Can AI truly predict the next Bitcoin bull run? This question lies at the heart of a fascinating debate, merging the volatile world of cryptocurrency with the rapidly advancing field of artificial intelligence. Bitcoin’s history is punctuated by dramatic price swings, fueled by a complex interplay of market sentiment, technological advancements, and global events. Can sophisticated algorithms, trained on vast datasets, truly decipher this chaotic landscape and accurately forecast future price movements?
This exploration delves into the capabilities and limitations of AI in predicting Bitcoin’s notoriously unpredictable trajectory.
We’ll examine various AI models, their data sources, and their predictive accuracy, comparing them to traditional market analysis techniques. The discussion will also highlight the inherent limitations of any predictive model, particularly in a market as volatile as Bitcoin’s, influenced by unforeseen events and macroeconomic factors beyond the reach of even the most advanced algorithms. Ultimately, we aim to provide a balanced perspective on the potential and pitfalls of using AI to anticipate the next Bitcoin bull run.
Bitcoin’s Price History and Market Sentiment
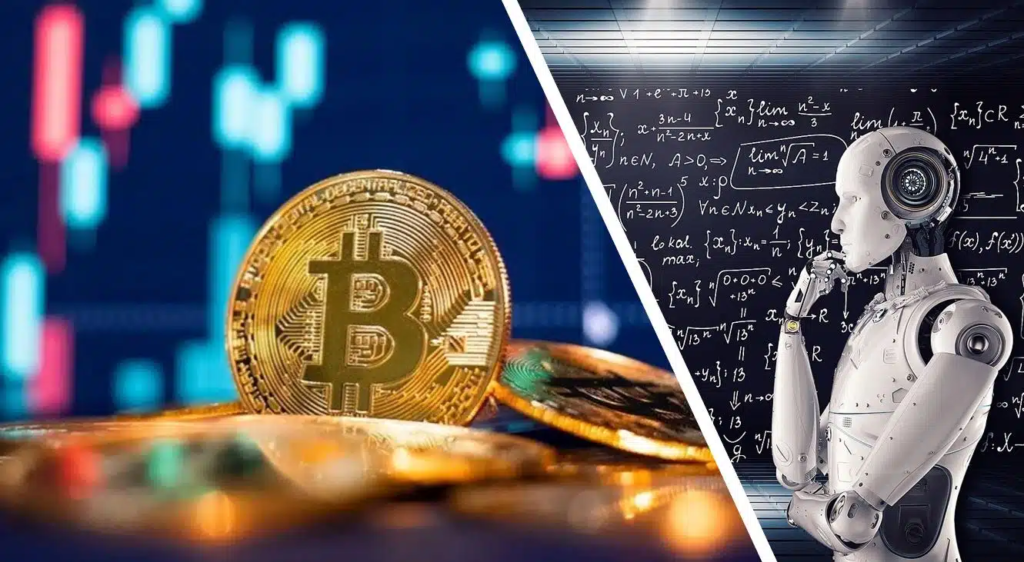
Bitcoin’s price has been characterized by extreme volatility since its inception, exhibiting periods of dramatic growth (bull markets) interspersed with significant declines (bear markets). Understanding this historical price action, along with the prevailing market sentiment during these periods, is crucial for any attempt to predict future price movements. Analyzing these patterns, alongside other market indicators, can offer valuable insights, though it’s important to remember that predicting future price movements with certainty remains impossible.
Bitcoin Price Fluctuations and Market Sentiment
The following table summarizes key periods in Bitcoin’s price history, highlighting major bull and bear markets, associated market sentiment, and significant events. Note that market sentiment is a subjective assessment based on prevailing narratives and observable investor behavior.
Date | Price (USD) (Approximate) | Market Sentiment | Significant Events |
---|---|---|---|
2010-2013 | <$1 - $1,000 | Mostly Neutral, then Bullish | Early adoption, limited awareness, Mt. Gox exchange launch. |
2013-2015 | $1,000 – <$200 | Bullish, then Bearish | Mt. Gox hack, regulatory uncertainty, price bubble burst. |
2016-2017 | <$1,000 - ~$20,000 | Bearish, then Extremely Bullish | SegWit implementation, increasing institutional interest, growing mainstream media coverage. |
2017-2018 | ~$20,000 – ~$3,000 | Extremely Bullish, then Extremely Bearish | Regulatory crackdowns in various countries, market manipulation concerns. |
2019-2021 | ~$3,000 – ~$65,000 | Bearish, then Bullish | Halving event, DeFi boom, institutional adoption accelerates. |
2021-Present | ~$65,000 – Variable | Bullish, then Bearish, then fluctuating | Increased regulatory scrutiny, macroeconomic factors (inflation, interest rates), ongoing institutional investment. |
Influence of Social Media and News Sentiment
Social media platforms and news outlets significantly influence Bitcoin’s price through the dissemination of information and the shaping of public perception. Positive news, such as institutional adoption or technological advancements, often fuels bullish sentiment and drives price increases. Conversely, negative news, like regulatory crackdowns or security breaches, can trigger bearish sentiment and price drops. The speed and reach of social media amplify these effects, leading to rapid price swings.
For example, Elon Musk’s tweets have historically been shown to correlate with short-term price volatility.
Indicators Preceding Bitcoin Bull Runs
Several indicators have historically preceded Bitcoin bull runs. These are not guarantees of future price increases, but rather patterns observed in the past.Halving events, which reduce the rate of new Bitcoin creation, have often been followed by bull markets. This is attributed to the decreased supply leading to potential price appreciation. Regulatory changes, while sometimes negative in the short term, can create clarity and pave the way for increased institutional adoption and subsequent price growth.
Significant institutional adoption, marked by large-scale investments from corporations and financial institutions, generally signals increased confidence and often precedes bull markets. These factors, along with overall macroeconomic conditions and technological advancements within the cryptocurrency space, contribute to the complex interplay that drives Bitcoin’s price.
AI’s Role in Predicting Market Trends: Can AI Truly Predict The Next Bitcoin Bull Run?
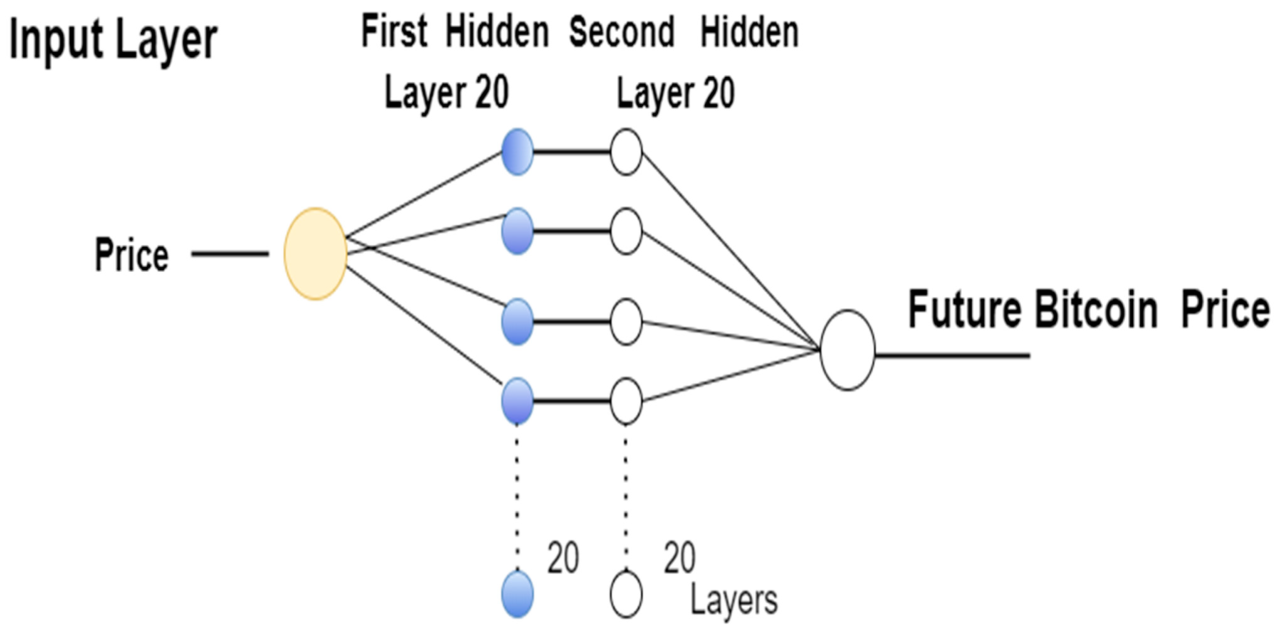
Artificial intelligence (AI) is increasingly being employed in financial markets to analyze vast datasets and identify patterns that might predict future price movements. While its application holds promise, particularly in the volatile cryptocurrency market, its effectiveness in accurately predicting Bitcoin bull runs remains a complex and debated topic. The inherent limitations of AI, coupled with the unpredictable nature of Bitcoin, necessitate a cautious approach to interpreting AI-driven predictions.AI algorithms used in financial market prediction leverage various techniques, primarily focusing on machine learning and deep learning methodologies.
These methods excel at identifying complex relationships within large datasets that might be missed by traditional methods.
Machine Learning and Deep Learning in Bitcoin Price Prediction, Can AI truly predict the next Bitcoin bull run?
Machine learning algorithms, such as support vector machines (SVMs), random forests, and gradient boosting machines, analyze historical Bitcoin price data, trading volume, and other relevant market indicators to build predictive models. These models learn from past data to identify patterns and predict future price movements. Deep learning, a subset of machine learning, uses artificial neural networks with multiple layers to analyze data and identify even more complex patterns.
Recurrent neural networks (RNNs), for example, are particularly well-suited for analyzing time-series data like Bitcoin price fluctuations. These algorithms can incorporate a wide range of factors, including social media sentiment, news articles, and regulatory developments, to enhance their predictive capabilities. For instance, a model might analyze historical price data alongside news articles about regulatory changes to predict how the price might react to future regulatory announcements.
Limitations of AI in Predicting Bitcoin’s Price
Despite the potential of AI, accurately predicting Bitcoin’s price remains extremely challenging due to the inherent volatility and unpredictable nature of the cryptocurrency market. Bitcoin’s price is susceptible to significant swings driven by factors such as regulatory changes, technological advancements, market sentiment, and large-scale investor activity. These factors often defy simple statistical modeling and can lead to unpredictable price spikes or crashes.
Furthermore, the relatively short history of Bitcoin, compared to traditional financial assets, limits the amount of historical data available for training AI models. The lack of sufficient historical data can hinder the ability of AI algorithms to accurately capture the full complexity of the Bitcoin market. Finally, the presence of market manipulation and “whale” activity can significantly distort price movements, making accurate prediction even more difficult for AI models.
A sudden large sale by a major holder can trigger a cascade of sell-offs that are virtually impossible for an AI to accurately anticipate.
Comparison of AI-Driven Predictions with Traditional Analysis
AI-driven predictions offer the potential advantage of processing vast amounts of data quickly and identifying complex relationships that might be missed by human analysts. However, traditional technical and fundamental analysis still hold value. Technical analysis, which involves studying price charts and trading volume to identify patterns, provides a framework for understanding short-term price movements. Fundamental analysis focuses on evaluating the underlying value of an asset by examining factors such as its technology, adoption rate, and regulatory environment.
While AI can augment these traditional methods by providing additional insights from large datasets, it’s crucial to understand that neither AI-driven predictions nor traditional methods guarantee accurate forecasts in the highly volatile Bitcoin market. The accuracy of both approaches is often limited, and combining insights from multiple sources, including both AI and traditional methods, is often a more prudent strategy than relying solely on one method.
For example, an AI might identify a correlation between social media sentiment and price movements, but a fundamental analyst could offer insights into the long-term viability of the cryptocurrency itself, providing a more holistic view.
Data Sources and Predictive Models
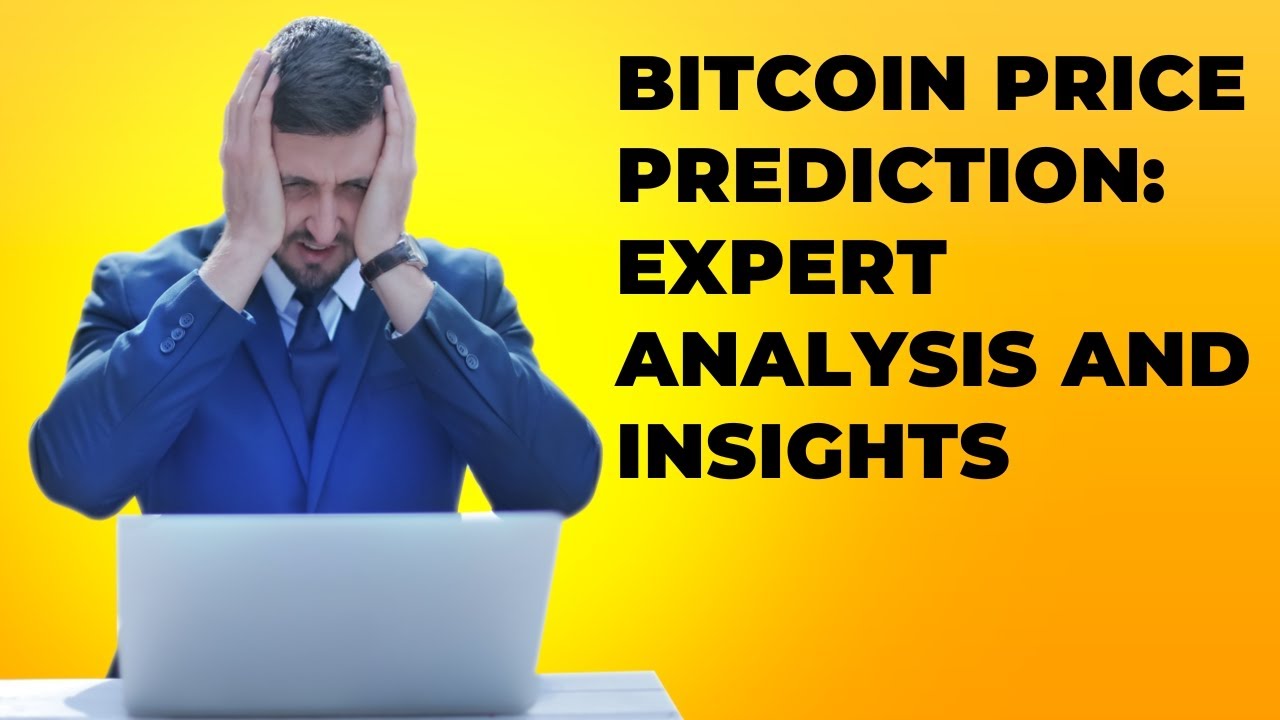
Predicting Bitcoin’s price with AI requires leveraging diverse data sources and sophisticated predictive models. The accuracy of these predictions hinges on the quality and comprehensiveness of the input data and the suitability of the chosen algorithm. A robust model needs to account for both technical indicators and the broader sentiment surrounding the cryptocurrency.AI models for Bitcoin price prediction rely on a multifaceted approach, incorporating various data streams to build a comprehensive picture of market dynamics.
The efficacy of these models is directly linked to the quality and relevance of the data they consume.
Key Data Sources for Bitcoin Price Prediction
The effectiveness of any AI model for Bitcoin price prediction is critically dependent on the quality and diversity of its input data. A wide range of data sources are commonly utilized, each offering unique insights into market behavior. These sources provide a holistic view, encompassing both quantitative and qualitative factors that influence Bitcoin’s price.
- On-chain metrics: These metrics, derived directly from the Bitcoin blockchain, provide insights into network activity and user behavior. Examples include transaction volume, mining difficulty, hash rate, the number of active addresses, and the distribution of Bitcoin holdings (e.g., the number of large wallets).
- Trading volume and price data: Historical price data from various exchanges, alongside trading volume information, are fundamental inputs. These data points reveal market trends, volatility, and liquidity.
- Social media sentiment: Analyzing sentiment expressed on platforms like Twitter and Reddit provides insights into public opinion and market psychology. This data is often processed using natural language processing (NLP) techniques to gauge overall sentiment (positive, negative, or neutral).
- Macroeconomic indicators: Broader economic factors, such as inflation rates, interest rates, and geopolitical events, can significantly impact Bitcoin’s price. Integrating macroeconomic data into the model adds context and improves predictive accuracy.
Predictive Models for Bitcoin Price Prediction
Several AI algorithms are employed to create predictive models for Bitcoin’s price. The selection of an appropriate algorithm depends on the specific characteristics of the data and the desired level of prediction accuracy. Two prominent examples are discussed below.
The creation of an effective predictive model requires careful consideration of various factors. Data preprocessing, feature selection, and model parameter tuning are crucial steps in ensuring the model’s accuracy and reliability.
Long Short-Term Memory (LSTM) Networks
LSTMs are a type of recurrent neural network (RNN) particularly well-suited for analyzing time-series data like Bitcoin’s price history. Their ability to retain information over long periods makes them ideal for capturing long-term trends and patterns.
- Data Preparation: The historical Bitcoin price data is cleaned, normalized, and formatted appropriately for the LSTM network. This might involve removing outliers, handling missing values, and scaling the data to a suitable range.
- Model Architecture: An LSTM network with multiple layers is designed, with the number of layers and neurons per layer determined through experimentation and hyperparameter tuning. The input layer receives the preprocessed price data, while the output layer predicts the future price.
- Training and Validation: The model is trained on a portion of the historical data, and its performance is evaluated on a separate validation set to prevent overfitting. This involves adjusting the model’s parameters (weights and biases) to minimize the prediction error.
- Prediction: Once trained, the model can be used to predict future Bitcoin prices by feeding it the most recent price data.
Support Vector Regression (SVR)
Support Vector Regression is a powerful machine learning algorithm capable of handling high-dimensional data and nonlinear relationships. It is particularly effective in identifying complex patterns within the data to predict future price movements.
- Feature Engineering: Relevant features from the various data sources are carefully selected and engineered. This may involve creating new features from existing ones (e.g., moving averages, technical indicators).
- Kernel Selection: An appropriate kernel function (e.g., radial basis function or polynomial kernel) is chosen to map the data into a higher-dimensional space where linear separation is possible.
- Model Training: The SVR model is trained using the selected features and the historical Bitcoin price data. The model learns to map the input features to the corresponding price targets.
- Hyperparameter Tuning: The model’s performance is optimized by adjusting hyperparameters such as the regularization parameter (C) and the kernel parameter (gamma).
Training and Validating an AI Model for Bitcoin Price Prediction
The training and validation process is crucial for building a reliable Bitcoin price prediction model. This iterative process involves several key steps to ensure the model’s accuracy and generalization capabilities.
A common approach involves splitting the available data into three sets: training, validation, and testing. The training set is used to train the model, the validation set to tune hyperparameters and prevent overfitting, and the testing set to evaluate the model’s performance on unseen data. This rigorous process helps to ensure that the model generalizes well to new, unseen data, which is essential for accurate prediction.
- Data Splitting: The dataset is divided into training, validation, and testing sets. A typical split might be 70% for training, 15% for validation, and 15% for testing.
- Model Training: The chosen AI algorithm is trained using the training dataset. This involves iteratively adjusting the model’s parameters to minimize the difference between the predicted and actual Bitcoin prices.
- Hyperparameter Tuning: The model’s hyperparameters are tuned using the validation set. This process involves experimenting with different hyperparameter values and selecting the combination that yields the best performance on the validation set.
- Model Evaluation: The final model is evaluated on the testing set to assess its performance on unseen data. Metrics such as mean squared error (MSE), root mean squared error (RMSE), and R-squared are commonly used to evaluate the model’s accuracy.
Factors Beyond AI’s Predictive Capabilities
Predicting Bitcoin’s price movements, even with sophisticated AI models, remains a challenging endeavor. While AI can analyze historical data and identify patterns, it cannot account for the inherent unpredictability of the cryptocurrency market, which is susceptible to a range of external factors that lie outside the scope of purely data-driven predictions. These external forces often introduce significant volatility and can drastically alter price trajectories, rendering even the most advanced AI predictions inaccurate.AI models, by their nature, are retrospective.
They learn from past data, but the future is rarely a simple extrapolation of the past. The cryptocurrency market is particularly susceptible to “black swan” events—highly improbable occurrences with significant consequences—that are, by definition, impossible to predict with any degree of certainty. The following sections detail some of these crucial factors that consistently undermine the predictive power of AI in the Bitcoin market.
Unforeseen Events and Their Impact on Bitcoin’s Price
Unexpected events, often categorized as “black swan” events, consistently disrupt established market trends and invalidate purely data-driven predictions. For instance, the collapse of the FTX exchange in November 2022 triggered a significant sell-off in the cryptocurrency market, including a sharp drop in Bitcoin’s price. This event, unforeseen by most market analysts and AI models alike, highlighted the vulnerability of the market to sudden shocks related to trust and regulatory issues.
Similarly, the 2020 COVID-19 pandemic initially caused a dramatic crash followed by a surprising surge in Bitcoin’s value, demonstrating how unexpected global events can override any AI-predicted trajectory. These instances underscore the limitations of relying solely on historical data for forecasting future price movements. The inherent unpredictability of such events renders purely data-driven AI predictions prone to significant errors.
Macroeconomic Factors and Bitcoin’s Price Volatility
Macroeconomic conditions exert a substantial influence on Bitcoin’s price, often overriding any AI-driven predictions. Inflation, for example, can drive investors towards Bitcoin as a hedge against inflation, leading to price increases. Conversely, rising interest rates, which make traditional investments more attractive, can cause a shift away from Bitcoin and lead to price declines. The correlation between Bitcoin’s price and macroeconomic indicators is not always consistent or predictable, making it difficult for AI models to accurately incorporate these factors into their predictions.
For instance, the Federal Reserve’s monetary policy decisions significantly impact the overall market sentiment and, consequently, Bitcoin’s price, often in ways that are not easily anticipated by AI algorithms.
Regulatory Changes and Geopolitical Events
Government regulations and geopolitical events represent another significant source of uncertainty that can drastically affect Bitcoin’s price, regardless of AI predictions. Changes in regulatory frameworks, such as the introduction of stricter KYC/AML rules or outright bans on cryptocurrency trading in certain jurisdictions, can dramatically impact market liquidity and investor confidence. Geopolitical instability, including international conflicts or sanctions, can also trigger significant price fluctuations as investors seek safe haven assets.
The Chinese government’s crackdown on cryptocurrency mining in 2021, for example, caused a notable decline in Bitcoin’s price, demonstrating the substantial influence of regulatory actions on the market. These unpredictable shifts in the regulatory and geopolitical landscape often overshadow any patterns identified by AI algorithms.
Illustrative Examples of AI Predictions and Outcomes
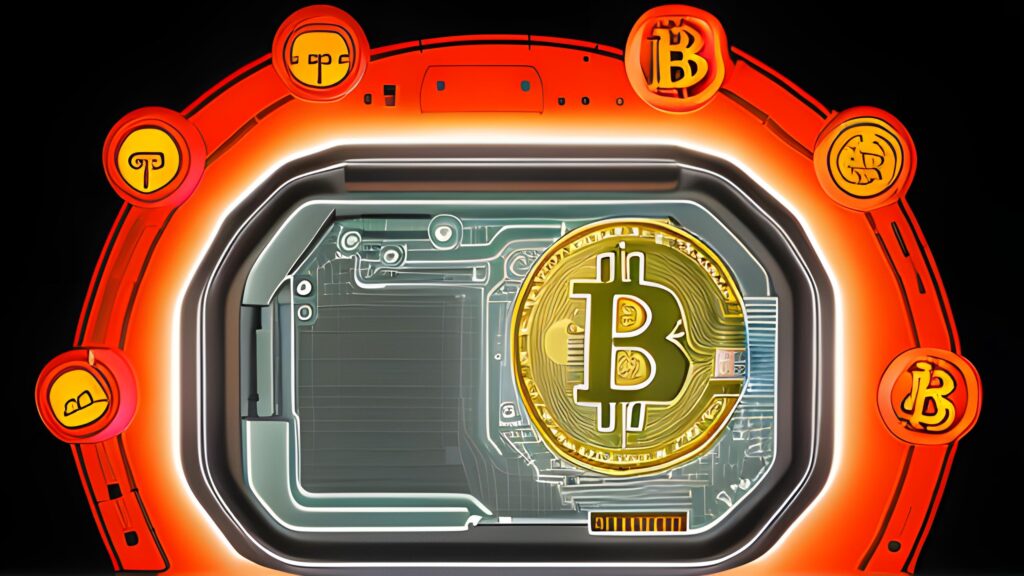
AI’s ability to predict Bitcoin bull runs is still under development, and its accuracy is far from perfect. Analyzing hypothetical scenarios and real-world examples helps illustrate the complexities involved and the limitations of current AI predictive models in the volatile cryptocurrency market.
Consider a hypothetical scenario where a sophisticated AI model, trained on a vast dataset encompassing Bitcoin’s price history, on-chain metrics, social media sentiment, and macroeconomic indicators, predicts a significant bull run in Q4
2024. The AI’s prediction is based on a confluence of factors: increasing institutional adoption, positive regulatory developments in a key jurisdiction, and a surge in on-chain activity suggesting growing user confidence.
The AI model projects a price increase to $100,000 by the end of the year, with a high degree of confidence (e.g., 85%).
Hypothetical AI Prediction and Market Response
Following the AI’s prediction, a wave of optimism sweeps through the cryptocurrency market. Retail investors, emboldened by the AI’s seemingly robust forecast, rush to buy Bitcoin, driving up the price initially. However, unexpected events intervene. A major global financial institution announces a significant pullback from cryptocurrency investments due to unforeseen regulatory hurdles. This, coupled with a sudden surge in inflation, causes a market correction, pushing the price down considerably.
By the end of Q4 2024, the Bitcoin price reaches only $60,000, significantly lower than the AI’s prediction. The discrepancy arises from the AI’s inability to fully account for unpredictable geopolitical events and regulatory changes that significantly impact investor sentiment and market dynamics.
Visual Representation of AI Prediction vs. Actual Price
A line graph would effectively illustrate the comparison. The horizontal axis represents time (e.g., months in Q4 2024). The vertical axis represents Bitcoin’s price in US dollars. Two lines would be plotted: one representing the AI’s predicted price trajectory, showing a steady incline to $100,000, and another representing the actual Bitcoin price, initially rising in response to the prediction but then experiencing a sharp downturn due to the unforeseen negative events, ultimately reaching $60,000.
The graph would clearly highlight the divergence between the AI’s prediction and the reality, emphasizing the inherent uncertainties in market forecasting.
Ethical Considerations of AI in Financial Markets
The use of AI in predicting and influencing financial markets raises several ethical concerns. The potential for market manipulation through algorithmic trading based on AI predictions is a significant risk. AI models could be used to create artificial price swings, benefiting those with access to the AI’s predictions while harming others. Furthermore, the opacity of some AI models makes it difficult to understand their decision-making processes, raising concerns about accountability and transparency.
The potential for bias in the data used to train AI models could lead to unfair or discriminatory outcomes. Ensuring fairness, transparency, and accountability in the use of AI in financial markets is crucial to mitigate these ethical risks.
Wrap-Up
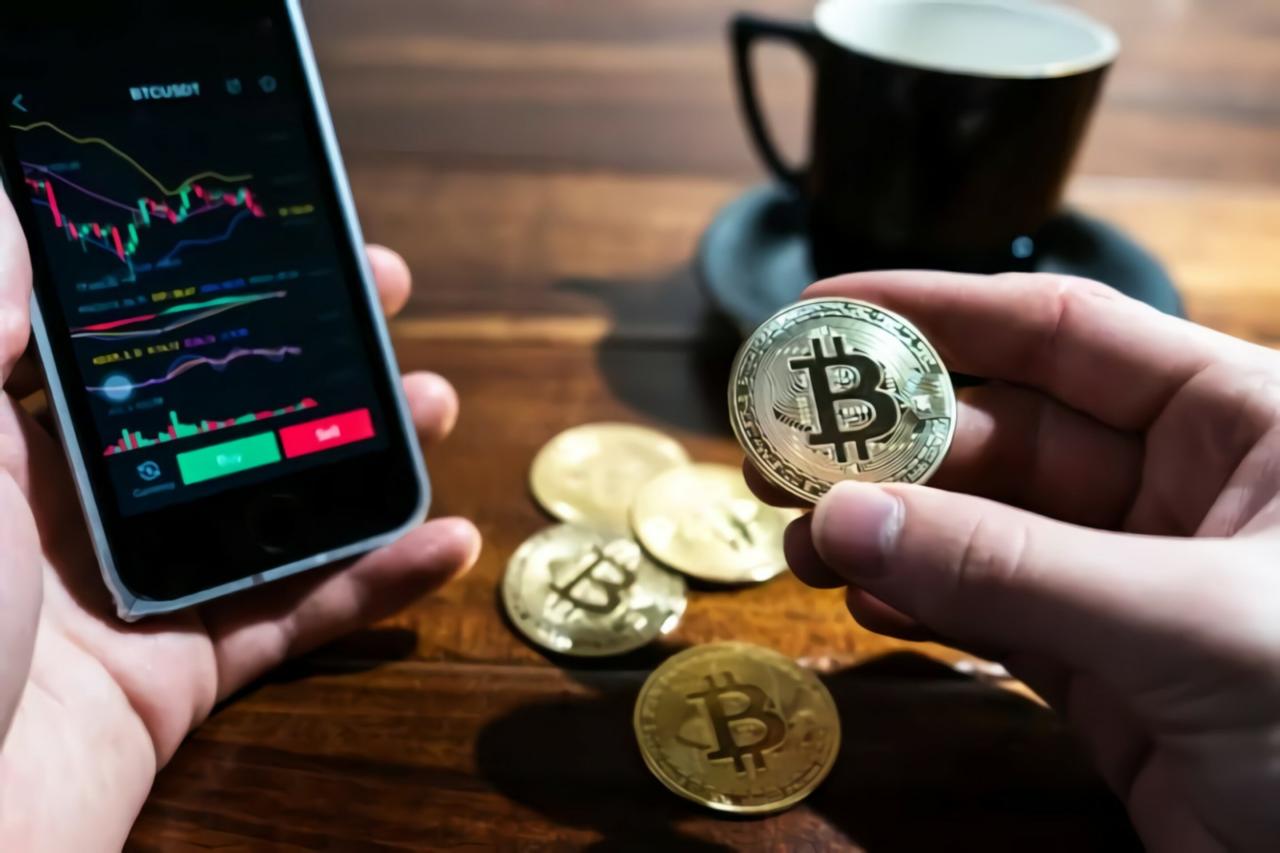
While AI offers powerful tools for analyzing market trends, predicting the next Bitcoin bull run remains a complex challenge. The inherent volatility of cryptocurrency, coupled with the influence of unpredictable global events, significantly limits the accuracy of even the most sophisticated AI models. While AI can identify patterns and correlations, it cannot account for unforeseen circumstances or the irrational exuberance (or fear) that often drives market behavior.
Therefore, relying solely on AI predictions for investment decisions is ill-advised. A balanced approach, incorporating AI insights alongside traditional market analysis and a thorough understanding of macroeconomic factors, offers a more robust strategy for navigating the dynamic Bitcoin market.