Ethical considerations of using AI in UI UX design – Ethical considerations of using AI in UI/UX design are paramount. As artificial intelligence increasingly shapes user interfaces and experiences, we face critical questions regarding bias, accessibility, privacy, transparency, and the very future of work in the field. This exploration delves into the ethical complexities of integrating AI, examining potential pitfalls and proposing strategies for responsible development and implementation.
From algorithmic bias potentially leading to discriminatory outcomes to the crucial need for data privacy and user consent, the ethical landscape of AI in UI/UX is multifaceted. We’ll analyze how AI can both enhance and compromise user experience, focusing on building trust and ensuring inclusivity for all.
Bias in AI-driven UI/UX Design
AI is rapidly transforming UI/UX design, offering powerful tools for personalization and recommendation. However, the algorithms powering these tools are not immune to bias, potentially leading to unfair or discriminatory user experiences. Understanding the sources and impacts of this bias is crucial for creating equitable and inclusive digital products.
Sources of Bias in Personalization and Recommendation Algorithms
Algorithmic bias in UI/UX stems from various sources. Data used to train AI models often reflects existing societal biases, perpetuating and even amplifying them. For example, if a dataset used to train a product recommendation system primarily features data from a specific demographic group, the system may learn to prioritize recommendations for that group, neglecting the needs and preferences of others.
Furthermore, the design choices made during the algorithm’s development, such as feature selection and model architecture, can inadvertently introduce bias. Finally, the lack of diversity within the teams developing these algorithms can contribute to a blind spot regarding potential biases affecting underrepresented user groups. These factors combine to create systems that may not serve all users equally.
Impact of Biased Algorithms on User Experience Across Demographic Groups
Biased algorithms can significantly impact user experience, leading to disparities across different demographic groups. For instance, a biased job search algorithm might prioritize displaying job postings to specific genders or ethnicities, hindering opportunities for others. Similarly, a biased loan application system might unfairly deny credit to certain demographics, based on flawed correlations learned from biased data. These experiences can lead to frustration, feelings of exclusion, and reduced trust in the platform or service.
The impact extends beyond individual users, potentially reinforcing existing societal inequalities and creating a less inclusive digital environment.
Hypothetical Scenario: Algorithmic Bias in an E-commerce Platform
Consider an e-commerce platform using AI-driven product recommendations. The training data for the recommendation engine primarily features purchase history from a younger, predominantly affluent demographic. As a result, the algorithm learns to prioritize recommendations for expensive, trendy items, neglecting products that might be more relevant or affordable for older users or those with lower incomes.
User Group | Recommended Products | Relevance | User Experience |
---|---|---|---|
Young, Affluent Users | High-end fashion, luxury electronics, premium experiences | High | Positive; feels personalized and relevant |
Older Users with Lower Incomes | Limited selection; mostly expensive items irrelevant to their needs | Low | Negative; feels excluded and underserved; may abandon the platform |
Accessibility and Inclusivity in AI-powered UI/UX
AI’s potential to revolutionize UI/UX design extends beyond mere aesthetics; it offers powerful tools to enhance accessibility and inclusivity for users with diverse needs and abilities. By leveraging AI’s capabilities, designers can create more user-friendly and equitable digital experiences, breaking down barriers that traditionally exclude certain user groups. This section will explore how AI can be implemented responsibly to achieve these goals.AI’s role in improving accessibility features significantly impacts user experience.
The technology can analyze user interactions, identify potential accessibility issues, and suggest design modifications to improve usability for people with disabilities. This proactive approach moves beyond reactive adjustments, leading to more inclusive designs from the outset.
AI-Driven Enhancement of Accessibility Features
AI can analyze website content and automatically generate alternative text for images, improving accessibility for visually impaired users who rely on screen readers. Furthermore, AI can transcribe audio content into text, making podcasts and videos accessible to those with hearing impairments. AI-powered tools can also automatically generate captions and subtitles, enhancing accessibility across various media formats. For example, a tool could analyze a video’s audio track and generate accurate captions, reducing the manual effort required and improving accuracy.
Another example is an AI system that analyzes website layouts and suggests improvements to color contrast, font size, and keyboard navigation, making the site more accessible to users with visual or motor impairments. Such systems can identify issues like insufficient color contrast between text and background, which can be difficult for users with low vision to discern.
Strategies for Inclusive AI-Driven Design Solutions
Ensuring inclusivity in AI-driven design necessitates a multifaceted approach. Firstly, diverse user groups must be actively involved in the design process from the initial stages. This includes users with various disabilities, age groups, cultural backgrounds, and technological literacy levels. Their feedback is crucial for identifying potential barriers and shaping solutions that truly cater to their needs. Secondly, employing AI algorithms that are trained on diverse and representative datasets is essential.
Biased datasets can lead to AI systems that perpetuate and even amplify existing inequalities. For instance, an AI system trained primarily on data from one demographic may not accurately recognize or respond to the needs of other demographics. Thirdly, rigorous testing and evaluation with diverse user groups are vital to identify and address any unforeseen accessibility issues.
This iterative process helps refine the design and ensures that the final product is truly inclusive. Finally, ongoing monitoring and feedback mechanisms are crucial for continually improving accessibility and inclusivity. Regularly collecting user feedback and analyzing usage patterns can help identify areas for improvement and ensure that the AI-driven design remains relevant and effective for all users.
Ethical Implications of Excluding User Groups
Excluding certain user groups from the design process or the benefits of AI-driven solutions has significant ethical implications. It reinforces existing inequalities and can lead to a digital divide, where certain populations are left behind in the digital world. This exclusion can have far-reaching consequences, impacting access to information, services, and opportunities. For example, if an AI-powered healthcare application is not designed to be accessible to visually impaired users, it limits their ability to manage their health effectively.
Similarly, excluding users with motor impairments from the design process can lead to applications that are unusable for them. Failing to consider diverse user needs not only violates principles of ethical design but also limits the potential reach and impact of AI-driven solutions. The focus should be on creating universally accessible designs that empower all users, regardless of their abilities or backgrounds.
Data Privacy and Security in AI-powered UI/UX: Ethical Considerations Of Using AI In UI UX Design
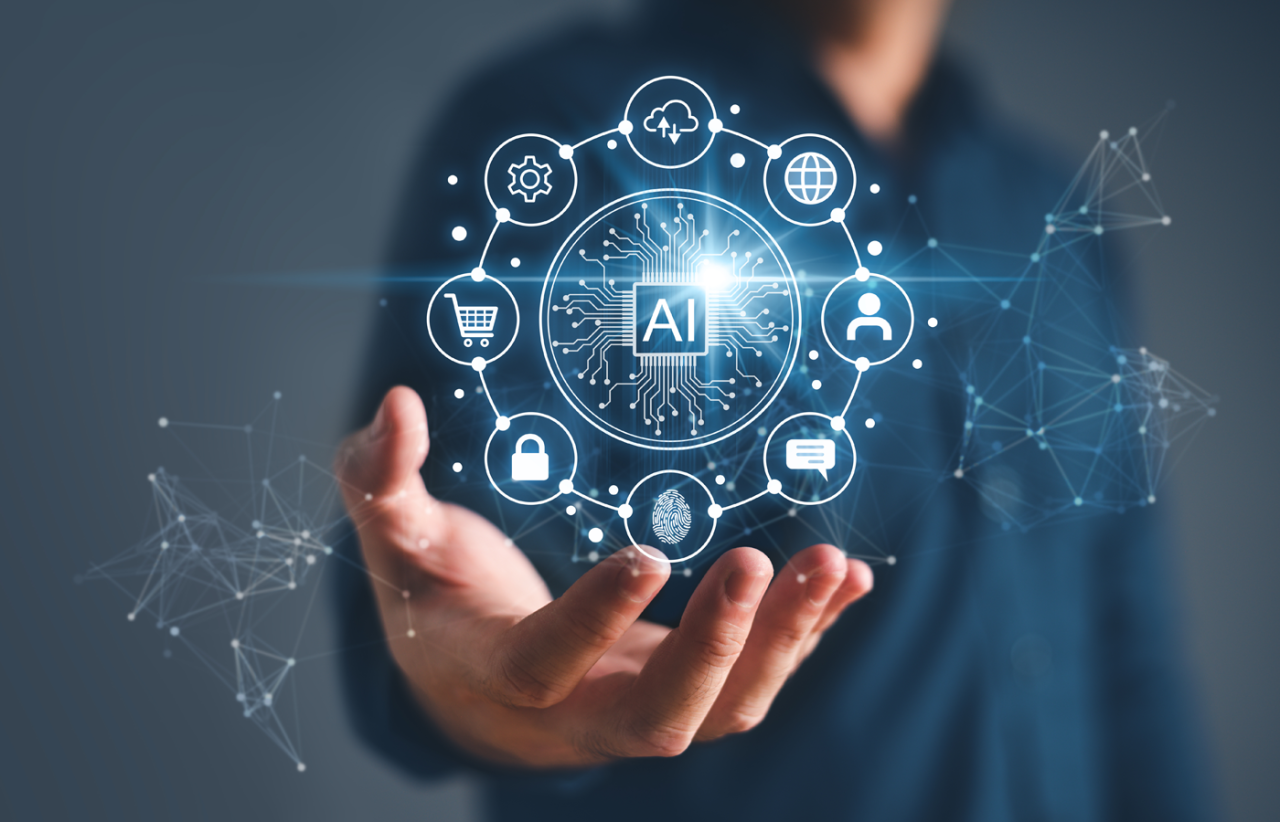
The increasing reliance on AI in UI/UX design presents significant ethical challenges concerning user data. AI systems, particularly those employing machine learning, require vast amounts of data to function effectively. This data often includes sensitive personal information, raising concerns about privacy violations and potential misuse. Balancing the benefits of personalized user experiences with the fundamental right to privacy is a critical design consideration.
Transparency and user control over data collection and usage are paramount.The collection, storage, and use of user data in AI-driven UI/UX must adhere to stringent ethical guidelines. This involves clearly informing users about what data is being collected, why it’s being collected, how it will be used, and who will have access to it. Furthermore, robust security measures must be implemented to prevent data breaches and unauthorized access.
The potential for algorithmic bias in data analysis and the subsequent impact on user experience also necessitates careful consideration and mitigation strategies. Data minimization—collecting only the data strictly necessary—should be a guiding principle.
Data Anonymization and Encryption Techniques
Several techniques exist to protect user privacy during the development and deployment of AI-powered UI/UX systems. Data anonymization aims to remove or obscure identifying information from datasets, while encryption protects data by transforming it into an unreadable format. Choosing the appropriate method depends on the sensitivity of the data and the level of protection required.
Data anonymization techniques include data masking (replacing sensitive data with non-sensitive substitutes), generalization (replacing specific values with broader categories), and pseudonymization (replacing identifying information with pseudonyms). However, perfect anonymization is difficult to achieve, and re-identification remains a possibility with sophisticated techniques. Therefore, anonymization should be considered a risk mitigation strategy rather than a guarantee of complete privacy.
Encryption, on the other hand, involves transforming data into ciphertext using a cryptographic key. Symmetric encryption uses the same key for encryption and decryption, while asymmetric encryption uses a pair of keys (a public key for encryption and a private key for decryption). Asymmetric encryption is particularly useful for secure communication and data protection in distributed systems. Strong encryption algorithms, such as AES (Advanced Encryption Standard) and RSA (Rivest-Shamir-Adleman), are essential for safeguarding user data.
The choice between symmetric and asymmetric encryption depends on the specific application and security requirements. For example, symmetric encryption might be preferred for encrypting large datasets due to its higher speed, while asymmetric encryption is better suited for secure key exchange and digital signatures.
Example Privacy Policy for an AI-Powered Application
This hypothetical privacy policy demonstrates how a company can transparently communicate its data handling practices to users of an AI-powered application:
Privacy Policy for “SmartHomeAssist”
SmartHomeAssist collects user data to personalize your experience and improve our service. This data includes your device usage patterns, preferences, and location data (only when explicitly enabled by the user). We use encryption to protect your data during transmission and storage. Your data is anonymized where possible and only used for internal purposes to improve the application’s functionality.
We do not share your data with third parties except as required by law. You have the right to access, modify, or delete your data at any time. By using SmartHomeAssist, you consent to this privacy policy. For further details, please refer to our comprehensive privacy policy available on our website.
Transparency and Explainability of AI in UI/UX
The increasing integration of artificial intelligence (AI) into UI/UX design presents a critical challenge: ensuring transparency and explainability. Users need to understand how AI influences their interactions, fostering trust and allowing for informed decision-making. A lack of transparency can lead to user distrust, reduced engagement, and even legal repercussions. Therefore, designing for transparency is not merely a best practice but a necessity for responsible AI implementation in UI/UX.AI’s inherent complexity often obscures its decision-making processes.
Many algorithms, particularly deep learning models, operate as “black boxes,” making it difficult to trace the reasoning behind their outputs. This opacity poses a significant hurdle to building user trust and understanding. Furthermore, the sheer volume of data processed by AI systems can make it challenging to pinpoint the specific factors contributing to a particular outcome, further complicating efforts to achieve transparency.
Effectively communicating the role and limitations of AI in the user experience is crucial for mitigating these challenges.
Challenges in Achieving Transparency and Understandability
Achieving transparency in AI-driven UI/UX design presents several interconnected challenges. First, the technical complexity of many AI algorithms makes it difficult to translate their inner workings into easily understandable terms for non-technical users. Second, even when explanations are possible, conveying them in a way that is both accurate and accessible requires careful design considerations. Third, balancing the need for transparency with the need to protect proprietary algorithms and data poses a significant dilemma for developers.
Finally, the evolving nature of AI technology requires ongoing efforts to adapt and refine transparency strategies as new algorithms and applications emerge. Addressing these challenges requires a multi-faceted approach, combining technical solutions with thoughtful design choices.
Designing UI Elements for AI Transparency
Clear communication about AI involvement is paramount. UI elements can play a vital role in achieving this. For example, a simple indicator, such as a small icon or a subtle text label, could inform users when an AI system is involved in personalizing their experience or making recommendations. This could be as simple as a small gear icon next to a personalized recommendation with the tooltip reading “AI-powered suggestion”.
Another approach is to provide users with brief explanations of how the AI system works and the factors influencing its decisions, presented in a clear and concise manner, perhaps within a help section or FAQ. In cases where the AI’s decision-making process is particularly complex, providing a simplified overview or summary can be more effective than attempting to fully explain the underlying mechanisms.
The key is to strike a balance between providing sufficient information without overwhelming the user.
Best Practices for Explainable and Accountable AI in UI/UX, Ethical considerations of using AI in UI UX design
Implementing explainable and accountable AI systems in UI/UX requires a proactive approach.
- Prioritize User-Centric Design: Involve users throughout the design process to understand their needs and expectations regarding AI transparency. Conduct user research to identify preferred methods for communicating AI involvement and to assess the effectiveness of different design approaches.
- Employ Explainable AI (XAI) Techniques: Integrate XAI techniques into the AI system itself to make its decision-making processes more understandable. This may involve using algorithms that are inherently more transparent or developing methods for visualizing and interpreting the AI’s internal workings.
- Provide Clear and Concise Explanations: Avoid technical jargon and present information in a way that is easily accessible to users with varying levels of technical expertise. Use visual aids, such as charts and diagrams, to enhance understanding.
- Offer User Control and Agency: Allow users to control the level of AI involvement in their experience and to override AI-driven suggestions when desired. This empowers users and fosters trust.
- Establish Clear Accountability Mechanisms: Implement procedures for addressing user concerns and complaints related to AI-driven decisions. Ensure that there are clear channels for feedback and mechanisms for resolving disputes.
- Regularly Audit and Evaluate AI Systems: Conduct ongoing audits to assess the fairness, accuracy, and transparency of AI systems. Use this information to continuously improve the design and functionality of AI-powered UI/UX elements.
Job Displacement and the Future of Work in AI-driven UI/UX
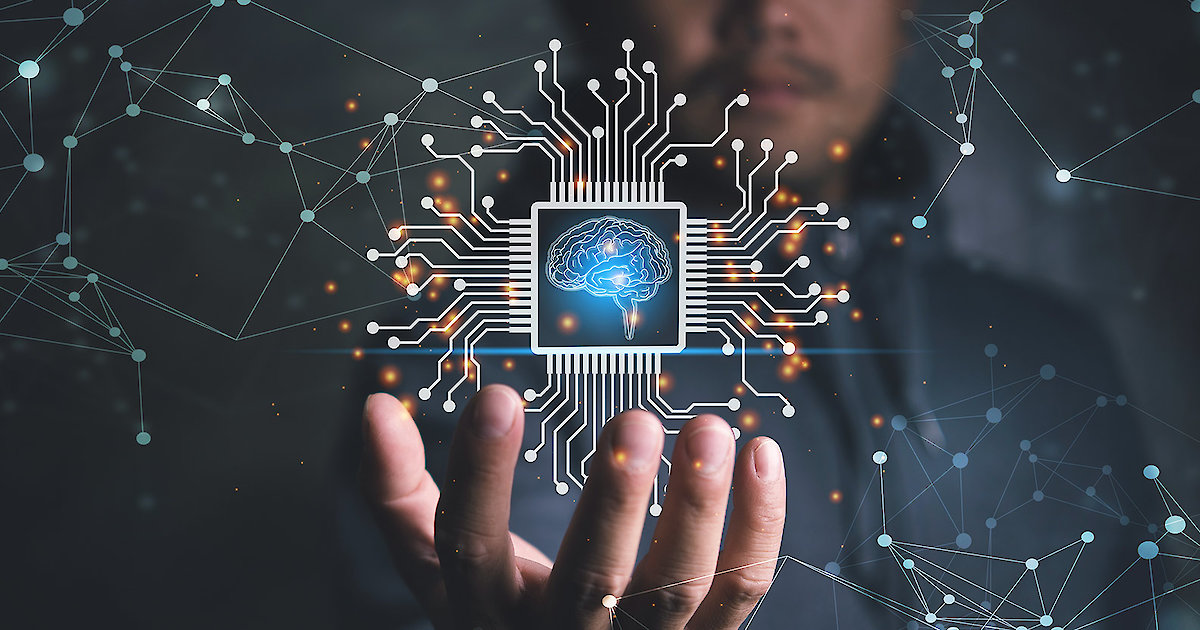
The rise of artificial intelligence (AI) in UI/UX design presents both exciting opportunities and significant challenges, particularly concerning the future of work for designers and related professionals. While AI tools promise increased efficiency and automation of certain tasks, concerns about job displacement are valid and require careful consideration. This section explores the potential impact of AI on UI/UX roles and responsibilities, strategies for mitigating negative employment consequences, and a potential training program to equip designers for the evolving landscape.AI’s impact on UI/UX roles is multifaceted.
Automation of repetitive tasks, such as generating basic layouts or creating variations of design elements, is already underway. This could lead to a reduction in demand for entry-level designers focused on these specific tasks. However, the core skills of UX research, user empathy, strategic design thinking, and complex problem-solving remain crucial and are less susceptible to automation.
Instead of replacing designers entirely, AI is more likely to augment their capabilities, allowing them to focus on higher-level tasks requiring creative thinking and strategic decision-making. For example, AI can assist in analyzing user data to identify patterns and inform design choices, but the interpretation of this data and the creative application of those insights remain the purview of human designers.
The Impact of AI on UI/UX Roles and Responsibilities
AI tools are increasingly capable of automating tasks previously handled by human designers. This includes generating design variations based on input parameters, automating basic coding tasks, and performing A/B testing analysis. Consequently, roles focused on these specific tasks may experience decreased demand. Conversely, roles requiring complex problem-solving, strategic thinking, and advanced user research skills will likely remain in high demand.
The focus will shift towards designers who can effectively leverage AI tools to enhance their workflow and create innovative solutions, rather than those who perform solely manual tasks. The demand for professionals skilled in integrating AI tools into the design process, including prompt engineering and AI model fine-tuning, will significantly increase.
Strategies for Mitigating Negative Employment Consequences
Several strategies can mitigate the potential negative impact of AI on UI/UX employment. Firstly, investing in continuous learning and upskilling is crucial. Designers must adapt to the changing landscape by acquiring expertise in AI tools and techniques. Secondly, focusing on developing uniquely human skills, such as critical thinking, emotional intelligence, and complex problem-solving, will become increasingly important to remain competitive.
Thirdly, fostering collaboration between human designers and AI tools will be essential. AI should be viewed as a collaborator, not a replacement, enabling designers to enhance their efficiency and creative output. Finally, promoting ethical considerations and responsible AI implementation in the design process will ensure that AI tools are used to benefit users and society as a whole.
This includes addressing biases in AI algorithms and ensuring accessibility for all users.
A Training Program for Upskilling UI/UX Professionals
A comprehensive upskilling program for UI/UX professionals should incorporate several key elements. The program should begin with foundational training in AI concepts and the ethical considerations surrounding its use in design. This would be followed by practical training on specific AI tools and technologies relevant to UI/UX, including generative design tools, AI-powered prototyping platforms, and user data analysis tools.
The curriculum should also include modules focused on developing advanced skills such as prompt engineering, AI model fine-tuning, and data interpretation. Finally, the program should emphasize the importance of human-centered design principles and the ethical implications of AI in ensuring inclusivity and accessibility in the design process. This multifaceted approach would equip UI/UX professionals with the necessary skills to thrive in the AI-driven future of the industry.
The program could be delivered through a combination of online courses, workshops, and mentorship opportunities. Real-world case studies and hands-on projects would be integral to the learning experience, ensuring that participants gain practical skills and experience.
Responsibility and Accountability in AI-driven UI/UX Failures
The increasing reliance on AI in UI/UX design necessitates a robust framework for assigning responsibility and accountability when these systems malfunction or generate undesirable outcomes. This is crucial not only for maintaining user trust and safety but also for fostering ethical development and deployment of AI in this rapidly evolving field. Without clear lines of responsibility, the potential for harm—from minor inconveniences to significant biases—is amplified.AI systems, even those seemingly sophisticated, are ultimately tools.
Their performance is dependent on the data they are trained on, the algorithms governing their behavior, and the human decisions made during their design, implementation, and deployment. Therefore, establishing accountability requires a multi-faceted approach that considers all these contributing factors.
Defining Roles and Responsibilities
Establishing clear lines of responsibility involves defining the roles and responsibilities of various stakeholders throughout the AI lifecycle. This includes the data scientists who prepare the training data, the developers who build and maintain the AI models, the UI/UX designers who integrate the AI into the user interface, and the company leadership that oversees the entire process. A well-defined responsibility matrix, outlining who is accountable for specific aspects of the AI system’s development and performance, is essential.
For example, the data scientists are responsible for ensuring the data used to train the AI is unbiased and representative, while the developers are responsible for building robust error-handling mechanisms. The UI/UX designers ensure that the AI’s outputs are presented clearly and understandably to users. Finally, company leadership bears the ultimate responsibility for overseeing the ethical considerations and compliance with relevant regulations.
Implementing Robust Error Detection and Recovery Mechanisms
Robust error detection and recovery mechanisms are paramount to mitigating the impact of AI failures in UI/UX. This involves integrating mechanisms that can identify anomalies in the AI’s behavior, such as unexpected outputs or inconsistencies in performance. These mechanisms might include monitoring systems that track key performance indicators (KPIs) and alert developers to potential issues. Furthermore, AI systems should be designed with fail-safes, allowing for graceful degradation or fallback mechanisms when errors occur.
For instance, if an AI-powered recommendation system malfunctions, a default system could be triggered, providing basic recommendations until the issue is resolved. Regular testing and validation of the AI system are also critical, simulating various scenarios to identify potential weaknesses and vulnerabilities. This proactive approach can help prevent major failures and minimize their impact on users.
Ethical Implications of Different Approaches to Assigning Responsibility
Different approaches to assigning responsibility for AI-related failures have distinct ethical implications. A purely technical approach, focusing solely on identifying coding errors or algorithmic flaws, may overlook the broader ethical context. Conversely, assigning responsibility solely to the company leadership might overlook the contributions and potential negligence of other stakeholders. A balanced approach is necessary, considering the roles and responsibilities of all stakeholders while acknowledging the limitations of AI systems.
This includes establishing mechanisms for transparency and accountability, enabling users to understand how AI impacts their experience and report any issues. Furthermore, clear communication channels should be established between developers, designers, and users to facilitate quick identification and resolution of problems. A strong emphasis on user feedback and continuous improvement is vital in fostering trust and mitigating ethical risks associated with AI failures.
The Impact of AI on User Trust and Confidence
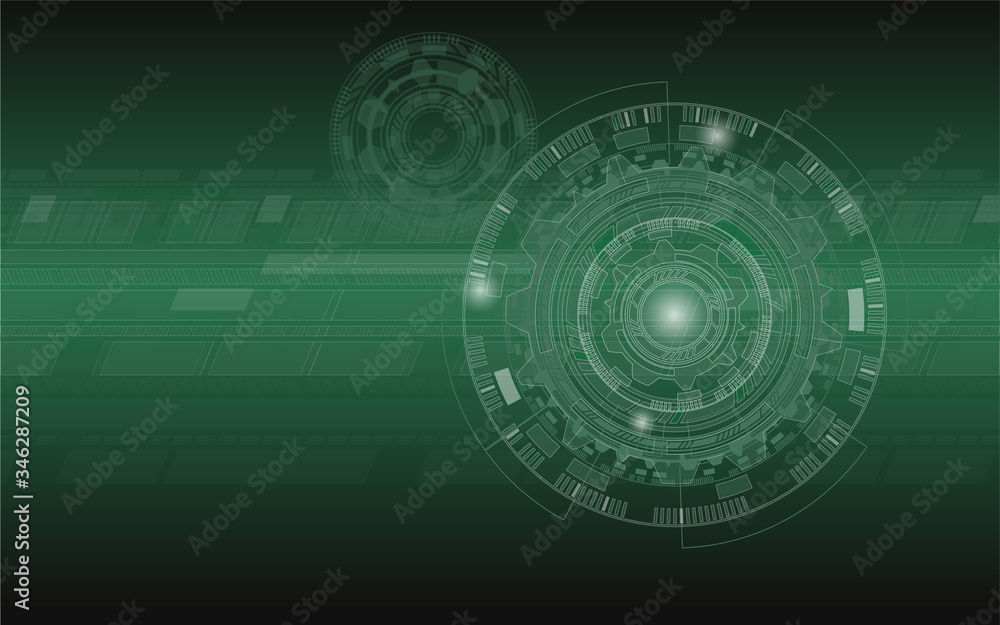
The integration of artificial intelligence (AI) into UI/UX design presents a double-edged sword. While AI offers the potential to create highly personalized and efficient user experiences, its deployment can also significantly impact user trust and confidence, either positively or negatively. Understanding this impact is crucial for designers to leverage AI’s benefits while mitigating potential risks.AI’s influence on user trust hinges on several factors, including the perceived transparency of its operations, the accuracy and reliability of its recommendations, and the overall user experience it facilitates.
A lack of transparency can lead to distrust, while a consistently positive and helpful experience can foster confidence. This delicate balance necessitates careful consideration of ethical implications and user-centered design principles.
Factors Influencing User Perception and Acceptance of AI-powered UI/UX Elements
User acceptance of AI in UI/UX is not solely dependent on technological sophistication; it’s deeply intertwined with psychological and sociological factors. Trust, a fundamental element in human-computer interaction, is significantly influenced by perceived competence, benevolence, and integrity of the AI system. Competence refers to the system’s ability to perform its tasks accurately and efficiently. Benevolence relates to the user’s belief that the AI acts in their best interests.
Integrity reflects the consistency and predictability of the AI’s behavior. A lack in any of these areas can severely undermine user trust. For instance, an AI-powered recommendation system that consistently provides irrelevant or inaccurate suggestions will quickly erode user confidence. Conversely, a system that transparently explains its reasoning and consistently delivers helpful results will foster a positive user experience and build trust over time.
Examples of AI Building or Eroding User Trust
AI can build trust through personalized experiences tailored to individual user needs and preferences. For example, a music streaming service using AI to curate personalized playlists based on listening history demonstrates competence and benevolence, fostering user trust and engagement. Similarly, AI-powered chatbots that provide quick and accurate responses to customer queries can enhance customer satisfaction and build trust in the brand.Conversely, AI can erode trust through biases embedded in algorithms, leading to unfair or discriminatory outcomes.
A job recruitment platform using AI to screen candidates might inadvertently discriminate against certain demographic groups if the training data reflects existing societal biases. This can lead to a loss of user trust and even legal repercussions. Another example is an AI-powered image recognition system that misidentifies individuals or objects, leading to inaccurate results and a diminished sense of reliability.
The lack of transparency in how these systems operate further exacerbates the problem.
Visual Representation of AI Transparency, User Understanding, and Trust
Imagine a three-dimensional graph. The X-axis represents AI transparency (low to high), the Y-axis represents user understanding (low to high), and the Z-axis represents user trust (low to high). A positive correlation exists between all three variables. The graph would show a surface that slopes upward as AI transparency and user understanding increase, resulting in higher user trust.
Points on the graph represent specific AI applications; those with high transparency and user understanding would be clustered at the high end of the Z-axis, indicating high user trust. Conversely, applications lacking transparency and user understanding would cluster at the low end of the Z-axis, reflecting low user trust. The slope of the surface illustrates that even with high transparency, a lack of user understanding can still limit trust.
Similarly, high user understanding alone may not build trust without sufficient transparency in the AI system’s workings.
Last Recap
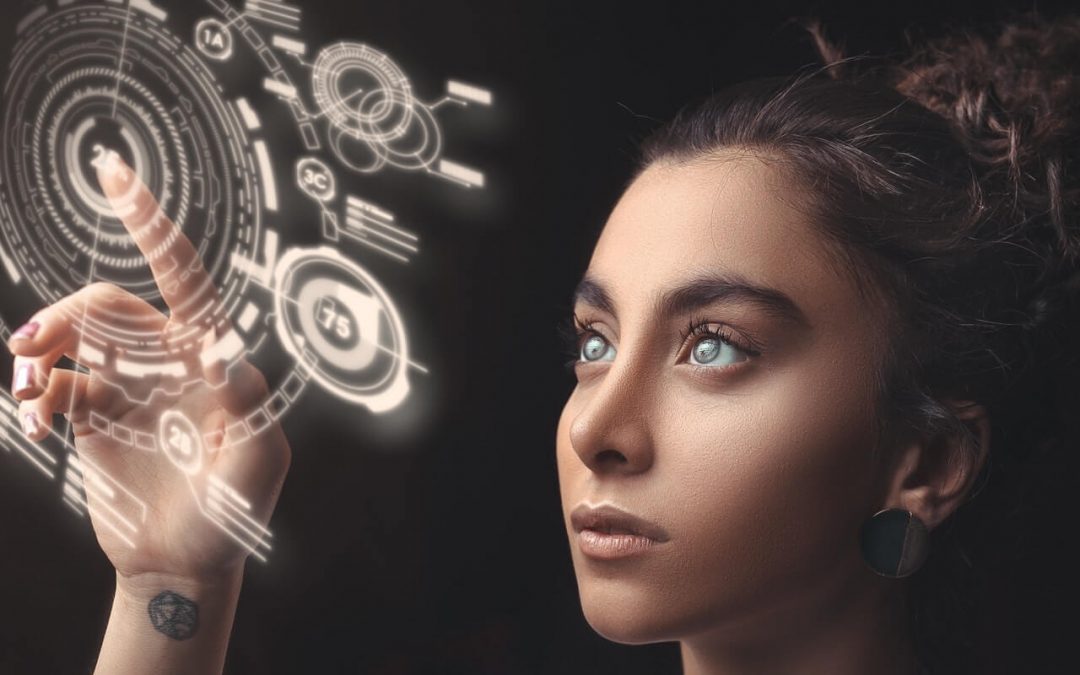
Ultimately, the ethical integration of AI in UI/UX demands a proactive and responsible approach. By acknowledging and addressing the potential challenges, from algorithmic bias to job displacement, we can harness the power of AI to create truly inclusive, accessible, and trustworthy digital experiences. This requires a collaborative effort among designers, developers, policymakers, and users to establish ethical guidelines and best practices that prioritize user well-being and societal benefit.