Can AI systems be used to steal sensitive information from individuals? The answer, unfortunately, is a resounding yes. Artificial intelligence, while offering incredible benefits, presents a significant threat to personal data security. From sophisticated phishing attacks leveraging deepfakes to AI-powered malware capable of evading detection, the potential for misuse is alarming. This exploration delves into the various methods employed, the vulnerabilities exploited, and the crucial steps individuals and organizations can take to mitigate these risks.
The rapid advancement of AI has created new avenues for cybercriminals to exploit. AI’s ability to automate tasks, analyze data at scale, and learn from past behavior makes it a powerful tool in the hands of malicious actors. This article will examine how AI is used in data breaches, the role it plays in malware development and deployment, and the inherent vulnerabilities within AI systems themselves.
We will also explore the ethical implications and discuss practical mitigation strategies to safeguard sensitive information in this increasingly digital world.
Methods of Data Breaches via AI
Artificial intelligence (AI) is rapidly evolving, and its capabilities are being exploited for malicious purposes, including the theft of sensitive information. AI’s ability to automate tasks, analyze vast datasets, and learn from patterns makes it a powerful tool for cybercriminals. This section details common methods used to breach data security using AI.
AI-Powered Phishing and Social Engineering Attacks
AI significantly enhances the effectiveness of phishing and social engineering attacks. These attacks rely on manipulating individuals into revealing sensitive information, such as login credentials or financial details. AI’s role is to personalize and automate the process, increasing the likelihood of success.
Method | Target | Vulnerability Exploited | AI Role |
---|---|---|---|
Spear Phishing | Specific individuals (e.g., executives, employees with access to sensitive data) | Trust in legitimate-seeming communication; lack of security awareness | Personalizes phishing emails using data analysis to craft convincing messages tailored to the target’s profile and interests; analyzes responses to optimize future campaigns. |
Whaling | High-profile individuals (e.g., CEOs, CFOs) | High value of their information; assumption of legitimacy from seemingly authoritative sources | Analyzes public information to create highly targeted and credible phishing emails or phone calls, potentially including deepfakes. |
Social Engineering via Chatbots | Individuals susceptible to social manipulation | Trust in interactive communication; lack of awareness of chatbot interaction | Creates sophisticated chatbots capable of engaging in realistic conversations to build trust and extract information. |
Fake Website Generation | Users seeking to access legitimate services | Trust in visually similar websites; lack of URL verification | Generates realistic-looking fake websites that mimic legitimate platforms, tricking users into entering their credentials. |
Deepfakes and AI-Powered Voice Cloning in Identity Theft, Can AI systems be used to steal sensitive information from individuals?
Deepfakes, AI-generated videos or audio recordings that convincingly portray someone else, and AI-powered voice cloning are potent tools for identity theft. These technologies allow criminals to impersonate individuals convincingly, bypassing traditional security measures.For instance, a deepfake video of a company CEO could be used to authorize a fraudulent transaction or reveal sensitive information to a seemingly trusted source. Similarly, AI-powered voice cloning could be used to impersonate a bank representative over the phone, convincing a victim to disclose their account details.
These attacks exploit the trust placed in visual and auditory cues. A real-world example would be a deepfake video of a bank manager instructing a customer to transfer funds, a scenario made more believable with the cloned voice of the manager.
AI-Automated Credential Stuffing and Brute-Force Attacks
AI significantly accelerates credential stuffing and brute-force attacks, both of which aim to gain unauthorized access to accounts by trying numerous username and password combinations.AI can automate this process by:
1. Gathering Credentials
AI algorithms can scrape credentials from data breaches available on the dark web.
2. Testing Credentials
The AI then systematically tests these credentials against various online services.
3. Adapting Strategies
AI learns from successful and unsuccessful attempts, adjusting its strategies to improve efficiency and bypass security measures such as rate limiting or CAPTCHAs.
4. Scaling Attacks
AI can easily scale attacks across multiple accounts and services simultaneously, significantly increasing the chances of success.This automated process makes these attacks far more effective and efficient than manual attempts. For example, an AI could try thousands of combinations per second, exponentially increasing the likelihood of finding a working credential.
AI’s Role in Malware Development and Deployment: Can AI Systems Be Used To Steal Sensitive Information From Individuals?
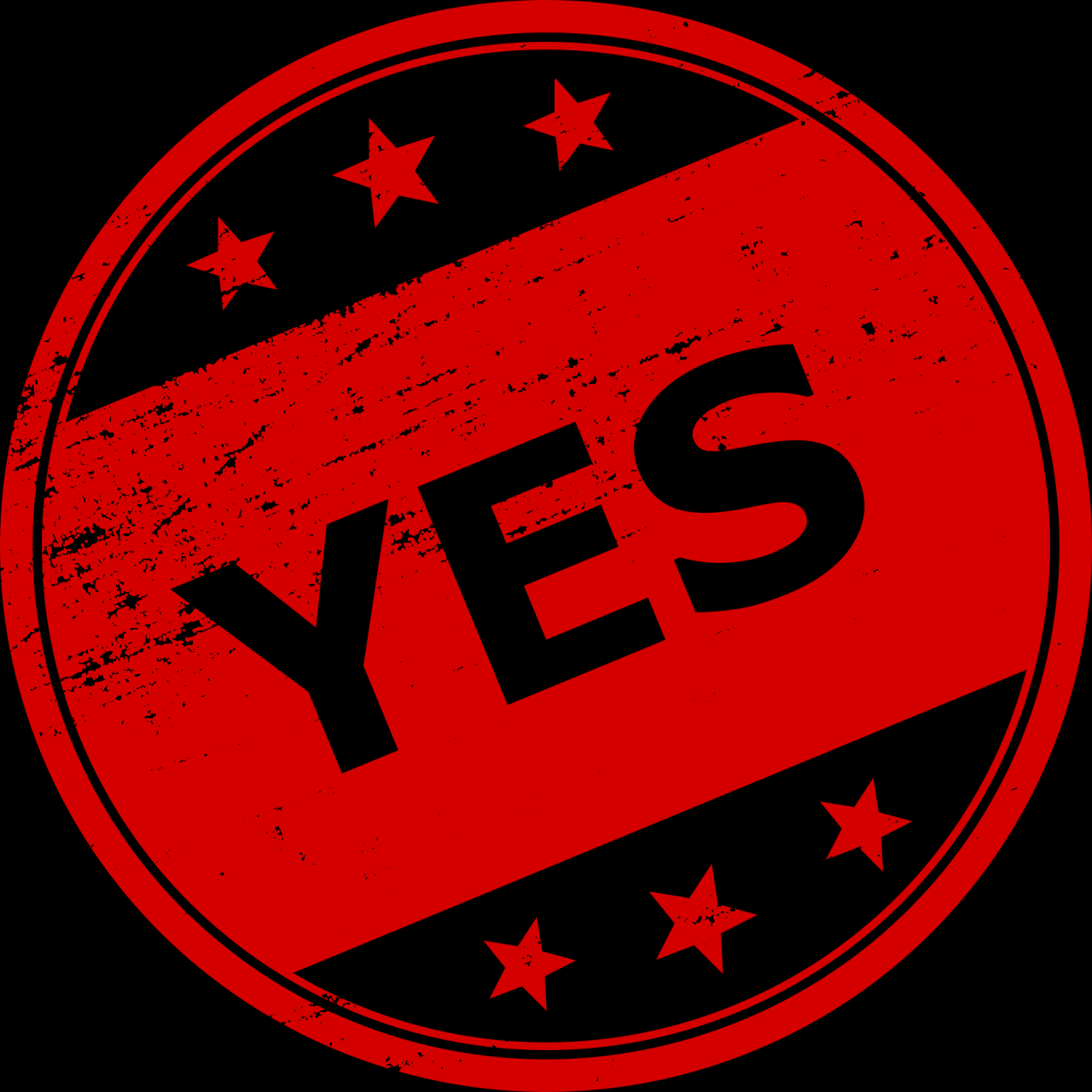
The convergence of artificial intelligence and malicious actors presents a significant threat to cybersecurity. AI’s capabilities, traditionally used for beneficial purposes, can be readily weaponized to create more sophisticated, adaptive, and evasive malware, automating its distribution, and targeting vulnerabilities with unprecedented precision. This section explores the specific ways AI is being leveraged in the development and deployment of malicious software.AI algorithms are being increasingly used to enhance the capabilities of malware in several key ways.
This leads to a new generation of threats that are significantly harder to detect and mitigate using traditional security methods.
AI-Enhanced Malware Creation
AI can significantly enhance the creation of sophisticated and evasive malware. The following points illustrate how AI algorithms are being employed:
- Automated Code Generation: AI can generate diverse variations of malware code, making it difficult for signature-based detection systems to identify all instances. This involves using Generative Adversarial Networks (GANs) to create new malware samples that resemble legitimate software, thereby bypassing traditional security measures.
- Polymorphic Malware Generation: AI can create polymorphic malware that changes its code structure frequently, making it exceptionally difficult to detect and analyze. This constant evolution allows the malware to evade signature-based detection systems and sandbox analysis.
- Self-Learning Malware: AI algorithms enable the creation of malware that can learn and adapt to its environment, becoming more effective over time at evading detection and achieving its malicious goals. This adaptive nature makes it a persistent and evolving threat.
- Exploit Generation: AI can be used to automatically identify and exploit software vulnerabilities, leading to the creation of highly targeted and effective attacks. This automated process reduces the time and expertise needed to develop effective exploits.
AI-Driven Malware Distribution Through Targeted Phishing
AI is rapidly transforming phishing campaigns, making them more effective and difficult to identify. The automation capabilities of AI allow for the creation of highly personalized and targeted attacks at scale. The following flowchart illustrates this process:
+-----------------+ | AI-Powered | | Phishing Engine| +--------+--------+ | V +-----------------+ | Data Collection | | (Victim Profiles)| +--------+--------+ | V +-----------------+ | Campaign | | Personalization| +--------+--------+ | V +-----------------+ | Email/SMS | | Generation | +--------+--------+ | V +-----------------+ | Targeted | | Delivery | +--------+--------+ | V +-----------------+ | Infection | | & Data Exfiltration| +-----------------+
AI-Powered Network Vulnerability Analysis and Exploitation
AI can be used to analyze vast amounts of network traffic data to identify vulnerabilities and subsequently exploit them.
This allows for highly targeted attacks against specific systems or networks.
- Zero-Day Exploit Discovery: AI can analyze network traffic patterns to identify previously unknown vulnerabilities (zero-day exploits) that haven’t been patched. This allows attackers to exploit these weaknesses before security vendors are aware of them.
- Network Intrusion Detection System (NIDS) Evasion: AI can be used to create malware that evades detection by NIDS by adapting its behavior and communication patterns in response to security measures. This makes it harder for security systems to identify and block malicious activity.
- Vulnerability Prioritization: AI can prioritize vulnerabilities based on their potential impact and exploitability, allowing attackers to focus their efforts on the most critical targets. This targeted approach increases the likelihood of a successful attack.
Examples of vulnerabilities that can be exploited include outdated software versions, misconfigurations of network devices, and weaknesses in web applications. The use of AI significantly accelerates the process of identifying and exploiting these vulnerabilities.
Vulnerabilities in AI Systems Themselves
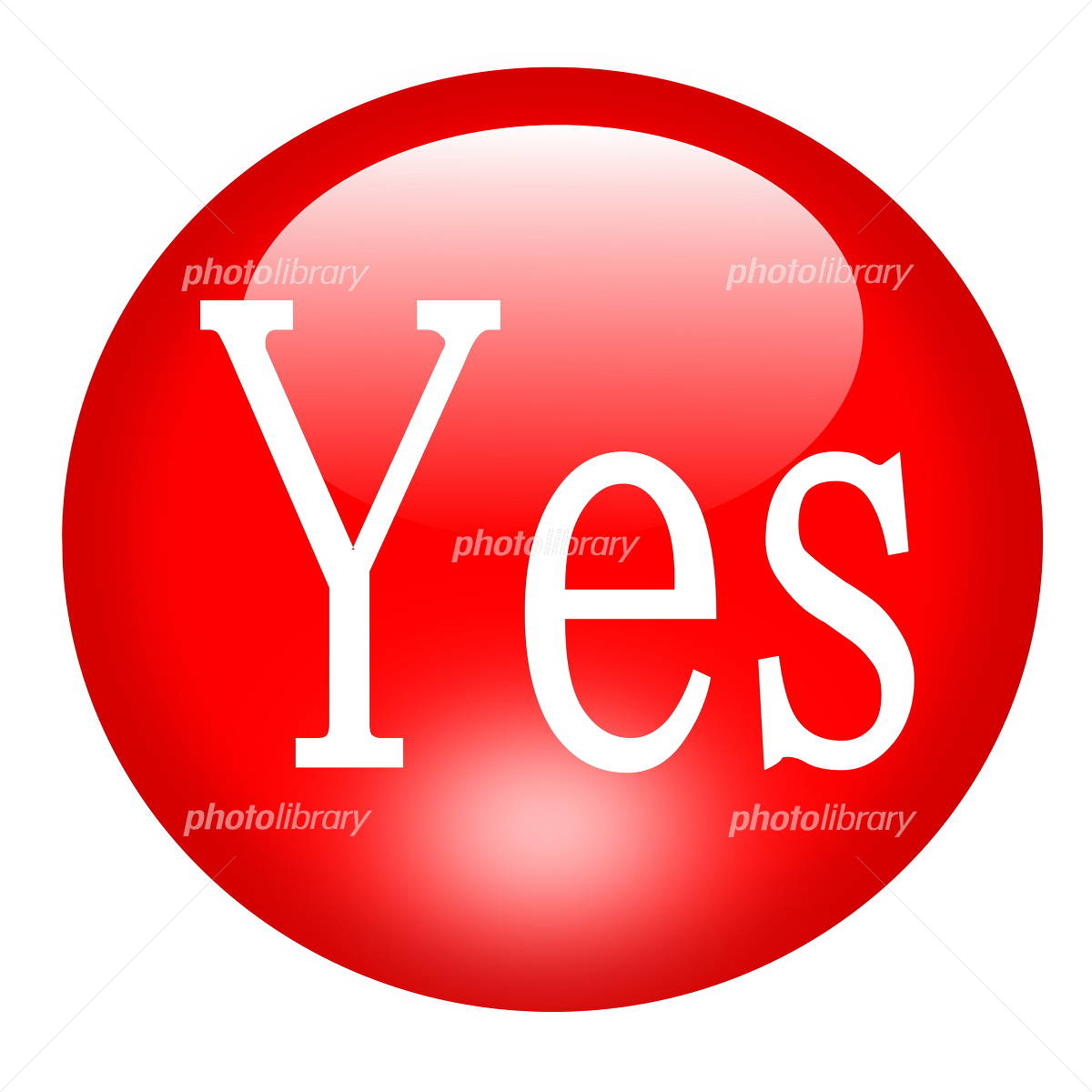
AI systems, while powerful, are not immune to exploitation. Malicious actors can leverage inherent weaknesses in their design, training data, and deployment to gain unauthorized access to sensitive information. Understanding these vulnerabilities is crucial for mitigating the risks associated with AI-driven data breaches.
AI systems are susceptible to a range of attacks that exploit their vulnerabilities, leading to sensitive data theft. These vulnerabilities can be categorized in several ways, each presenting unique challenges for security professionals.
Types of AI System Vulnerabilities
The following table compares different types of vulnerabilities in AI systems that can be exploited for data theft.
Vulnerability Type | Description | Example | Consequences |
---|---|---|---|
Data Poisoning | Introducing malicious data into the training dataset to manipulate the AI’s behavior. | Adding false positive entries into a fraud detection system to allow fraudulent transactions. | Compromised model accuracy, leading to incorrect decisions and potential data leaks due to flawed logic. |
Model Extraction | Inferring the internal structure and parameters of a machine learning model by querying it repeatedly. | An attacker might use a series of carefully crafted inputs to reconstruct a sensitive model used for facial recognition. | Exposure of intellectual property, leading to the replication of sensitive algorithms and models. |
Adversarial Attacks | Introducing subtle, almost imperceptible changes to input data to cause the AI to make incorrect predictions. | Manipulating an image slightly to cause an autonomous vehicle’s object recognition system to misclassify a stop sign. In a data context, this could lead to incorrect classifications resulting in data leakage or unauthorized access. | Data misclassification, leading to inaccurate analysis and potential security breaches. |
Backdoors | Introducing intentional vulnerabilities during the development process that allow unauthorized access. | A hidden command within a voice assistant that grants access to personal data upon activation of a specific phrase. | Direct access to sensitive data and control over the AI system. |
Software Vulnerabilities | Exploiting flaws in the software infrastructure supporting the AI system. | A SQL injection attack against a database storing AI model training data. | Direct access to the underlying data used to train and operate the AI system. |
Risks of Inadequate Security Measures in AI-Driven Data Analysis
Using AI for data analysis without robust security measures significantly increases the risk of data breaches. The sensitive nature of the data often analyzed (e.g., personal health information, financial records, intellectual property) makes these systems prime targets for malicious actors. A breach could lead to significant financial losses, reputational damage, legal repercussions (like GDPR fines), and erosion of customer trust.
For example, a healthcare provider using AI to analyze patient data without adequate security could experience a breach exposing protected health information, leading to substantial fines and loss of patient confidence.
Challenges in Detecting and Preventing AI-Powered Attacks
Detecting and preventing AI-powered attacks presents significant challenges. The sophisticated nature of these attacks, combined with the ever-evolving landscape of AI techniques, makes traditional security measures insufficient. Moreover, the lack of standardized security protocols specifically designed for AI systems exacerbates the problem.
The following security practices are recommended to mitigate these risks:
Robust security practices are essential to mitigate the risks associated with AI-powered attacks. A multi-layered approach is necessary, combining preventative measures with robust detection and response capabilities.
- Data Sanitization and Anonymization: Removing or altering personally identifiable information from datasets used for AI training and analysis.
- Model Security: Implementing techniques to protect AI models from extraction and adversarial attacks, including model obfuscation and differential privacy.
- Secure Development Lifecycle: Integrating security considerations throughout the entire lifecycle of AI system development, from design to deployment.
- Regular Security Audits and Penetration Testing: Proactively identifying and addressing vulnerabilities in AI systems and their supporting infrastructure.
- Threat Intelligence and Monitoring: Staying informed about emerging threats and monitoring AI systems for suspicious activity.
- Incident Response Plan: Developing a comprehensive plan to respond to and mitigate the impact of AI-powered data breaches.
- Employee Training: Educating employees about the risks of AI-powered attacks and best practices for data security.
Privacy Implications and Mitigation Strategies
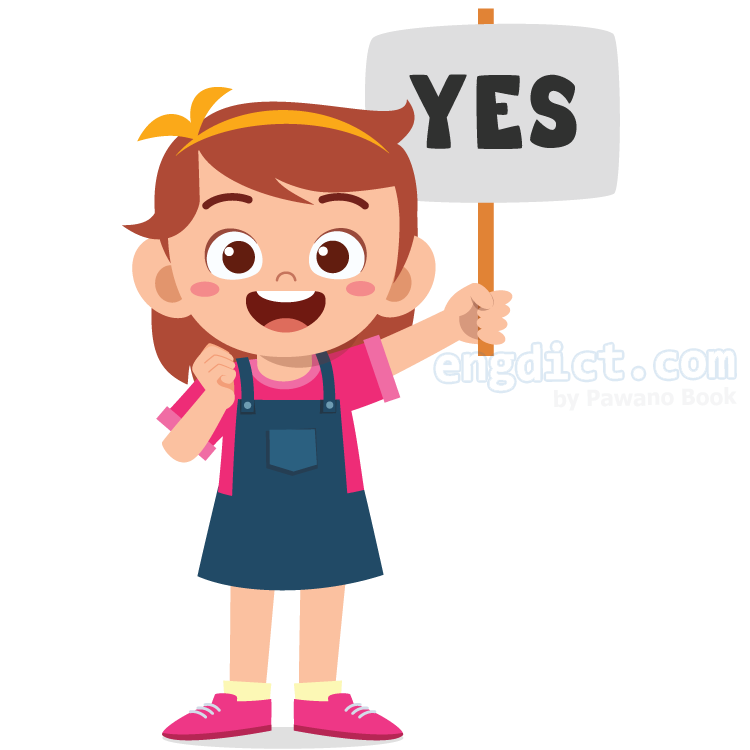
The increasing sophistication of AI systems presents significant challenges to individual privacy. AI’s ability to process vast amounts of data, identify patterns, and make predictions opens avenues for privacy violations that were previously unimaginable. Understanding these implications and implementing effective mitigation strategies is crucial for safeguarding personal information in the age of artificial intelligence.
Real-World Scenarios of AI-Powered Privacy Compromise
Several real-world scenarios illustrate the potential for AI to compromise individual privacy. For instance, facial recognition technology, while offering benefits in security and identification, has been used to track individuals without their knowledge or consent, raising concerns about surveillance and potential misuse. Deepfake technology, capable of generating realistic but fabricated videos and audio recordings, poses a serious threat to reputation and can be used to spread misinformation or impersonate individuals for malicious purposes.
Furthermore, AI-powered profiling based on online activity and personal data can lead to discriminatory practices in areas like loan applications, insurance pricing, and even employment opportunities. These scenarios highlight the need for robust regulations and ethical considerations in the development and deployment of AI systems.
Best Practices for Protecting Against AI-Powered Attacks
Protecting oneself from AI-powered attacks requires a multi-faceted approach encompassing both technological and behavioral safeguards.
- Employ strong passwords and multi-factor authentication: Robust passwords and multi-factor authentication significantly increase the difficulty for attackers to gain unauthorized access to online accounts, reducing the risk of data breaches exploited by AI systems.
- Be cautious about sharing personal information online: Limit the amount of personal data shared on social media and other online platforms. Avoid posting sensitive information such as addresses, financial details, or unique identifiers.
- Use privacy-enhancing technologies: Utilize tools such as VPNs (Virtual Private Networks) and privacy-focused browsers to encrypt online activity and mask IP addresses, making it more challenging for AI-powered surveillance to track online behavior.
- Regularly update software and security protocols: Keeping software and operating systems updated ensures that security vulnerabilities are patched, reducing the likelihood of AI-powered malware exploiting weaknesses in the system.
- Be aware of phishing and social engineering attempts: AI can be used to create sophisticated phishing emails and other social engineering attacks designed to trick individuals into revealing sensitive information. Maintaining awareness and skepticism towards unsolicited communications is crucial.
- Monitor online accounts for suspicious activity: Regularly check account statements and online activity for any unusual or unauthorized transactions or access attempts. Report suspicious activity immediately.
Methods for Detecting and Responding to AI-Powered Data Breaches
Detecting and responding to AI-powered data breaches requires a comprehensive strategy that combines technical solutions and human expertise.
Method | Description | Advantages | Disadvantages |
---|---|---|---|
Anomaly Detection | Utilizes AI algorithms to identify unusual patterns in data traffic and system behavior that may indicate a breach. | Proactive; can detect breaches early. | Requires significant computational resources; may generate false positives. |
Intrusion Detection Systems (IDS) | Monitors network traffic for malicious activity, including AI-powered attacks. | Established technology; widely available. | Can be bypassed by sophisticated attacks; requires regular updates. |
Security Information and Event Management (SIEM) | Collects and analyzes security logs from various sources to identify security threats, including those involving AI. | Provides comprehensive security monitoring; facilitates incident response. | Complex to implement and manage; requires skilled personnel. |
Threat Intelligence Platforms | Gather and analyze threat information from various sources to identify emerging threats and vulnerabilities, including those related to AI. | Provides proactive threat awareness; helps prioritize security efforts. | Relies on external data sources; may not capture all threats. |
The Ethical Considerations of AI and Data Security
The increasing sophistication of AI systems presents profound ethical challenges concerning data security and individual privacy. The capacity of AI to process vast amounts of data, identify patterns, and make predictions raises concerns about potential misuse, leading to significant societal consequences. Balancing the benefits of AI-driven innovation with the imperative to protect fundamental rights requires careful consideration of ethical principles and the implementation of robust safeguards.The ethical implications of using AI for surveillance and data collection are far-reaching.
AI-powered surveillance systems, for example, can track individuals’ movements, monitor their communications, and analyze their behavior in ways that were previously unimaginable. This raises concerns about potential abuses of power, mass surveillance, and the erosion of civil liberties. The potential for biased algorithms to disproportionately target specific demographics further exacerbates these concerns, potentially leading to social inequalities and discrimination.
The lack of transparency in many AI systems also makes it difficult to understand how decisions are made, raising concerns about accountability and due process.
Ethical Implications of AI-Powered Surveillance
The use of AI in surveillance raises significant ethical questions. The potential for misuse, such as profiling individuals based on their race, religion, or political beliefs, is a serious concern. Furthermore, the constant monitoring of individuals can create a chilling effect on free speech and association. The lack of transparency in how AI surveillance systems operate makes it difficult to hold those responsible for their deployment accountable.
For example, facial recognition technology, while offering potential benefits in security, can be misused for discriminatory purposes or used without proper oversight, leading to misidentification and wrongful accusations. This underscores the need for strict regulations and ethical guidelines governing the use of AI in surveillance.
Incorporating Security Measures in AI Development
The development and deployment of AI systems must incorporate robust security measures from the outset. This requires a “security-by-design” approach, integrating security considerations into every stage of the AI lifecycle, from data collection and model training to deployment and maintenance. Specific examples include: implementing differential privacy techniques to protect individual data during training, employing robust encryption methods to safeguard sensitive information, and regularly auditing AI systems for vulnerabilities.
Furthermore, rigorous testing and validation are crucial to ensure the reliability and security of AI systems. For instance, before deploying a facial recognition system for law enforcement, thorough testing should be conducted to assess its accuracy and potential for bias, and rigorous protocols should be established for data handling and access control.
Hypothetical AI-Powered Data Breach Scenario and Ramifications
Consider a scenario where a large financial institution uses an AI-powered fraud detection system. This system, however, contains a vulnerability that allows a malicious actor to access and manipulate the personal data of millions of customers. The attacker could use this data for identity theft, financial fraud, or blackmail. The legal ramifications would be significant, potentially involving hefty fines, lawsuits, and criminal charges.
Ethically, the company would face severe reputational damage and a loss of public trust. The breach would also raise questions about the company’s responsibility for protecting customer data and the adequacy of its security measures. Such an event highlights the critical need for proactive security measures and ethical considerations throughout the AI development lifecycle to prevent such catastrophic failures.
Final Conclusion
The potential for AI to be weaponized against individuals is a stark reality. While AI offers transformative possibilities, its capacity for malicious use demands immediate attention. Understanding the methods used, the vulnerabilities exploited, and the proactive measures needed to protect sensitive information is paramount. By fostering a collaborative approach between technology developers, security experts, and individuals, we can strive to harness the benefits of AI while mitigating its inherent risks to privacy and security.
The future of data security hinges on our collective ability to adapt and innovate in the face of this evolving threat landscape.