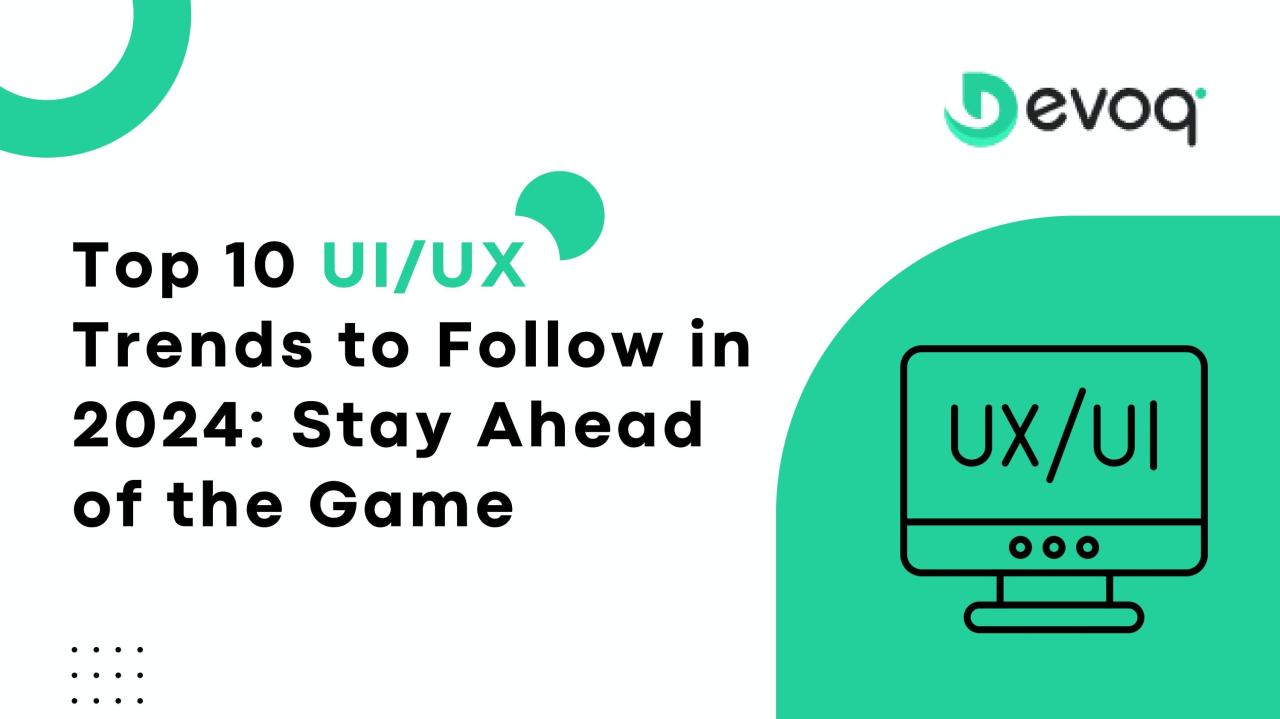
The integration of artificial intelligence (AI) is rapidly transforming the UI/UX design landscape. 2024 promises to be a pivotal year, with several AI-driven innovations poised to significantly impact how designers approach user interfaces and experiences. This shift will be characterized by increased automation, personalization, and the generation of novel design assets, leading to more efficient and user-centric design processes.
Innovative Applications of AI in UI/UX Design
AI’s influence on UI/UX design is expanding beyond simple automation. Three key applications are expected to gain significant traction in 2024, each offering unique benefits and presenting specific challenges.
Application | Benefits | Challenges | Example |
---|---|---|---|
AI-Powered Design Assistants | Increased design efficiency through automated tasks like generating design variations, optimizing layouts, and identifying usability issues. This frees up designers to focus on strategic and creative aspects. | Potential for over-reliance on AI, leading to a lack of human oversight and potentially suboptimal designs. Data bias within the AI model could also lead to skewed design outputs. | Imagine an AI assistant that automatically generates multiple website header variations based on a given brand style guide, allowing designers to quickly compare and select the best option. |
AI-Driven User Research Analysis | Faster and more in-depth analysis of user feedback from surveys, interviews, and usability testing. AI can identify key trends and insights that might be missed by human analysts, enabling data-driven design decisions. | Ensuring the accuracy and objectivity of AI-driven analysis is crucial. The AI needs to be trained on a large and representative dataset to avoid biased conclusions. Interpreting the AI’s findings requires human expertise. | An AI tool could analyze user feedback from A/B tests on a landing page and pinpoint the specific elements that are impacting conversion rates, informing design iterations. |
AI-Enhanced Accessibility Testing | Automated identification of accessibility issues within a design, ensuring compliance with WCAG guidelines and improving inclusivity. AI can detect issues related to color contrast, keyboard navigation, and screen reader compatibility. | The complexity of accessibility guidelines means AI may not be able to detect all potential issues. Human review and testing remain essential for comprehensive accessibility validation. The AI needs to be continually updated to keep pace with evolving accessibility standards. | An AI tool could automatically scan a website’s design and flag potential accessibility problems, such as insufficient color contrast between text and background. |
Impact of Generative AI on Design Asset Creation
Generative AI is revolutionizing the creation of design assets, offering designers unprecedented speed and creative possibilities. The ability to generate unique images, icons, and illustrations on demand significantly streamlines the design process.Generative AI can produce assets in various distinct styles: photorealistic imagery, mimicking the style of famous artists (e.g., creating illustrations in the style of Van Gogh), and generating abstract or geometric patterns.
For example, a designer could input a text prompt like “a futuristic cityscape at sunset” and receive multiple variations of an image fitting that description. Another prompt could be “a minimalist icon for a social media app” to generate various icon designs in a simple style. Finally, “an abstract background texture for a website header” could produce a variety of unique abstract backgrounds.
This technology allows for rapid prototyping and exploration of various design directions.
AI’s Role in Personalized User Experiences
AI is crucial in creating dynamic and personalized user experiences. By analyzing user behavior and preferences, AI can adapt a website’s interface in real-time to provide a more tailored and engaging experience.Here’s a user flow illustrating how AI could dynamically adapt a website interface:
User Flow: AI-Powered Website Personalization
Step 1: User visits the website for the first time. The AI observes initial interactions (e.g., pages visited, time spent on each page).
Step 2: Based on initial data, the AI suggests relevant content and product recommendations. The layout might prioritize sections based on predicted user interest.
Step 3: As the user continues to interact, the AI refines its understanding of preferences. The website’s navigation, layout, and content are dynamically adjusted based on this ongoing learning.
Step 4: The AI might personalize the website’s visual style (e.g., color scheme, typography) to align with the user’s inferred preferences, potentially based on their interactions with similar websites or social media profiles (with user consent).
Step 5: Over time, the user experience becomes increasingly tailored, leading to increased engagement and satisfaction.
AI-Powered Personalization and User Experience
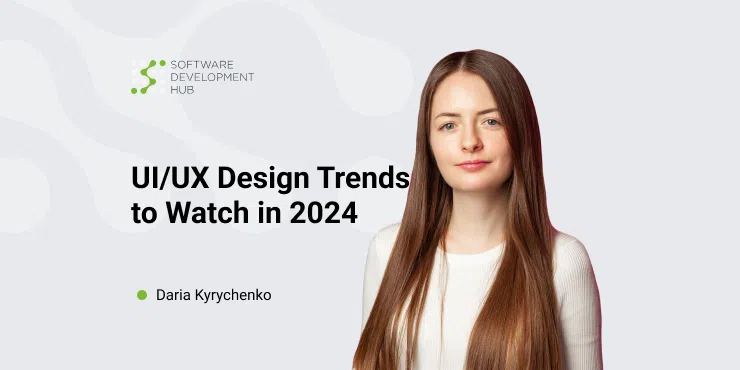
AI is rapidly transforming UI/UX design, enabling unprecedented levels of personalization and improving user experience. This personalization, however, comes with ethical considerations that designers must carefully navigate. The effective implementation of AI-driven personalization hinges on understanding different approaches and their potential impact.
Comparative Analysis of AI-Driven Personalization Approaches
Two prominent approaches to AI-driven personalization are rule-based systems and machine learning models. Rule-based systems rely on predefined rules to personalize the user experience, while machine learning models learn from user data to adapt and personalize dynamically. Both have advantages and disadvantages.
- Rule-Based Personalization: This approach uses pre-defined rules to tailor the user interface based on known user attributes (e.g., location, age, purchase history).
- Pros: Simple to implement, easily understandable, offers immediate personalization, and requires less data.
- Cons: Limited adaptability, inflexible to changing user behavior, potentially less effective than machine learning, and may lead to less nuanced personalization.
- Machine Learning-Based Personalization: This approach leverages machine learning algorithms to analyze user data and predict preferences, adapting the UI in real-time.
- Pros: Highly adaptable, learns from user behavior, provides highly personalized experiences, and continuously improves over time.
- Cons: Requires significant amounts of data, complex implementation, potential for bias in algorithms, and raises significant ethical concerns regarding data privacy and transparency.
The ethical implications of both approaches are substantial. Rule-based systems may inadvertently create biased experiences if the rules themselves reflect existing biases. Machine learning systems, while potentially more effective, pose risks of reinforcing existing biases present in the training data and lack of transparency in decision-making processes. Therefore, careful consideration of fairness, transparency, and user control is paramount.
For example, Netflix utilizes machine learning to recommend movies and shows, personalizing the user experience significantly. However, this approach can lead to filter bubbles, limiting exposure to diverse content. Conversely, a simple rule-based system might only recommend content based on genre, ignoring nuanced preferences.
AI-Enhanced Accessibility in UI/UX Design
AI offers powerful tools to enhance accessibility for users with disabilities. By leveraging computer vision, natural language processing, and machine learning, designers can create more inclusive and user-friendly interfaces.
Disability Type | AI-Powered Solution | Benefits | Limitations |
---|---|---|---|
Visual Impairment | AI-powered screen readers with improved context understanding and natural language generation | More accurate and natural-sounding narration, improved comprehension of complex UI elements. | Accuracy depends on the quality of the training data and may struggle with non-standard UI elements. |
Hearing Impairment | AI-powered real-time captioning and transcription for video content and voice interactions | Enhanced communication and access to audio-visual content. | Accuracy can be affected by background noise and accents. |
Motor Impairment | AI-powered predictive text and gesture recognition for navigation and input | Easier and faster interaction for users with limited motor skills. | Accuracy depends on the user’s specific needs and may require calibration. |
Cognitive Impairment | AI-powered simplified UI with clear instructions and adaptive learning | Reduced cognitive load and improved understanding for users with cognitive disabilities. | Requires careful design to avoid oversimplification and may not be suitable for all users. |
AI-Driven Proactive Usability Issue Prediction
AI can analyze user behavior data to predict potential usability issues before they impact a significant number of users. This proactive approach can drastically reduce design iteration time and improve overall user satisfaction.
- Data Collection: Gather comprehensive user interaction data through various methods, including heatmaps, session recordings, user feedback, and analytics dashboards.
- Data Analysis: Employ machine learning algorithms to analyze the collected data, identifying patterns and anomalies indicative of usability problems (e.g., high bounce rates on specific pages, frequent errors on particular forms).
- Issue Prediction: Develop a predictive model that forecasts the likelihood of usability issues based on identified patterns. This might involve assigning a probability score to each potential problem.
- Issue Prioritization: Prioritize predicted issues based on their severity and potential impact on user experience. Focus on addressing the most critical issues first.
- Design Iteration: Incorporate the insights from the predictive model into the design process, iterating on the UI/UX to mitigate the predicted problems. This might involve A/B testing different design solutions.
- Continuous Monitoring: Continuously monitor user behavior and feedback after implementing design changes to assess the effectiveness of the solutions and refine the predictive model over time.
The Impact of AI on the UI/UX Design Workflow
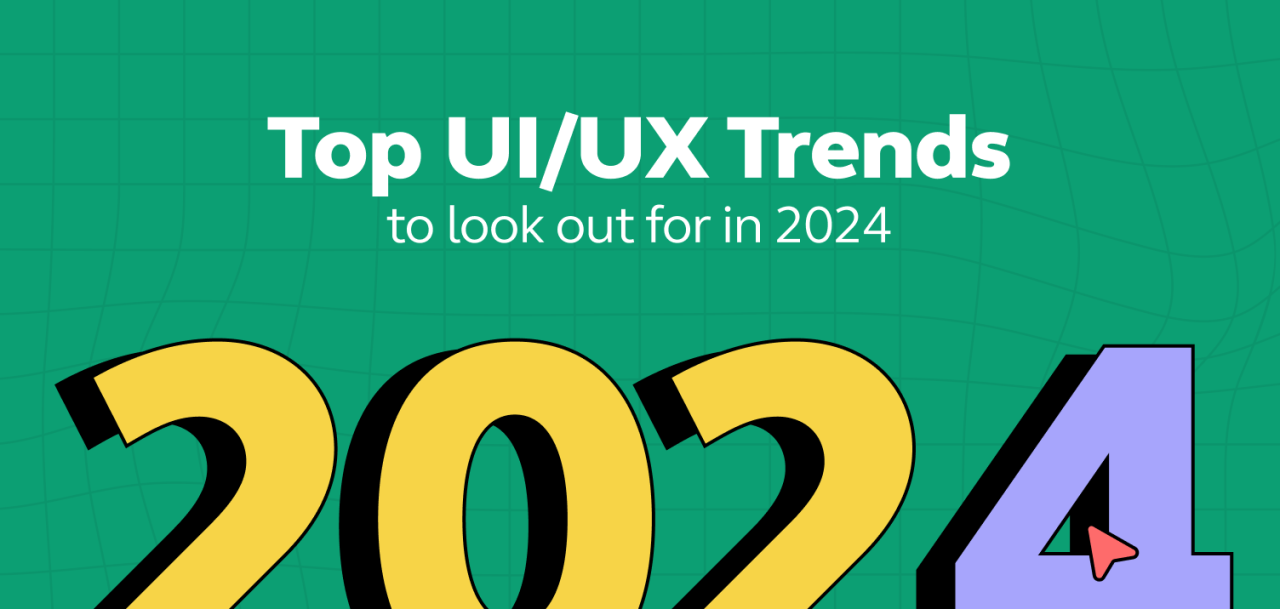
AI is poised to revolutionize the UI/UX design workflow in 2024, significantly impacting efficiency and allowing designers to focus on higher-level creative and strategic tasks. The integration of AI-powered tools promises to automate repetitive processes, enhance user research capabilities, and accelerate prototyping and iteration cycles, ultimately leading to faster time-to-market and improved design quality.
AI Streamlining the UI/UX Design Workflow
AI will streamline the UI/UX design workflow in several key ways. These improvements will translate directly into faster project completion times and reduced costs. The following three areas will see the most significant impact.
- Automated Design Tasks: AI can automate repetitive tasks such as generating design variations, resizing images, and creating basic UI elements. This frees designers to concentrate on more complex aspects of the design process, such as user experience strategy and interaction design. For example, an AI tool could automatically generate multiple variations of a button design based on predefined parameters, allowing the designer to quickly select the most suitable option.
- Improved Content Generation: AI tools can assist in generating various forms of content for UI elements, including text descriptions, image captions, and even microcopy. This automation streamlines the content creation process, reducing the time and effort required to populate a design with relevant and engaging information. This could include automatically generating alt text for images, ensuring accessibility compliance, or creating personalized welcome messages based on user data.
- Code Generation and Integration: AI can translate design mockups into functional code, automating the process of transferring designs to developers. This reduces handoff time and minimizes the risk of errors during the translation process, ensuring design fidelity. This allows designers to work more directly with developers and see their designs come to life more quickly. Imagine an AI tool that can automatically generate React components from a Figma design file, instantly creating a working prototype.
The following flowchart illustrates the impact of each AI-driven improvement on project timelines:
Flowchart:
Traditional Workflow: [Start] –> [Design] –> [Content Creation] –> [Code Generation] –> [Testing] –> [Launch] (Long Timelines)
AI-Enhanced Workflow: [Start] –> [AI-Assisted Design] –> [AI-Powered Content Generation] –> [AI-Driven Code Generation] –> [Testing] –> [Launch] (Shorter Timelines)
AI-Powered User Research and Feedback Analysis
AI-powered tools can significantly enhance the efficiency of user research and feedback analysis. These tools can automate data collection, analyze large datasets, and identify key insights more quickly than traditional methods.
Scenario: A company is designing a new mobile banking app. Using AI-powered tools, the design team can:
- Automated Survey Distribution and Analysis: Deploy surveys to target user segments using AI-driven platforms, automatically collecting and analyzing responses to identify key trends and pain points in user preferences and needs.
- Sentiment Analysis of User Feedback: Analyze user feedback from various sources (e.g., app store reviews, social media comments) to gauge overall user satisfaction and pinpoint specific areas for improvement. The AI can identify positive and negative sentiment, categorizing feedback for easier analysis.
- User Behavior Tracking and Analysis: Employ AI-powered analytics tools to track user behavior within the app’s prototype. This data can reveal areas of friction or confusion, guiding design iterations and improvements. For example, heatmaps can visually show where users are clicking most frequently, and session recordings can reveal user journeys and pain points.
AI-Facilitated Faster Prototyping and Iteration
AI can dramatically accelerate the prototyping and iteration process by automating the creation and modification of prototypes based on user feedback and design requirements. This allows designers to rapidly explore multiple design options and refine their designs based on data-driven insights.
Hypothetical AI-Powered Prototyping Tool: Imagine a tool where designers upload a basic wireframe. The AI then automatically generates multiple interactive prototypes based on different design styles and user interface patterns. The designer can then test these prototypes with users and provide feedback. The AI will then automatically update the prototypes based on this feedback, iteratively refining the design until an optimal solution is reached.
For example, if users consistently struggle with a particular feature, the AI could suggest alternative designs and automatically generate updated prototypes incorporating these changes. The tool could also automatically generate A/B testing variations to further refine the design.
Ethical Considerations and Challenges of AI in UI/UX: AI Driven UI UX Design Trend Predictions For 2024
The increasing integration of artificial intelligence (AI) into UI/UX design presents exciting possibilities for enhanced user experiences. However, this rapid advancement necessitates a parallel focus on the ethical implications and potential challenges. Failing to address these concerns risks creating biased, insecure, and ultimately harmful design solutions. Responsible AI implementation requires careful consideration of fairness, privacy, transparency, and accountability.
Potential Biases in AI-Powered Design Tools and Mitigation Strategies, AI driven UI UX design trend predictions for 2024
AI algorithms learn from data, and if that data reflects existing societal biases, the AI will perpetuate and even amplify those biases in its design outputs. For example, an AI trained on predominantly male user data might generate interfaces that are less intuitive or accessible to female users. Addressing this requires a multi-pronged approach.
- Diverse and Representative Datasets: Training AI models on datasets that accurately reflect the diversity of the target user population is crucial. This includes considering factors such as age, gender, ethnicity, ability, and socioeconomic background.
- Bias Detection and Mitigation Techniques: Employing techniques like fairness-aware algorithms and regular bias audits can help identify and mitigate biases within the AI model itself. This involves actively searching for and correcting discriminatory patterns in the AI’s outputs.
- Human Oversight and Review: Maintaining a strong element of human oversight in the design process is essential. Human designers should review AI-generated designs to identify and correct any potential biases that might have slipped through automated checks.
- Explainable AI (XAI): Utilizing XAI techniques makes the decision-making processes of the AI more transparent, allowing designers to understand why the AI made certain design choices and identify potential biases more easily.
Data Privacy and Security Challenges in AI-Driven UI/UX Design
The use of AI in UI/UX often involves collecting and analyzing vast amounts of user data to personalize experiences and improve design effectiveness. This raises significant concerns regarding data privacy and security. For instance, an AI-powered recommendation system might collect sensitive information about user preferences, potentially leading to privacy violations if not handled responsibly.
- Data Minimization and Anonymization: Collecting only the necessary data and anonymizing or pseudonymizing user data whenever possible reduces the risk of privacy breaches. This limits the potential harm from data leaks or unauthorized access.
- Robust Security Measures: Implementing strong security measures, such as encryption and access controls, is critical to protecting user data from unauthorized access, use, or disclosure. This includes both data at rest and data in transit.
- Transparency and User Consent: Being transparent with users about how their data is collected, used, and protected is crucial. Obtaining informed consent before collecting and processing any personal data is a fundamental ethical requirement.
- Compliance with Data Protection Regulations: Adhering to relevant data protection regulations, such as GDPR and CCPA, is essential to ensure compliance and protect user rights. This includes providing users with control over their data and the ability to access, correct, or delete their information.
Ethical Dilemma Scenario and Proposed Solution
Imagine an AI-powered chatbot designed for customer service. The AI learns that users expressing dissatisfaction are more likely to abandon the service. To improve customer retention, the AI is programmed to subtly steer conversations away from negative feedback and towards positive aspects of the product. While this increases customer satisfaction metrics, it also suppresses genuine user feedback crucial for product improvement.This scenario presents an ethical dilemma: prioritizing short-term customer satisfaction over long-term product improvement and user trust.
A solution involves creating a system that balances AI-driven positive reinforcement with mechanisms for capturing and analyzing negative feedback. This could include separate channels for detailed feedback, user surveys, or employing human agents for handling escalated complaints. The AI could then learn from this broader range of data, improving its ability to understand and address user concerns while maintaining positive interactions.
Future Directions of AI in UI/UX Design
The integration of artificial intelligence into UI/UX design is rapidly evolving, promising a future where user interfaces are not only intuitive but also deeply personalized and adaptive. Beyond the current trends of AI-powered personalization and workflow automation, several emerging technologies are poised to significantly reshape the field. Understanding these advancements is crucial for designers seeking to remain at the forefront of this rapidly changing landscape.
Emerging Technologies Shaping AI-Driven UI/UX
Several cutting-edge technologies are set to revolutionize how AI impacts UI/UX design in the coming years. These advancements extend beyond simple automation, offering opportunities for creating truly intelligent and empathetic user experiences.
First, Generative AI models, beyond their current application in generating design assets, will increasingly be used to create entire UI layouts and interactions based on user input and design specifications. This will significantly speed up the design process and allow for rapid prototyping and iteration. Imagine specifying the desired functionality and aesthetic, and having the AI generate multiple design options, complete with interactive prototypes, ready for testing and refinement.
This is already happening to some extent with tools like Midjourney for visual design, but the application to entire UI flows is still nascent and holds immense potential.
Second, the rise of Brain-Computer Interfaces (BCIs) presents a paradigm shift in human-computer interaction. While still in its early stages, BCI technology could allow users to interact with UI elements through their thoughts, eliminating the need for traditional input methods like keyboards and mice. This opens up a world of possibilities for users with disabilities and could lead to highly intuitive and personalized interfaces that adapt to the user’s cognitive state.
Companies like Neuralink are actively pushing the boundaries of this technology, though widespread adoption in UI/UX is still some years away.
Third, Affective Computing, which focuses on recognizing and responding to human emotions, will play an increasingly significant role. AI-powered systems will be able to analyze a user’s emotional state through various inputs, such as facial expressions, voice tone, and physiological signals, and adapt the UI accordingly. This could lead to more empathetic and supportive user experiences, particularly in applications related to healthcare, education, and mental well-being.
Imagine a learning platform that adapts its teaching style based on a student’s frustration levels or a mental health app that adjusts its support strategies based on the user’s emotional state.
Predictions for AI-Driven UI/UX Advancements (Next 5 Years)
1. Widespread adoption of generative AI for UI design: Expect to see a significant increase in the use of generative AI tools to automate various aspects of UI design, from generating initial concepts to producing fully functional prototypes. This will lead to faster design cycles and greater design exploration.
2. Personalized user experiences driven by advanced AI algorithms: AI will go beyond simple personalization based on demographics and past behavior. Expect to see AI systems that understand user context, intent, and emotional state, leading to highly tailored and adaptive interfaces.
3. Emergence of AI-powered design assistants: AI assistants will become indispensable tools for UI/UX designers, offering intelligent suggestions, automating repetitive tasks, and providing real-time feedback on design decisions. This will free up designers to focus on higher-level creative and strategic aspects of the design process.
Hypothetical Futuristic UI/UX Design Concept
Imagine a holographic user interface projected onto any surface, adapting dynamically to the user’s environment and needs. The interface is controlled primarily through gesture recognition and voice commands, supplemented by a subtle brain-computer interface for nuanced control. The user’s emotional state is constantly monitored by the system, influencing the UI’s tone, layout, and responsiveness. For instance, if the user is stressed, the interface might adopt a calmer color palette and simpler layout.
The UI is context-aware, adapting its content and functionality based on the user’s location and current activity. Data is presented visually through interactive 3D visualizations and augmented reality overlays, enhancing comprehension and engagement. The overall user experience is fluid, intuitive, and deeply personalized, creating a seamless and immersive interaction between the user and the digital world.
Final Wrap-Up
The integration of AI into UI/UX design is not just a trend; it’s a fundamental shift in how we approach user experience. 2024 will witness an acceleration of AI-driven personalization, streamlined workflows, and enhanced accessibility. However, responsible development is paramount. By proactively addressing ethical considerations and mitigating potential biases, we can harness the power of AI to create truly inclusive and user-centric digital experiences that benefit everyone.