Can AI accurately predict cryptocurrency price fluctuations? The question itself encapsulates the inherent volatility and complexity of the cryptocurrency market. While artificial intelligence offers powerful tools for analyzing vast datasets and identifying patterns, the unpredictable nature of cryptocurrencies, influenced by everything from regulatory changes to social media sentiment, presents a significant challenge. This exploration delves into the capabilities and limitations of AI in forecasting crypto prices, examining the algorithms used, data quality issues, and the ethical considerations involved.
We’ll dissect the strengths and weaknesses of various AI algorithms, comparing their performance against simpler methods. We’ll also investigate the crucial role of data quality, exploring the challenges of bias and noise in cryptocurrency datasets and how these affect prediction accuracy. Beyond technical analysis, we’ll consider the impact of macroeconomic factors, regulatory changes, and psychological influences on price movements, highlighting instances where these non-technical factors override even the most sophisticated AI predictions.
Finally, we’ll address the ethical implications of using AI for cryptocurrency price prediction, emphasizing the importance of responsible development and deployment.
Limitations of AI in Predicting Cryptocurrency Prices
Predicting cryptocurrency prices with AI, while seemingly promising, faces significant hurdles due to the inherent characteristics of these volatile markets and the limitations of current predictive modeling techniques. The complexity of the cryptocurrency ecosystem, coupled with the influence of unpredictable external factors, renders accurate price forecasting a challenging, if not impossible, task.
Inherent Volatility of Cryptocurrency Markets
Cryptocurrency markets are notoriously volatile, experiencing dramatic price swings in short periods. These fluctuations are driven by a multitude of factors, making it difficult for AI models, which rely on historical data and pattern recognition, to accurately predict future movements. Traditional financial markets, while also volatile, often exhibit more predictable patterns based on established economic principles and regulatory frameworks.
Cryptocurrency markets, however, are relatively new and largely unregulated, leading to amplified volatility and unpredictable behavior. This high volatility significantly impacts the reliability of AI predictions, as models trained on past data may not effectively extrapolate to future, drastically different market conditions. Small changes in market sentiment or news events can trigger disproportionately large price swings, rendering any predictive model prone to significant error.
Impact of Unpredictable News Events and Social Sentiment, Can AI accurately predict cryptocurrency price fluctuations?
News events, both positive and negative, can drastically influence cryptocurrency prices. A regulatory announcement, a major exchange hack, or even a prominent individual’s tweet can trigger immediate and substantial price changes. AI models struggle to incorporate these unpredictable events into their predictions, as these events often lack clear historical precedent and are difficult to quantify. Similarly, social sentiment, often expressed on social media platforms, plays a significant role in price fluctuations.
A sudden surge in positive sentiment can drive prices up, while negative sentiment can lead to sharp declines. Quantifying and integrating social sentiment data into AI models is challenging, leading to inaccuracies in price predictions.
Accuracy of AI Predictions Compared to Simpler Methods
While AI offers sophisticated modeling capabilities, its accuracy in predicting cryptocurrency prices isn’t necessarily superior to simpler forecasting methods. Moving averages, for instance, a basic technical analysis tool, can sometimes provide more reliable short-term predictions than complex AI algorithms. This is because moving averages smooth out short-term price fluctuations, focusing on broader trends. Complex AI models, on the other hand, can be overly sensitive to noise and outliers in the data, leading to inaccurate predictions.
The effectiveness of any forecasting method, AI-based or otherwise, depends heavily on the specific cryptocurrency, the time horizon of the prediction, and the overall market conditions.
Examples of Failed AI Price Predictions
Numerous instances demonstrate the limitations of AI in accurately predicting cryptocurrency prices. For example, in late 2017, several AI-powered platforms predicted continued growth in Bitcoin’s price, failing to anticipate the significant correction that followed. Similarly, numerous AI-driven trading bots experienced substantial losses during periods of extreme market volatility. These failures highlight the challenges of applying AI to a market characterized by unpredictable events and extreme price swings.
The inherent complexity and the inability to fully account for unpredictable external factors contribute to the inaccuracies of AI-based predictions.
Comparison of AI Algorithms and Success Rates
The success rate of different AI algorithms in predicting cryptocurrency price movements varies significantly, depending on factors such as the algorithm’s complexity, the dataset used for training, and the prediction horizon. A comprehensive evaluation requires extensive backtesting and rigorous validation across diverse market conditions. While precise success rates are difficult to quantify definitively due to the ever-changing nature of the cryptocurrency market, the following table provides a general comparison of commonly used algorithms.
Algorithm | Accuracy (General Estimate) | Strengths | Weaknesses |
---|---|---|---|
Long Short-Term Memory (LSTM) Networks | Moderate | Effective at capturing temporal dependencies in time series data | Sensitive to noise and overfitting; requires significant computational resources |
Support Vector Machines (SVM) | Low to Moderate | Relatively simple to implement and train | Performance can be affected by the choice of kernel function; less effective with high-dimensional data |
Random Forest | Low to Moderate | Robust to outliers and noise; relatively easy to interpret | Can be computationally expensive for large datasets |
Gradient Boosting Machines (GBM) | Moderate | High predictive accuracy; handles complex relationships well | Prone to overfitting if not properly tuned |
Data Sources and Quality for AI Prediction Models
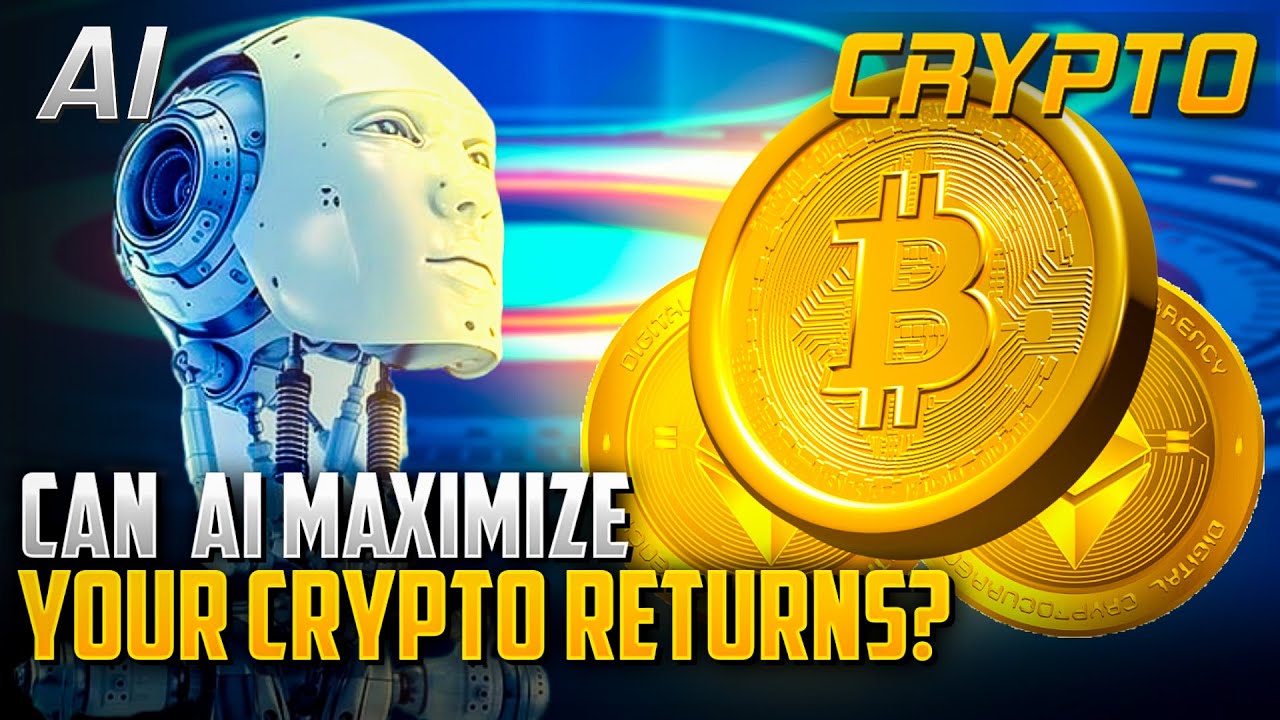
Accurately predicting cryptocurrency price fluctuations using AI relies heavily on the quality and diversity of the data used to train the models. The accuracy of any prediction is only as good as the data it’s based on; flawed data leads to flawed predictions. This section explores the various data sources employed, the inherent challenges in data quality, and methods for improving the reliability of these datasets.The effectiveness of AI in predicting cryptocurrency prices hinges on the comprehensiveness and accuracy of the input data.
AI models, whether employing machine learning or deep learning techniques, require substantial and diverse datasets to learn patterns and make accurate predictions. The lack of high-quality data is a significant hurdle in the development of reliable predictive models.
Data Sources for Cryptocurrency Price Prediction
AI models for cryptocurrency price prediction leverage a wide array of data sources. These sources can be broadly categorized into market data, on-chain data, and alternative data. Market data includes historical price information, trading volume, order book data, and market depth from various exchanges. On-chain data, directly extracted from the blockchain, includes transaction counts, transaction values, network hash rate, and the number of active addresses.
Alternative data encompasses social media sentiment analysis (measuring the overall positivity or negativity surrounding a cryptocurrency on platforms like Twitter), news articles, and even search trends. The combination of these data sources offers a more holistic view of market dynamics, potentially improving prediction accuracy. For instance, a surge in social media mentions alongside a rise in trading volume might signal a potential price increase.
Challenges of Data Quality and Bias in Cryptocurrency Datasets
Cryptocurrency datasets present unique challenges regarding data quality and bias. Data inconsistencies across exchanges are common, leading to discrepancies in price and volume figures. The decentralized nature of cryptocurrencies makes it difficult to obtain complete and reliable data. Furthermore, the market is susceptible to manipulation, with instances of wash trading (artificial inflation of trading volume) and pump-and-dump schemes that distort the true market signals.
Bias can also be introduced through the selection of data sources; relying solely on data from a single exchange might skew the results, as that exchange may not represent the overall market accurately.
Sources of Error and Noise in Cryptocurrency Data
Several factors contribute to error and noise in cryptocurrency data. Data inaccuracies stemming from reporting errors by exchanges are a significant source of noise. Flash crashes, periods of extreme volatility, and market manipulation introduce significant outliers that can negatively impact model training. The inherent volatility of the cryptocurrency market itself contributes to noise, making it challenging to identify genuine patterns amidst the fluctuations.
Furthermore, the lack of standardization in data reporting across different exchanges and platforms adds to the complexity.
Methods for Cleaning and Pre-processing Cryptocurrency Data
Data cleaning and pre-processing are crucial steps to improve the accuracy of AI prediction models. This involves handling missing values, smoothing noisy data, and removing outliers. Techniques like data imputation (filling in missing values based on existing data) and outlier detection algorithms (identifying and removing or adjusting extreme data points) are commonly employed. Data transformation techniques, such as normalization or standardization, can improve the performance of machine learning algorithms by scaling the data to a similar range.
Time series analysis techniques can help to identify trends and seasonality within the data, allowing for more accurate predictions.
Characteristics of High-Quality Cryptocurrency Data for AI Model Training
High-quality cryptocurrency data for AI model training should exhibit several key characteristics:
- Completeness: Minimal missing values, covering a sufficiently long time period and including all relevant variables.
- Accuracy: Data points accurately reflect real-world market activity, free from manipulation or errors.
- Consistency: Data from different sources should be consistent and comparable.
- Timeliness: Data should be updated frequently to reflect the dynamic nature of the cryptocurrency market.
- Relevance: Data should be relevant to the prediction task, avoiding unnecessary or irrelevant information.
- Representativeness: Data should represent the overall market, avoiding bias towards specific exchanges or trading strategies.
AI Algorithms and Their Applicability
Predicting cryptocurrency price fluctuations using AI involves employing various algorithms, each with its strengths and weaknesses. The choice of algorithm significantly impacts the accuracy and computational efficiency of the prediction model. Understanding these differences is crucial for developing effective predictive tools.
Several AI algorithms are applied to cryptocurrency price prediction, primarily falling under the umbrella of machine learning and deep learning. These approaches differ in their complexity, data handling capabilities, and susceptibility to the inherent volatility of the cryptocurrency market.
Machine Learning Algorithms for Cryptocurrency Price Prediction
Machine learning algorithms offer a range of approaches to model cryptocurrency price movements. These algorithms learn patterns from historical data and use these patterns to make predictions. Simpler algorithms require less computational power but may lack the sophistication to capture complex market dynamics. More complex algorithms, while potentially more accurate, require significantly more computational resources and data.
For instance, linear regression is a simple algorithm that establishes a linear relationship between historical price data and future price predictions. While computationally inexpensive, its simplicity often leads to inaccurate predictions in the volatile cryptocurrency market where non-linear relationships are prevalent. Support Vector Machines (SVMs), on the other hand, can handle non-linear relationships more effectively by mapping data into higher-dimensional spaces.
However, SVMs can be computationally expensive for large datasets and require careful parameter tuning.
Deep Learning Algorithms for Cryptocurrency Price Prediction
Deep learning, a subset of machine learning, utilizes artificial neural networks with multiple layers to extract complex features from data. These algorithms are particularly well-suited for handling high-dimensional and complex data like that found in cryptocurrency markets. However, their increased complexity comes with a significant increase in computational cost and the need for large datasets for effective training.
Recurrent Neural Networks (RNNs), specifically Long Short-Term Memory (LSTM) networks, are commonly used due to their ability to model sequential data, which is crucial for analyzing time-series data like cryptocurrency prices. LSTMs excel at capturing long-term dependencies in price patterns, offering a potential advantage over simpler algorithms. However, training LSTMs can be computationally intensive and requires careful hyperparameter tuning to avoid overfitting.
Convolutional Neural Networks (CNNs) can also be applied, leveraging their ability to identify patterns in the price data. However, CNNs are less suited for capturing the temporal dependencies inherent in time-series data compared to RNNs.
Algorithm Performance Comparison
A direct comparison of algorithm performance is challenging due to the variability in datasets, evaluation metrics, and market conditions. However, generally, deep learning algorithms, particularly LSTMs, tend to demonstrate higher predictive accuracy than simpler machine learning algorithms like linear regression. This increased accuracy comes at the cost of significantly higher computational requirements. For example, training a complex LSTM model on a large cryptocurrency dataset might require powerful GPUs and considerable time, while a linear regression model can be trained on a standard CPU in a fraction of the time.
The unpredictable nature of cryptocurrency prices, driven by factors like news events, regulatory changes, and social media sentiment, poses a significant challenge for all algorithms. Even the most sophisticated deep learning models struggle to accurately capture these unpredictable events, leading to inaccuracies in their predictions. The inherent volatility and noise in the data make it difficult for any algorithm to consistently outperform simple baselines.
Limitations in Capturing Unpredictable Nature
All algorithms, regardless of their complexity, struggle to account for unforeseen events that significantly impact cryptocurrency prices. These “black swan” events, such as unexpected regulatory announcements or major security breaches, are inherently unpredictable and can render even the most sophisticated models ineffective. Furthermore, the market’s susceptibility to manipulation and speculative trading further complicates accurate prediction. The influence of social media sentiment and news cycles, which can rapidly shift market sentiment, introduces another layer of unpredictability that is difficult for any algorithm to consistently model.
Factors Beyond Technical Analysis: Can AI Accurately Predict Cryptocurrency Price Fluctuations?
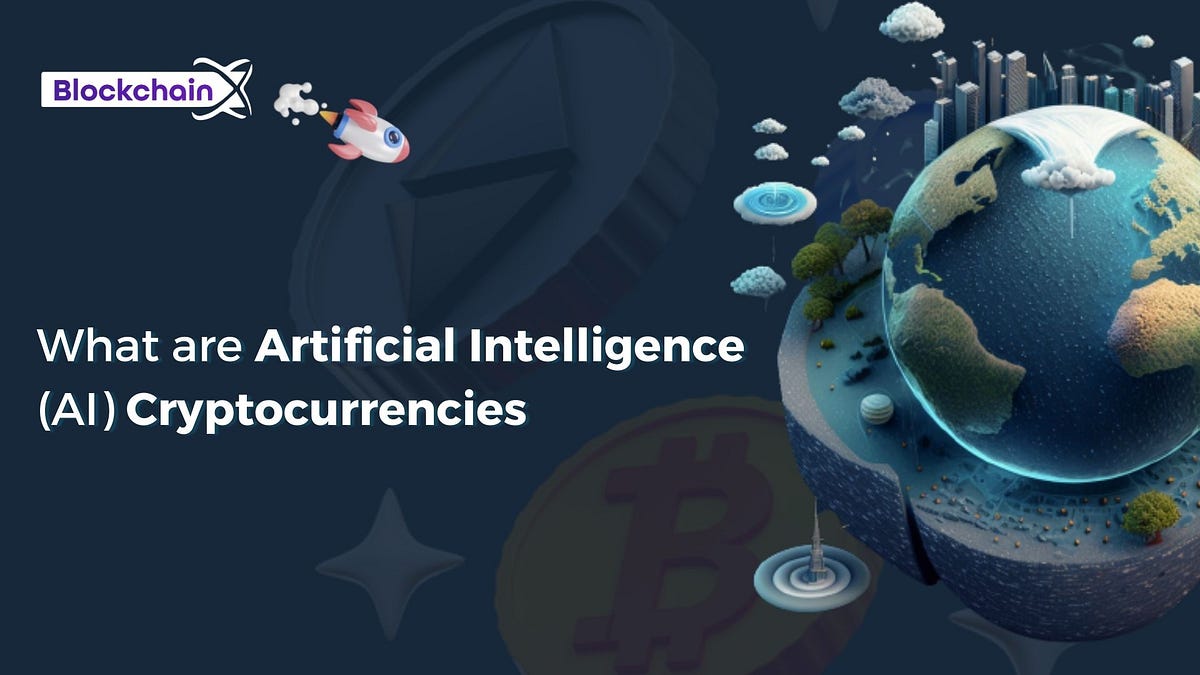
Predicting cryptocurrency prices accurately is a complex undertaking, even with sophisticated AI models. While technical analysis, using historical price and volume data, forms a crucial component of many prediction strategies, ignoring the significant influence of external factors leads to incomplete and potentially inaccurate forecasts. These external factors, often unpredictable and inherently human-driven, can dramatically override even the most refined AI-based predictions.Regulatory Changes and Their Impact on Cryptocurrency PricesRegulatory changes significantly impact cryptocurrency markets.
Governments worldwide are still developing frameworks for regulating cryptocurrencies, and these shifts in policy can cause substantial volatility. For instance, a sudden ban on cryptocurrency trading in a major market, or conversely, the introduction of favorable tax policies, can trigger massive price swings. AI models, while capable of processing vast amounts of data, struggle to accurately predict the timing and nature of these regulatory shifts, as they are often based on political considerations and public opinion rather than readily quantifiable data patterns.
The inherent unpredictability of regulatory decisions makes incorporating them reliably into AI prediction models a significant challenge.Macroeconomic Factors and Their Influence on Cryptocurrency MarketsMacroeconomic factors, such as inflation rates, interest rates, and overall economic growth, exert considerable influence on cryptocurrency markets. Cryptocurrencies, often considered an alternative investment asset, are frequently affected by shifts in investor sentiment driven by macroeconomic conditions.
For example, during periods of high inflation, investors might seek refuge in assets like cryptocurrencies, driving up their prices. Conversely, rising interest rates can make holding cryptocurrencies less attractive compared to interest-bearing accounts, leading to price declines. AI models can incorporate macroeconomic data into their predictions, but accurately forecasting these macroeconomic indicators themselves is a separate and equally complex challenge.
The intricate interplay between macroeconomic factors and cryptocurrency markets often defies simple predictive modeling. For instance, the unexpected surge in inflation in 2021-2022 had a far-reaching impact on cryptocurrency valuations, demonstrating the limitations of purely data-driven AI predictions.Psychological Factors Shaping Cryptocurrency Price FluctuationsHuman psychology plays a substantial role in shaping cryptocurrency price fluctuations. Fear, greed, and herd behavior drive significant market movements.
The “fear of missing out” (FOMO) can lead to rapid price increases as investors rush to buy, while fear of losses can trigger sell-offs, resulting in price drops. These emotional responses are difficult, if not impossible, to accurately model using AI. While AI can identify patterns in trading activity indicative of herd behavior, it cannot anticipate the specific triggers for these psychological shifts or their intensity.
The inherent irrationality of human behavior poses a fundamental limitation to accurate price prediction through AI alone. The rapid rise and fall of meme coins, driven largely by social media hype and emotional responses, serve as prime examples of this phenomenon.Examples of Non-Technical Factors Overriding AI PredictionsThe 2022 cryptocurrency market crash, partly driven by macroeconomic factors such as rising interest rates and increased regulatory scrutiny, is a clear example of non-technical factors overriding AI predictions.
Many AI models predicted continued growth based on technical analysis alone, failing to account for the significant influence of external forces. Similarly, the sudden collapse of FTX, a major cryptocurrency exchange, caused a significant market downturn that AI models struggled to anticipate. The event was triggered by a combination of regulatory concerns and internal mismanagement, factors largely outside the scope of typical AI predictive models.Illustration of Macroeconomic Events Affecting Cryptocurrency PricesImagine a scenario where a central bank unexpectedly raises interest rates to combat inflation.
This action makes traditional investments like bonds more attractive, diverting capital away from riskier assets such as cryptocurrencies. Simultaneously, the increased interest rates could also lead to a tightening of credit markets, making it more difficult for businesses operating in the cryptocurrency space to secure funding. These combined effects would likely create downward pressure on cryptocurrency prices, irrespective of any positive technical indicators detected by an AI model.
The initial increase in interest rates represents a macro-level event, while the resulting reduction in cryptocurrency funding and decreased investor interest demonstrate the ripple effect on the market. This cascade of effects, often nonlinear and complex, underscores the limitations of solely relying on AI for accurate price predictions.
Ethical Considerations and Responsible Use
The application of AI to predict cryptocurrency price fluctuations raises significant ethical concerns. The potential for misuse, coupled with the inherent volatility of the cryptocurrency market, necessitates a careful consideration of responsible development and deployment practices. Transparency and accountability are paramount to mitigating risks and ensuring fair market practices.The potential for AI-driven cryptocurrency price prediction to be used for market manipulation is substantial.
Sophisticated algorithms could be employed to artificially inflate or deflate prices, enabling coordinated trading strategies that benefit a select few at the expense of others. This could destabilize the market, leading to significant financial losses for unsuspecting investors. For instance, a powerful algorithm could detect a subtle shift in market sentiment before it’s reflected in price, allowing for the preemptive purchase or sale of assets, creating artificial price movements that could trigger a cascade effect.
Market Manipulation Risks
AI-powered prediction models, if misused, could facilitate insider trading and pump-and-dump schemes. The speed and scale at which AI can process information and execute trades significantly amplify the potential for manipulative behavior. Furthermore, the lack of transparency surrounding some AI algorithms makes it difficult to detect and prevent such activities. Consider a scenario where a group of traders uses a proprietary AI model to identify undervalued assets, purchasing them en masse before the market corrects, resulting in artificially inflated prices that then crash as they sell off their holdings.
This represents a clear ethical breach and market manipulation.
Transparency and Accountability in AI Development
Transparency and accountability are crucial to building trust and ensuring responsible use of AI in cryptocurrency trading. Open-source algorithms, publicly available datasets, and clear documentation of model development and deployment processes are vital. Regular audits and independent verification of AI models can help identify potential biases and vulnerabilities that could be exploited for manipulative purposes. Without these measures, the risk of unethical use remains high, eroding confidence in the entire cryptocurrency ecosystem.
For example, a model’s decision-making process should be understandable and auditable, preventing the use of “black box” algorithms that obfuscate their workings and hinder the detection of potential bias or manipulation.
Risks of Over-Reliance on AI Predictions
Over-reliance on AI predictions in cryptocurrency trading carries significant risks. AI models, no matter how sophisticated, are not infallible. They are trained on historical data and may not accurately predict future price movements, especially in a market as volatile as cryptocurrencies. This can lead to poor investment decisions, substantial financial losses, and a false sense of security.
Investors should remember that AI is a tool, not a crystal ball. Blind faith in AI predictions can be financially devastating. A real-world example could be an investor heavily leveraging their investments based on a prediction that proves inaccurate, leading to significant losses when the market moves in an unexpected direction.
Best Practices for Responsible AI Use in Cryptocurrency Markets
Responsible use of AI in cryptocurrency markets requires a multi-faceted approach. This includes rigorous testing and validation of AI models, ongoing monitoring for biases and vulnerabilities, and the establishment of clear ethical guidelines for developers and users. Education and awareness are also crucial, helping investors understand the limitations of AI and avoid over-reliance on its predictions. Furthermore, regulatory frameworks are necessary to ensure accountability and prevent market manipulation.
Robust data governance is also key; this involves ensuring data quality, accuracy, and provenance, preventing the use of manipulated or incomplete datasets that could lead to biased or inaccurate predictions.
Ethical Guidelines for Developers and Users
The responsible development and use of AI-powered cryptocurrency prediction tools require adherence to a strict set of ethical guidelines. These guidelines should emphasize transparency, accountability, and a commitment to fair market practices. Below is a list of key considerations:
- Transparency: Openly disclose the methodology, data sources, and limitations of AI prediction models.
- Accountability: Establish clear lines of responsibility for the development, deployment, and use of AI models.
- Data Integrity: Ensure the use of accurate, unbiased, and reliable data for training and validation of AI models.
- Fairness: Avoid the development or use of AI models that could be used to unfairly advantage certain individuals or groups.
- Security: Implement robust security measures to protect AI models and prevent unauthorized access or manipulation.
- User Education: Provide clear and accessible information to users about the limitations of AI predictions.
- Compliance: Adhere to all applicable laws and regulations related to cryptocurrency trading and AI.
Ultimate Conclusion
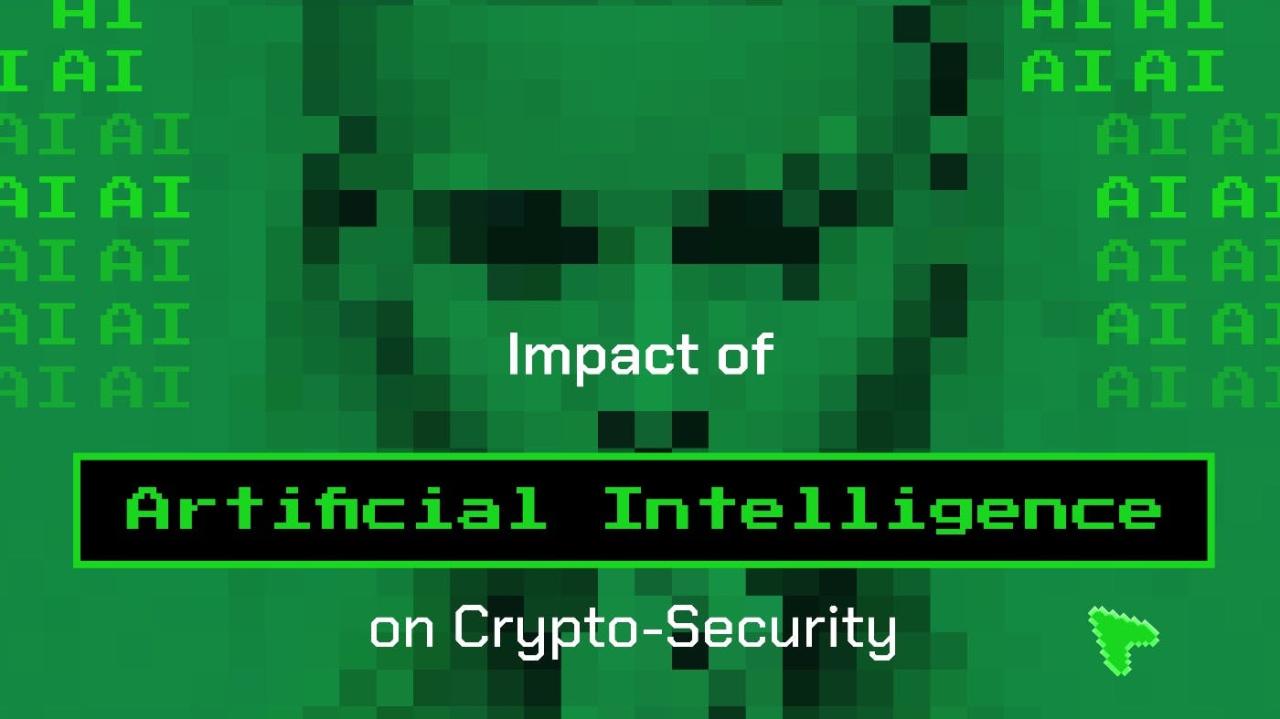
Ultimately, while AI can offer valuable insights into cryptocurrency market trends, accurately predicting price fluctuations remains elusive. The inherent volatility, influenced by a complex interplay of technical and non-technical factors, renders perfect prediction nearly impossible. The effectiveness of AI in this domain depends heavily on data quality, algorithm selection, and a nuanced understanding of the market’s unpredictable nature.
Responsible development and deployment of AI-driven prediction tools, coupled with a healthy dose of skepticism, are crucial to mitigating potential risks and ethical concerns.