AI tools for removing background noise in video editing are revolutionizing post-production. No longer are editors chained to painstaking manual noise reduction; AI offers speed, efficiency, and often superior results. This technology leverages sophisticated algorithms, primarily deep learning models, to intelligently identify and eliminate unwanted sounds, preserving the integrity of the desired audio. This guide explores the capabilities, applications, and future of this transformative technology.
From identifying subtle hums to tackling disruptive roars, AI-powered tools analyze audio spectrograms, identifying patterns indicative of noise. They then apply targeted processing, effectively separating noise from clean audio. This contrasts sharply with traditional methods like noise gates or spectral editing, which often require extensive manual tweaking and may compromise audio quality. The result? Cleaner audio, faster workflows, and a higher-quality final product, regardless of whether you’re a professional filmmaker or a passionate vlogger.
Introduction to AI-Powered Noise Reduction in Video Editing
Video editing often involves wrestling with unwanted background noise – the hum of a refrigerator, the rumble of traffic, or the distracting chatter of a nearby conversation. These audio imperfections can significantly detract from the overall quality of a video, making it challenging to deliver a polished and professional final product. Traditional methods of noise reduction, while functional, often lack the precision and efficiency required for complex audio landscapes.
This is where AI-powered noise reduction tools offer a significant advancement, transforming the video editing workflow.The limitations of traditional noise reduction techniques are considerable. Methods like spectral subtraction, for instance, can lead to artifacts and audio distortion, especially when dealing with subtle background noise or complex audio signals. Manual noise gate adjustments are time-consuming and require a high degree of expertise.
Moreover, these techniques struggle with unpredictable or dynamic noise sources. AI, on the other hand, offers a powerful alternative, capable of handling these challenges with greater accuracy and speed.
Advantages of AI-Powered Noise Reduction
AI-based noise reduction leverages machine learning algorithms trained on vast datasets of audio, enabling it to identify and isolate noise with remarkable precision. This results in cleaner audio with significantly fewer artifacts compared to traditional methods. Furthermore, AI algorithms can adapt to different types of noise and audio environments, providing a more robust and versatile solution. The automation offered by AI also dramatically reduces the time and effort required for noise reduction, freeing up editors to focus on other aspects of the video production process.
This translates to increased efficiency and potentially lower production costs.
A Brief History of AI in Audio and Video Processing
The application of AI in audio and video processing has evolved rapidly in recent years. Early attempts focused on simple noise reduction algorithms, but the advent of deep learning has revolutionized the field. Convolutional Neural Networks (CNNs) and Recurrent Neural Networks (RNNs) have proven particularly effective in tackling complex audio challenges, including noise reduction, audio separation, and speech enhancement.
Initially, AI-powered tools were primarily used in professional studios, but their accessibility and affordability have increased significantly, making them readily available to amateur and independent video editors. The development of cloud-based AI processing has further accelerated this trend, enabling even users with limited computational resources to access powerful noise reduction capabilities.
Types of AI Noise Reduction Tools
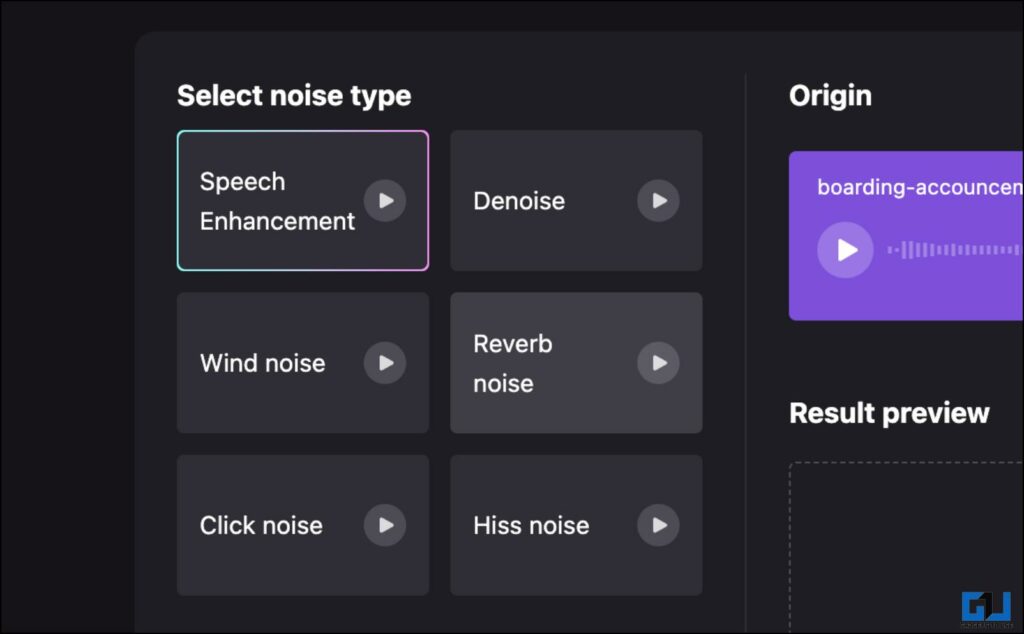
AI-powered noise reduction tools for video editing are transforming the post-production workflow, offering efficient and effective solutions to eliminate unwanted audio and visual disturbances. These tools leverage various algorithms and processing methods, categorized primarily by their application: real-time processing for live streaming or recording, and post-processing for editing existing footage. Understanding these distinctions is crucial for selecting the appropriate tool for specific needs.
The core difference lies in the timing of noise reduction. Real-time tools process audio and video concurrently with capture, requiring minimal latency. Post-processing tools, on the other hand, analyze and clean the footage after recording, allowing for more sophisticated algorithms and potentially higher quality results but at the cost of increased processing time.
Real-time vs. Post-Processing AI Noise Reduction
Real-time AI noise reduction is essential for live streaming, video conferencing, and situations where immediate processing is crucial. These tools often employ lighter algorithms to minimize latency, prioritizing speed over absolute noise reduction. Post-processing tools, used during video editing, can employ more computationally intensive algorithms to achieve superior results, as processing time is not a critical constraint. This allows for the use of advanced deep learning models that can more effectively identify and remove subtle noise artifacts.
The choice between real-time and post-processing depends on the application; live broadcasts benefit from real-time processing, while film editing often favors the superior quality of post-processing.
Algorithms Used in AI Video Noise Reduction
AI-powered noise reduction tools utilize a variety of algorithms, primarily focusing on machine learning and deep learning approaches. Machine learning algorithms, such as Support Vector Machines (SVMs) and Random Forests, can effectively learn patterns in noise and signal, enabling separation and reduction of unwanted sounds. However, deep learning algorithms, especially Convolutional Neural Networks (CNNs) and Recurrent Neural Networks (RNNs), have shown superior performance in complex noise reduction tasks.
CNNs excel at identifying spatial patterns in images and videos, while RNNs are adept at handling temporal dependencies in audio. Deep learning models can be trained on massive datasets of noisy and clean audio and video, leading to highly accurate noise reduction. For instance, a CNN might learn to identify and remove the consistent hiss of a microphone, while an RNN could target the intermittent pops and clicks caused by handling equipment.
Comparison of Popular AI Video Noise Reduction Tools
The following table compares several popular AI-powered video noise reduction tools based on their key features, pricing, and platform compatibility. Note that pricing and features are subject to change.
Tool Name | Key Features | Pricing | Platform Compatibility |
---|---|---|---|
Adobe Premiere Pro (with AI features) | AI-powered noise reduction for both audio and video, integrated into a comprehensive video editing suite. | Subscription-based, various tiers available. | Windows, macOS |
DaVinci Resolve (with AI features) | Offers powerful AI-based noise reduction and other AI-assisted tools within a professional-grade video editing application. | Free and paid versions available. | Windows, macOS, Linux |
Topaz Video AI | Specialized AI noise reduction tool focusing on enhancing video quality, including noise reduction, upscaling, and sharpening. | One-time purchase or subscription. | Windows, macOS |
Neat Video | A long-standing plugin offering advanced noise reduction capabilities, integrating with various video editing software. | One-time purchase. | Windows, macOS |
How AI Noise Reduction Tools Work
AI-powered noise reduction tools leverage sophisticated algorithms to intelligently differentiate between desired audio (speech, music) and unwanted background noise. This process involves several key steps, from initial analysis to final output, and relies heavily on machine learning techniques to achieve high-quality results. The effectiveness of these tools hinges on the underlying AI models and the user’s ability to adjust various parameters.The process typically begins with importing the video file into the chosen software.
The software then analyzes the audio track, identifying patterns and characteristics of both the desired audio and the background noise. This analysis utilizes machine learning models, often trained on vast datasets of audio samples, to create a detailed representation of the audio spectrum. Subsequently, the AI applies algorithms to selectively attenuate or remove the identified noise, while attempting to preserve the integrity of the desired audio.
Finally, the processed audio is integrated back into the video, and the user can export the cleaned video file.
AI Techniques for Noise Reduction
AI noise reduction tools primarily utilize deep learning techniques, specifically convolutional neural networks (CNNs) and recurrent neural networks (RNNs), to achieve effective noise reduction. CNNs are particularly adept at analyzing the spatial characteristics of audio signals, identifying patterns and textures within the sound. RNNs, on the other hand, excel at processing sequential data, which is crucial for capturing temporal dependencies in audio signals.
These models are trained on massive datasets of audio recordings, learning to distinguish between different types of noise and desired audio components. The training process involves feeding the network with numerous examples of noisy audio and their corresponding clean versions, allowing the network to learn the mapping between the two. Once trained, the model can then process new audio inputs, identifying and removing noise based on its learned patterns.
Parameter Adjustment and its Impact
Various parameters within AI noise reduction tools allow users to fine-tune the noise reduction process. These parameters often include noise reduction strength, frequency range, and spectral smoothing. Increasing the noise reduction strength aggressively removes more noise but also risks introducing artifacts or unwanted changes to the desired audio. Adjusting the frequency range allows focusing on specific frequency bands where noise is more prominent, preventing unnecessary alterations to other frequency ranges.
Spectral smoothing helps to reduce harshness and artifacts introduced during the noise reduction process. For instance, a setting that prioritizes speech clarity might emphasize preserving the mid-range frequencies essential for vocal intelligibility, even at the cost of slightly less reduction in background hum. Conversely, a setting focused on musical fidelity might preserve high-frequency details, even if it means leaving some low-level background noise.
The optimal settings depend heavily on the type of audio, the nature of the noise, and the desired outcome. Experimentation and careful listening are key to achieving the best results.
Practical Applications and Use Cases: AI Tools For Removing Background Noise In Video Editing
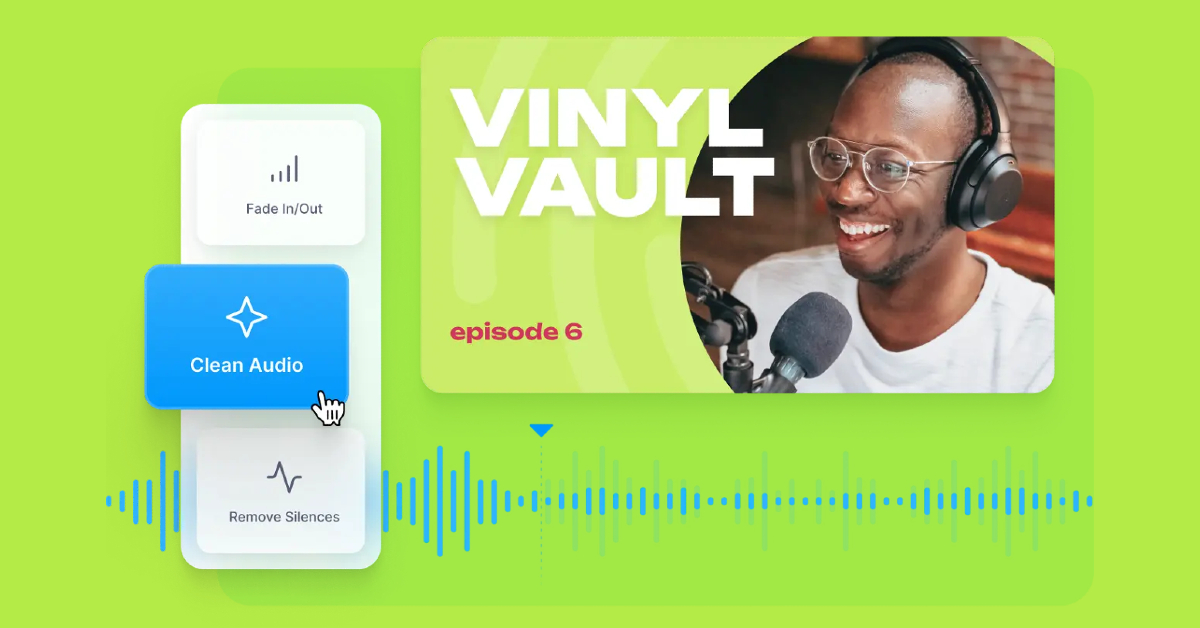
AI-powered noise reduction tools have revolutionized video editing, offering significant improvements across various production scenarios. These tools are no longer niche technologies; they’ve become integral to professional and amateur workflows, enhancing both the efficiency and quality of video production. Their applications span a wide range, from high-budget filmmaking to everyday vlogging and live streaming.The benefits extend beyond mere noise reduction.
By eliminating distracting background sounds, these tools enhance clarity, improve audience engagement, and streamline the post-production process, saving editors valuable time and resources. This leads to a more polished final product, regardless of the scale of the project or the editor’s experience level.
Filmmaking Applications
In filmmaking, AI noise reduction is invaluable for cleaning up audio recorded on location. Imagine a scene shot outdoors, where wind noise or distant traffic interferes with dialogue. AI tools can effectively isolate and reduce these unwanted sounds, preserving the clarity of the actors’ voices without sacrificing the ambiance of the scene. For example, a scene filmed near a busy street might initially sound muddy and unintelligible.
After applying an AI noise reduction tool, the dialogue becomes clear and crisp, allowing the audience to fully appreciate the emotional impact of the scene. The visual difference would be subtle, reflected only in the improved clarity of the audio track; the image itself would remain unchanged. A before-and-after comparison would show a significant reduction in audio waveform amplitude in the frequency ranges associated with the unwanted noise.
Vlogging and Live Streaming Enhancements
Vloggers and live streamers often record in less-than-ideal acoustic environments. A cluttered room, background chatter, or even the hum of a computer can significantly detract from the viewing experience. AI noise reduction tools offer a simple solution. Consider a vlogger recording in their home office, with the constant hum of a refrigerator and the occasional distant siren. After applying noise reduction, the video audio would be significantly cleaner, focusing the audience’s attention on the vlogger’s voice and message.
Visually, the improvement would be primarily in the audio quality, indicated by a cleaner audio waveform in a before-and-after comparison. The video itself would appear unchanged.
Professional Workflow Integration
In professional video editing workflows, AI noise reduction tools are often integrated into larger post-production pipelines. They are used to prepare audio for further processing such as audio sweetening and sound design, allowing editors to focus on more creative aspects of the project. Consider a documentary filmmaker working with archival footage. The original audio might be severely degraded due to age and poor recording quality.
AI noise reduction can help to restore some of the intelligibility of the audio, making it easier to transcribe and incorporate into the final documentary. A before-and-after comparison would reveal a smoother, less grainy audio waveform, with a reduction in background hiss and crackle.
Amateur Video Editing Benefits, AI tools for removing background noise in video editing
Even amateur video editors can benefit significantly from AI noise reduction tools. These tools lower the barrier to entry for high-quality video production, enabling individuals with limited budgets or experience to create professional-sounding videos. For example, a student creating a short film for a class project might record dialogue in a noisy environment. Using an AI noise reduction tool, they can significantly improve the audio quality, resulting in a more polished and professional final product.
A visual comparison would again show the improvement in the audio waveform, demonstrating the effectiveness of the tool in reducing background noise without affecting the video quality.
Limitations and Future Trends
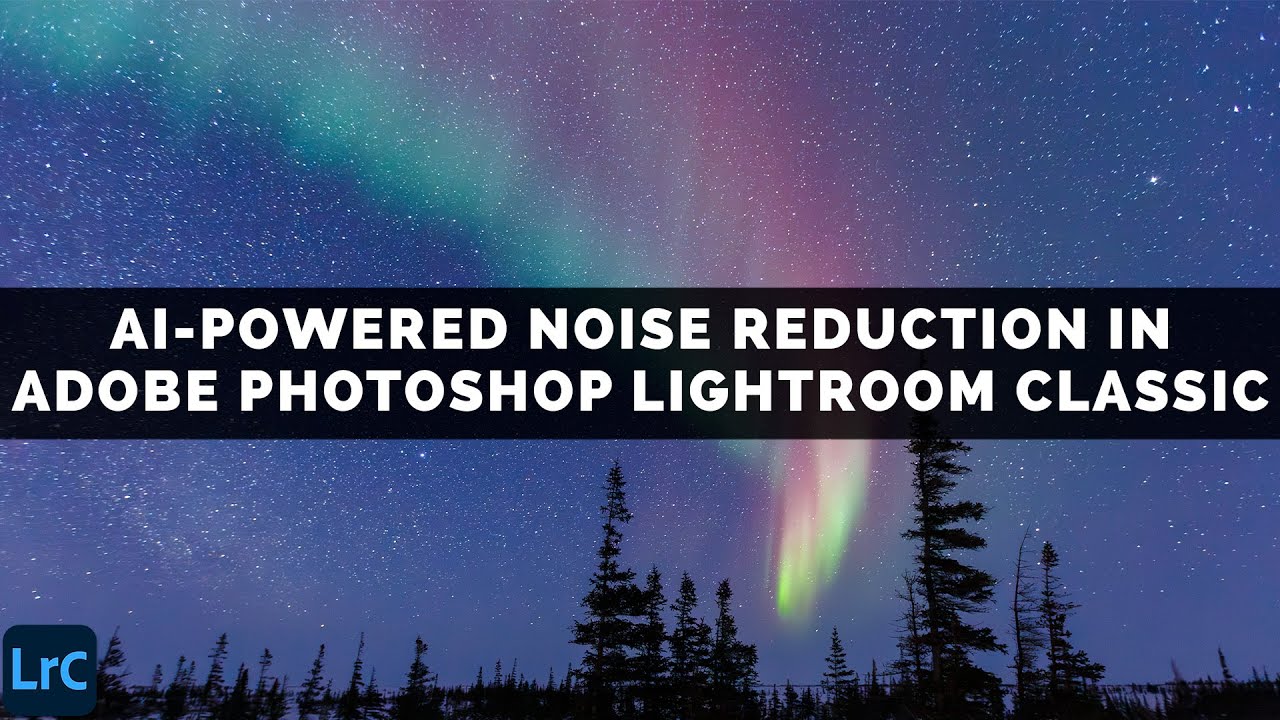
Current AI-powered video noise reduction tools, while impressive, are not without their limitations. These limitations stem from the inherent complexities of video data, the ongoing evolution of AI algorithms, and the diverse nature of noise itself. Understanding these constraints is crucial for appreciating the potential of future advancements in this field.While AI significantly improves video quality, perfect noise removal remains elusive.
The algorithms often struggle to differentiate between actual noise and fine details within the video, potentially leading to unintended artifacts or a loss of subtle textures and sharpness. This is especially noticeable in high-resolution videos where fine details are more prominent. Furthermore, the computational resources required for advanced noise reduction can be substantial, impacting processing time and accessibility for users with less powerful hardware.
Limitations of Current AI Noise Reduction Technology
Current AI noise reduction technology faces several challenges. One significant limitation is the difficulty in distinguishing between noise and important video details. For example, removing grain from a film might unintentionally smooth out subtle textures in clothing or skin, resulting in a loss of realism. Another challenge is the computational cost. Processing high-resolution videos with complex AI models can require significant computing power and time, limiting the practicality for users with less powerful hardware or those working on tight deadlines.
Finally, the effectiveness of AI noise reduction can vary greatly depending on the type and characteristics of the noise present in the video. Different algorithms perform better with different types of noise, making it difficult to find a one-size-fits-all solution. For instance, algorithms trained on high-frequency noise might struggle with low-frequency hum.
Potential Future Improvements and Developments
Future developments in AI-powered video noise reduction are likely to focus on several key areas. Improved algorithms will be designed to better differentiate between noise and fine details, reducing artifacts and preserving image quality. This could involve the development of more sophisticated deep learning models with enhanced capabilities for feature extraction and noise discrimination. Researchers are also exploring more efficient algorithms that reduce computational demands, making advanced noise reduction accessible to a wider range of users.
This could involve optimizing existing algorithms or developing entirely new architectures that are computationally less intensive. Finally, advancements in adaptive noise reduction techniques will allow for more customized noise removal based on the specific characteristics of the video and the type of noise present. This personalized approach will lead to higher quality results and a more refined user experience.
Hypothetical Future AI Video Noise Reduction Tool: “ClarityAI”
Imagine a future AI video noise reduction tool called “ClarityAI.” This tool would go beyond simple noise reduction, offering several advanced features. ClarityAI would utilize a multi-stage, adaptive noise reduction process, analyzing the video’s content to identify and selectively remove different types of noise without sacrificing detail. It would employ a novel deep learning architecture, significantly reducing processing time compared to current tools, while maintaining superior noise reduction capabilities.
Furthermore, ClarityAI would include a customizable noise profile editor, allowing users to fine-tune the noise reduction process based on their specific needs. Users could adjust parameters to control the level of grain reduction, the preservation of fine details, and the overall aesthetic of the final video. The tool would also incorporate advanced denoising techniques for different video formats and resolutions, optimizing the process for each individual input.
Finally, ClarityAI would offer a preview mode allowing users to see the impact of different noise reduction settings in real-time before final processing, ensuring the best possible results are achieved. The tool’s advanced algorithms would also be able to identify and correct for various forms of noise including digital noise, wind noise, background hum, and even camera shake, offering a comprehensive noise reduction solution.
Comparison of AI vs. Traditional Noise Reduction Methods
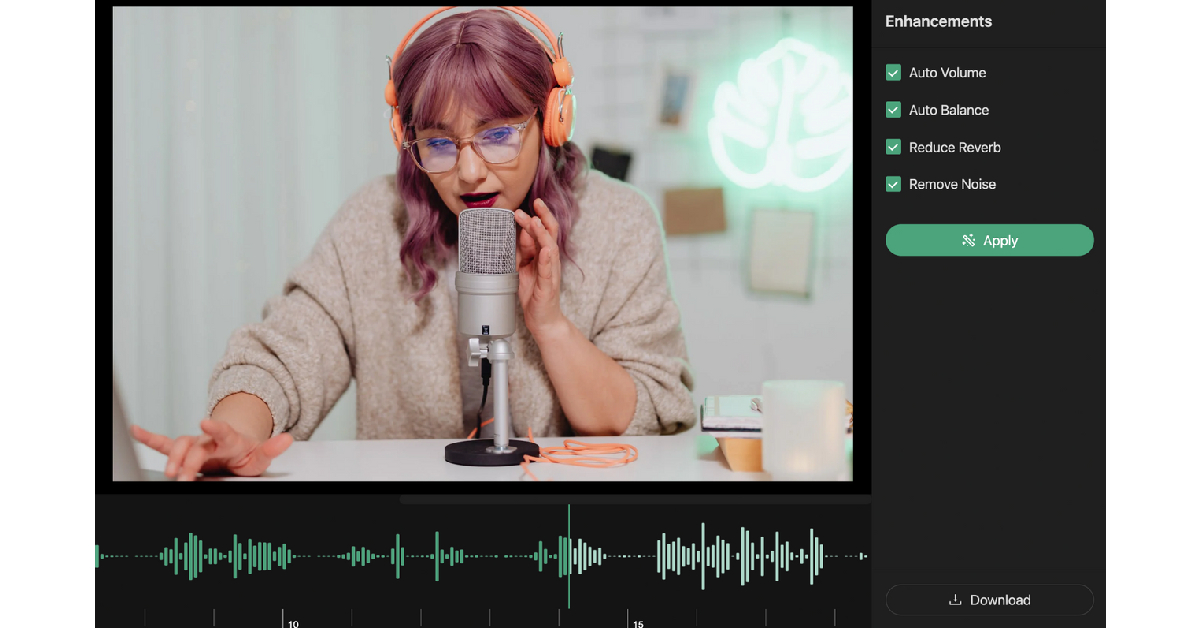
AI-powered noise reduction has revolutionized video editing, offering significant improvements over traditional methods. However, understanding the strengths and weaknesses of each approach is crucial for choosing the right tool for a specific project. This comparison examines the key differences between AI and traditional techniques, focusing on efficiency, results, and resource requirements.
Traditional noise reduction techniques, primarily spectral editing and noise gates, rely on manual adjustments and algorithmic rules based on frequency analysis. AI-powered methods, on the other hand, leverage machine learning to identify and remove noise intelligently, adapting to the specific characteristics of the audio or video.
Processing Time and Computational Resources
The processing time and computational resources needed differ substantially between AI and traditional methods. Traditional methods, such as spectral editing, are relatively fast for simple tasks but can become time-consuming for complex noise reduction. This is because they require meticulous manual adjustments and often involve multiple passes. Noise gates, while quicker, may introduce artifacts or unintended attenuation of desired audio elements.
AI-powered solutions, while initially requiring substantial training data and computational power for model development, offer faster processing times for actual noise reduction once the model is trained. The computational cost shifts from the user’s real-time effort to the upfront development and deployment of the AI model. A well-trained AI model can process large video files significantly faster than manual spectral editing, especially when dealing with complex noise profiles.
For example, a 10-minute video with significant background noise might take several hours to clean manually using spectral editing, while an AI-powered tool could complete the process in minutes.
Advantages and Disadvantages
A direct comparison reveals the unique strengths and limitations of both approaches.
- AI-Powered Noise Reduction:
- Advantages: Superior noise reduction capabilities, particularly for complex noise profiles; faster processing times for trained models; often produces more natural-sounding results; less user expertise required.
- Disadvantages: Requires significant computational resources for model training; can be expensive to implement; may struggle with unusual or unexpected noise types; potential for over-processing or unintended artifacts in complex scenarios; may require high-quality training data for optimal performance.
- Traditional Noise Reduction (Spectral Editing & Noise Gates):
- Advantages: Precise control over the noise reduction process; generally requires less computational power during processing; can be cost-effective for simple tasks; readily accessible in many video editing software packages.
- Disadvantages: Time-consuming and requires significant user expertise; prone to user error; can introduce artifacts; limited effectiveness against complex noise profiles; often requires multiple passes for satisfactory results.
Final Review
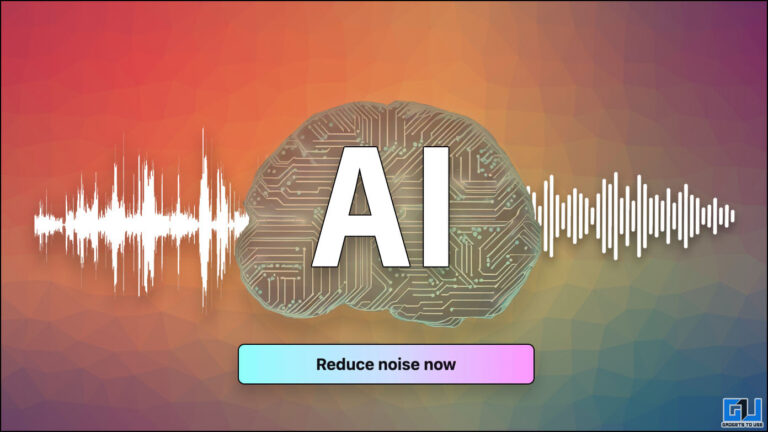
The rise of AI in video editing marks a significant leap forward in audio post-production. While current tools have limitations, ongoing development promises even more sophisticated and accurate noise reduction. The ability to quickly and effectively clean audio opens creative possibilities, allowing editors to focus on storytelling rather than tedious cleanup. As AI algorithms continue to refine their ability to distinguish between noise and desired audio, the future of video editing looks increasingly clear and—importantly—quiet.