AI tools for improving the speed and quality of product design are revolutionizing the industry. No longer a futuristic fantasy, artificial intelligence is actively streamlining workflows, enhancing creative output, and pushing the boundaries of what’s possible in product development. This exploration delves into the practical applications of AI, showcasing how it’s accelerating design processes, improving product quality, and fostering more efficient collaboration across teams.
From automating repetitive tasks to predicting material performance, AI is reshaping the entire product design lifecycle.
This article will examine specific AI-powered tools, analyze their impact on various industries, and explore the ethical considerations surrounding their implementation. We’ll also investigate real-world case studies demonstrating the tangible benefits of AI integration, offering a clear picture of how businesses can leverage these technologies to gain a competitive edge. The future of product design is undeniably intertwined with AI, and understanding its capabilities is crucial for staying ahead of the curve.
Introduction
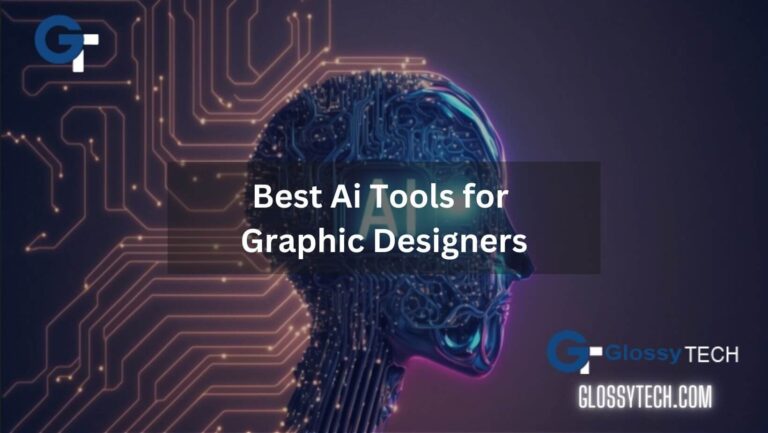
The product design landscape is undergoing a rapid transformation, driven by increasing market demands for faster turnaround times and higher quality products. Traditional design processes often struggle to meet these simultaneous requirements, leading to bottlenecks, compromised designs, and missed market opportunities. The pressure to innovate quickly and efficiently is immense, forcing companies to explore new methods to streamline their workflows and enhance their design capabilities.
AI is emerging as a powerful tool to address these challenges, offering the potential to significantly improve both the speed and quality of product design across diverse industries.AI’s impact on product design is multifaceted, extending beyond simple automation. It’s not just about speeding up existing processes; it’s about fundamentally changing how designers approach their work, enabling them to explore a wider range of possibilities and create more innovative and user-centric products.
AI algorithms are now capable of assisting with various stages of the design process, from initial ideation and concept generation to prototyping, testing, and manufacturing. This integrated approach allows for a more streamlined and efficient design workflow, resulting in faster time-to-market and improved product quality.
AI’s Impact on Key Areas of Product Design
AI is revolutionizing several key areas within product design. For example, generative design algorithms can explore countless design variations based on specified parameters, significantly expanding the design space and identifying optimal solutions that might be missed by human designers alone. AI-powered tools can also automate repetitive tasks, such as rendering and 3D modeling, freeing up designers to focus on more creative and strategic aspects of the project.
Furthermore, AI can analyze vast amounts of user data to inform design decisions, ensuring that products are tailored to meet specific customer needs and preferences. This data-driven approach leads to more successful product launches and improved user satisfaction.
Industries Benefiting from AI-Powered Design Tools
The automotive industry is a prime example of an industry significantly benefiting from AI-driven design tools. Automakers are leveraging AI to optimize vehicle aerodynamics, improve crash safety, and personalize interior design based on user preferences. Similarly, the aerospace industry uses AI to design lighter, stronger, and more fuel-efficient aircraft components. The consumer electronics sector is also rapidly adopting AI-powered design tools to create more aesthetically pleasing and functional products, while the fashion industry utilizes AI for trend forecasting and personalized garment design.
These are just a few examples; the application of AI in product design is expanding rapidly across various sectors.
AI-Powered Tools for Accelerated Design Processes
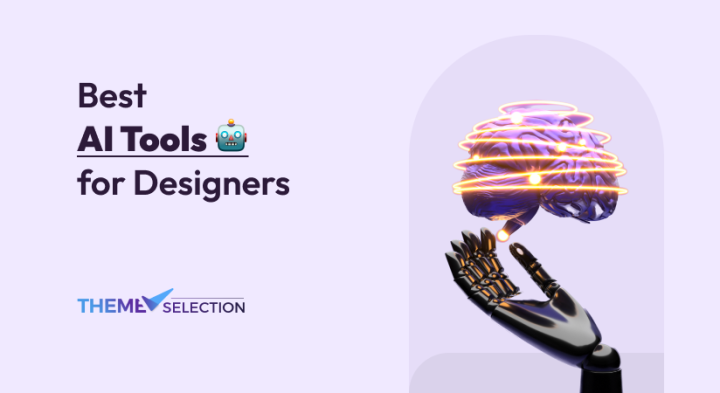
AI is rapidly transforming the product design landscape, offering powerful tools to significantly accelerate design processes and enhance overall quality. These tools leverage machine learning and artificial intelligence to automate repetitive tasks, optimize designs, and generate innovative solutions, ultimately reducing time-to-market and improving product competitiveness. This section will delve into specific AI-powered tools and explore how AI algorithms contribute to faster and more efficient design iterations.
AI Tools Automating Repetitive Design Tasks
Several AI-powered tools are available to streamline the design process by automating repetitive tasks such as generating design variations, optimizing layouts, and even creating initial design concepts. This automation frees up designers to focus on more creative and strategic aspects of the design process, leading to increased productivity and improved overall design quality. The following table compares four such tools:
Feature | Tool A (e.g., Autodesk Generative Design) | Tool B (e.g., Procreate with AI features) | Tool C (e.g., Adobe Sensei) | Tool D (e.g., Midjourney) |
---|---|---|---|---|
Design Variation Generation | Generates multiple design options based on specified parameters and constraints. | Offers AI-powered brushes and tools for quick variations and stylization. | Provides intelligent features for image upscaling, background removal, and content-aware fill. | Creates variations of images based on text prompts. |
Layout Optimization | Optimizes layouts for functionality, aesthetics, and manufacturability. | Provides tools for efficient canvas organization and layer management. | Offers automated layout adjustments and alignment features. | Less direct layout optimization, but image generation can be used to create layout components. |
Repetitive Task Automation | Automates tasks like parameter sweeps and design exploration. | Automates simple repetitive actions through customizable gestures and shortcuts. | Automates tasks such as image resizing and batch processing. | Automates image generation based on text prompts, allowing for rapid iteration. |
Pricing | Subscription-based, varying based on features and usage. | One-time purchase or subscription, depending on the device and features. | Integrated into Adobe Creative Cloud subscription. | Subscription-based, offering different tiers with varying features. |
Ease of Use | Requires some technical expertise. | Relatively user-friendly, intuitive interface. | Generally user-friendly, integrated into familiar Adobe workflow. | Relatively easy to use, text-based interface for image generation. |
Note: The specific features and pricing of these tools may vary over time. This table provides a general overview at the time of writing. The tools listed are examples and represent different approaches to AI in design.
AI Algorithms Accelerating Design Iteration
AI algorithms, particularly machine learning models, accelerate the design iteration process through several mechanisms. For example, generative adversarial networks (GANs) can be used to generate a wide range of design variations, allowing designers to quickly explore different possibilities. Reinforcement learning algorithms can optimize designs by iteratively testing different parameters and selecting the best performing options. These algorithms significantly reduce the time and effort required for manual exploration and optimization, leading to faster design cycles.
For instance, in automotive design, AI can rapidly explore thousands of aerodynamic configurations, identifying optimal shapes far more efficiently than traditional methods.
AI in Generative Design and Its Impact on Speed
Generative design, powered by AI, significantly impacts design speed by automating the exploration of a vast design space. Instead of relying solely on human intuition, generative design algorithms can explore numerous design possibilities based on specified constraints and objectives. This automated exploration leads to the discovery of innovative and optimized designs that might not have been considered through traditional methods.
The speed advantage is substantial, especially for complex designs with numerous variables and constraints. For example, in architectural design, generative design can optimize building structures for strength, weight, and cost, generating multiple design options within a fraction of the time it would take a human designer.
Enhancing Design Quality with AI
AI is revolutionizing product design by not only accelerating the process but also significantly enhancing the quality of the final product. Its ability to analyze vast datasets, identify patterns, and predict outcomes allows for earlier detection of flaws, more precise specifications, and ultimately, more aesthetically pleasing and user-friendly designs. This results in products that are not only faster to market but also better received by consumers.AI’s role in improving design quality extends across various stages of the design lifecycle, from initial concept to final production.
By leveraging machine learning algorithms and advanced computational power, AI tools can identify subtle issues that might be missed by human designers, leading to more robust and reliable products. This proactive approach to quality assurance minimizes costly revisions and delays later in the development process.
AI’s Role in Early Detection of Design Flaws
AI algorithms can analyze CAD models and simulations to identify potential structural weaknesses, ergonomic issues, or manufacturing challenges early in the design process. For example, AI-powered stress analysis tools can predict points of failure under various load conditions, allowing designers to reinforce vulnerable areas before prototyping. Similarly, AI can analyze user interface designs to identify potential usability problems, such as confusing navigation or inaccessible features, leading to more intuitive and user-friendly products.
This proactive approach reduces the need for costly and time-consuming redesigns later in the development cycle. A well-known example is the use of AI in automotive design to simulate crash tests and optimize vehicle safety features, reducing the need for extensive physical testing.
Improving the Accuracy and Precision of Design Specifications
AI enhances design precision by automating tasks such as generating detailed specifications from high-level design concepts. This ensures consistency and accuracy across the entire design process, reducing errors and misunderstandings between design teams and manufacturing facilities. For instance, AI can automatically generate precise dimensions and tolerances for manufactured parts, minimizing discrepancies between the design and the final product.
Furthermore, AI can optimize designs for manufacturability, considering factors such as material properties, manufacturing processes, and cost constraints, leading to more efficient and cost-effective production. This precision minimizes waste and improves overall product quality.
AI-Driven Tools for Enhanced Aesthetics and User Experience
Several AI-driven tools are available to enhance the aesthetic appeal and user experience of products. Generative design algorithms can explore a vast design space to create innovative and aesthetically pleasing solutions, often exceeding human creativity. AI-powered tools can also personalize designs based on user preferences and demographics, creating customized products that resonate with individual consumers. For example, AI can analyze user data to optimize the layout and functionality of a mobile app, improving its usability and engagement.
Another example is the use of AI in generating realistic renderings and visualizations, helping designers communicate their ideas more effectively and allowing clients to visualize the final product more accurately.
Case Study: AI-Enhanced Design of a Smartwatch
Imagine a smartwatch manufacturer using AI to improve its product design. Initially, the design team faced challenges in optimizing the smartwatch’s battery life while maintaining a sleek and aesthetically pleasing design. Using AI-powered simulation tools, the team could analyze various design iterations, predicting battery consumption under different usage scenarios. The AI identified a design flaw that was causing unexpected power drain, a flaw that human designers had missed.
The AI then suggested design modifications to improve thermal management and optimize power consumption, resulting in a 20% increase in battery life without compromising the aesthetic design. Furthermore, AI-powered user experience analysis tools helped refine the smartwatch’s interface, making it more intuitive and user-friendly, leading to higher customer satisfaction ratings.
AI for Material Selection and Optimization
Traditional material selection relies heavily on engineers’ experience, material databases, and iterative testing. This process can be time-consuming, expensive, and may not explore the full range of potential materials. AI-driven approaches offer a significant advancement by leveraging machine learning algorithms to analyze vast datasets, predict material performance, and optimize material usage for improved efficiency and design outcomes. This shift accelerates the design process and unlocks possibilities previously unattainable through traditional methods.AI-driven material selection leverages machine learning models trained on extensive material property databases, coupled with design requirements and constraints.
These models can predict material behavior under various conditions, facilitating faster and more informed decision-making. Unlike traditional methods which often involve trial and error, AI can efficiently analyze numerous material options simultaneously, identifying optimal candidates based on predefined criteria, such as strength-to-weight ratio, cost, sustainability, and manufacturability. This data-driven approach significantly reduces the reliance on intuition and accelerates the iterative design process.
AI Tools for Material Performance Prediction, AI tools for improving the speed and quality of product design
Several AI tools are emerging to predict material performance and optimize material usage. These tools utilize machine learning algorithms, such as neural networks and support vector machines, to analyze complex relationships between material properties and performance characteristics. For example, software incorporating AI can predict the fatigue life of a component based on its material properties, geometry, and loading conditions, thereby enabling designers to choose materials that meet specific durability requirements.
Another example involves predicting the corrosion resistance of a material in a specific environment, allowing for the selection of materials that minimize the risk of degradation. These predictive capabilities drastically reduce the need for extensive physical testing, leading to significant cost and time savings. Furthermore, some tools integrate with CAD software, allowing for seamless integration of material selection within the design process.
Benefits of AI-Driven Material Selection
The integration of AI into material selection offers numerous advantages. A significant benefit is the accelerated design process, as AI can quickly evaluate numerous material options and identify optimal candidates. This leads to considerable time and cost savings compared to traditional methods. Furthermore, AI can uncover novel material combinations and designs that may not have been considered using traditional approaches.
This leads to improved design performance and the exploration of innovative material solutions.
- Faster Design Cycles: AI significantly reduces the time required for material selection, accelerating the overall design process.
- Cost Reduction: Reduced reliance on physical testing and prototyping leads to substantial cost savings.
- Improved Material Performance: AI can identify optimal material combinations for enhanced performance characteristics.
- Enhanced Design Innovation: AI can uncover novel material solutions and design concepts that may not have been considered through traditional methods.
- Sustainable Material Selection: AI can assist in selecting environmentally friendly materials, promoting sustainable design practices.
AI and Collaboration in Product Design
AI is revolutionizing collaboration in product design, breaking down traditional silos and fostering a more efficient and innovative design process. By integrating AI-powered tools, teams can streamline communication, accelerate feedback loops, and ultimately deliver higher-quality products in shorter timeframes. This enhanced collaboration extends across the entire product lifecycle, from initial concept to final manufacturing.AI facilitates seamless information sharing and real-time collaboration among designers, engineers, and manufacturers.
This integration significantly reduces misunderstandings and delays commonly associated with traditional communication methods. For example, AI-driven platforms can automatically translate design specifications into manufacturing instructions, eliminating the need for manual interpretation and reducing the risk of errors. This shared understanding accelerates the overall design process and minimizes costly rework.
Real-time Feedback and Design Revisions Enabled by AI
AI tools provide real-time feedback on design iterations, enabling rapid design revisions and improvements. For instance, generative design software can instantly analyze design parameters and suggest optimal solutions based on specified constraints. This immediate feedback loop allows designers to explore multiple options quickly, identifying potential flaws and optimizing designs before they are finalized. Furthermore, AI-powered simulation tools can accurately predict the performance of a design under various conditions, providing valuable insights for informed decision-making.
This accelerated feedback cycle significantly shortens the overall design process and improves the quality of the final product. Imagine a scenario where a structural engineer can instantly see the stress points on a bridge design based on AI analysis and suggest immediate modifications, reducing costly errors and potential safety risks.
Impact of AI-Driven Communication Tools on Project Timelines and Efficiency
AI-driven communication tools significantly impact project timelines and overall efficiency by streamlining information flow and reducing communication bottlenecks. These tools can automate routine tasks such as progress reporting and design change management, freeing up team members to focus on more creative and strategic aspects of the project. For example, AI-powered chatbots can answer frequently asked questions, providing instant support to team members and reducing the reliance on email or in-person meetings.
This increased efficiency translates to faster project completion times and improved resource allocation. A study by a major automotive manufacturer showed a 15% reduction in project timelines after implementing AI-driven communication tools in their product design process, demonstrating a tangible impact on efficiency.
Case Studies
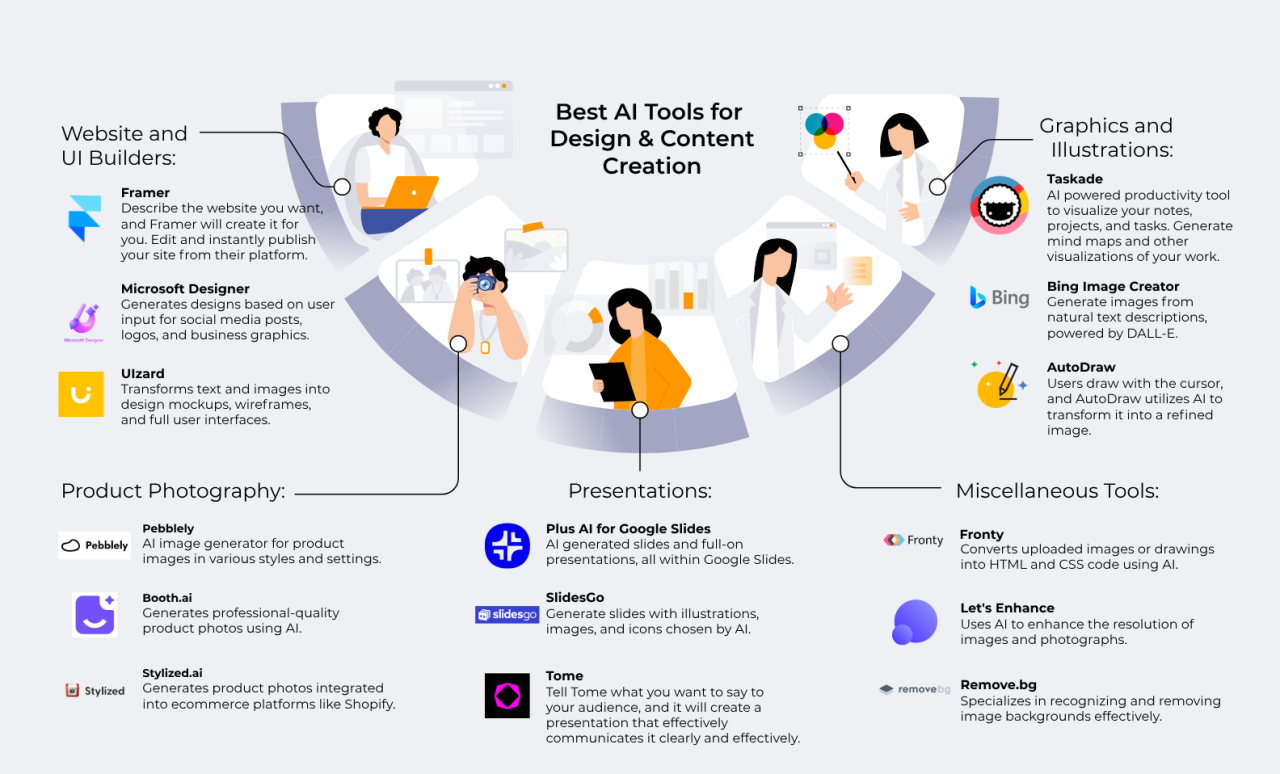
The successful integration of AI in product design is no longer a futuristic concept; it’s a reality transforming industries. These case studies demonstrate the tangible benefits achieved through the adoption of AI-powered tools, highlighting both the challenges overcome and the significant improvements in speed, quality, and efficiency. The examples below represent diverse sectors, illustrating the broad applicability of AI across the product design landscape.
Examining real-world applications reveals the power of AI to streamline design processes, optimize material usage, and foster collaboration, ultimately leading to superior products and reduced time-to-market.
AI-Driven Optimization in Automotive Design
A leading automotive manufacturer leveraged AI to optimize the aerodynamic design of a new electric vehicle. The goal was to improve efficiency and range while maintaining aesthetic appeal.
- Challenge: Balancing aerodynamic performance with design aesthetics proved challenging using traditional methods. Extensive wind tunnel testing and iterative design adjustments were time-consuming and costly.
- AI Solution: The company employed a generative design AI tool that explored a vast design space, generating thousands of potential aerodynamic shapes. The AI was trained on a dataset of successful vehicle designs and aerodynamic principles. It then evaluated each design based on pre-defined criteria, such as drag coefficient, lift, and visual appeal.
- Results: The AI identified a design that improved aerodynamic efficiency by 15% compared to the initial design, resulting in a projected 20% increase in vehicle range. The process was significantly faster than traditional methods, reducing development time by approximately 40%.
AI-Assisted Material Selection in Aerospace Engineering
An aerospace company utilized AI to select optimal materials for a new aircraft component, focusing on weight reduction and enhanced structural integrity.
- Challenge: Finding the ideal material combination that met stringent weight, strength, and cost requirements was a complex task, requiring extensive material testing and simulations.
- AI Solution: The company used an AI-powered material selection tool that analyzed a vast database of material properties and performance characteristics. The AI considered various factors, including material strength, density, cost, and manufacturing feasibility. The system used machine learning algorithms to predict the performance of different material combinations under various stress conditions.
- Results: The AI identified a novel material combination that reduced the component’s weight by 20% without compromising structural integrity. This weight reduction translated to significant fuel savings and improved aircraft performance. The AI-driven approach also reduced the time required for material selection by 60%.
Generative Design for Consumer Electronics
A consumer electronics manufacturer employed AI-powered generative design software to develop a new smartphone case.
- Challenge: Designing a case that was both aesthetically pleasing and structurally robust, while minimizing material usage and manufacturing costs, presented a significant challenge.
- AI Solution: The company utilized generative design software to explore a wide range of design possibilities based on predefined parameters, such as material strength, weight, and manufacturing constraints (e.g., injection molding). The AI generated numerous design options, which were then evaluated based on these parameters and aesthetic considerations.
- Results: The AI-generated design was 15% lighter and 20% stronger than the initial design, leading to significant cost savings in materials and manufacturing. The design also received positive feedback from focus groups for its improved aesthetics.
Future Trends and Challenges in AI-Driven Product Design: AI Tools For Improving The Speed And Quality Of Product Design
The integration of artificial intelligence (AI) into product design is rapidly evolving, promising significant advancements in speed, efficiency, and creativity. However, this transformative technology also presents unique challenges and ethical considerations that require careful attention. Understanding both the potential and the pitfalls is crucial for harnessing the full power of AI in this field.The convergence of AI and product design is poised for exponential growth.
Emerging trends indicate a move towards more sophisticated AI algorithms capable of handling complex design problems and incorporating user feedback in real-time. This will lead to personalized product experiences and more efficient design iterations. Furthermore, the increasing accessibility of AI tools through cloud-based platforms will democratize access to advanced design capabilities, empowering smaller companies and individual designers.
Emerging Trends in AI-Powered Design Tools and Technologies
The field is witnessing a rapid evolution of AI capabilities, moving beyond simple automation to encompass generative design, predictive analytics, and advanced simulation. Generative design algorithms, for instance, can explore a vast design space, generating numerous options based on specified constraints and objectives. This surpasses human capabilities in exploring unconventional solutions. Simultaneously, the integration of AI-powered predictive analytics allows for more accurate forecasting of product performance and market demand, reducing the risk of costly failures.
Advanced simulation tools, fueled by AI, enable designers to test and refine their designs virtually, significantly reducing the need for costly physical prototypes. For example, companies like Autodesk are already integrating generative design capabilities into their software, allowing engineers to explore thousands of design options within minutes, significantly accelerating the design process.
Challenges and Limitations of AI Adoption in Product Design
Despite the immense potential, the widespread adoption of AI in product design faces several significant hurdles. Data dependency is a major challenge; AI algorithms require large, high-quality datasets for training and accurate predictions. The lack of readily available, appropriately labeled data in certain design niches can hinder AI implementation. Furthermore, the “black box” nature of some AI algorithms makes it difficult to understand their decision-making processes, leading to a lack of trust and transparency, especially crucial in safety-critical design applications.
Another significant challenge is the need for skilled professionals who can effectively integrate and manage AI tools within existing design workflows. This requires upskilling and reskilling initiatives to bridge the gap between AI capabilities and human expertise. For example, the automotive industry, while embracing AI for design optimization, still relies heavily on experienced engineers to validate and interpret AI-generated designs.
Ethical Considerations in AI-Driven Creative Fields
The use of AI in creative fields like product design raises important ethical questions. One major concern is the potential displacement of human designers. While AI can augment human capabilities, there’s a risk of job losses if AI tools become overly sophisticated. Another crucial aspect is the issue of intellectual property rights. Determining ownership of designs generated by AI algorithms remains a complex legal and philosophical challenge.
Finally, the potential for algorithmic bias is a significant ethical concern. If the training data reflects existing biases, the AI system may perpetuate and amplify these biases in its design outputs, leading to unfair or discriminatory outcomes. For instance, AI-generated designs could inadvertently reinforce existing gender or cultural stereotypes if the training data lacks diversity and representation.
Addressing these ethical concerns requires a multi-faceted approach, involving industry collaboration, regulatory frameworks, and a commitment to responsible AI development.
Closure
The integration of AI in product design isn’t merely a trend; it’s a fundamental shift in how products are conceived, developed, and brought to market. By automating tedious tasks, enhancing design quality, and fostering seamless collaboration, AI tools are empowering designers and engineers to achieve previously unimaginable levels of efficiency and innovation. While challenges and ethical considerations remain, the potential benefits are undeniable, promising a future where product design is faster, more efficient, and more responsive to evolving market demands.
Embracing AI is no longer a choice but a necessity for businesses aiming for sustainable growth and competitive dominance in the dynamic landscape of modern product development.