Exploring AI’s capabilities in video editing stabilization reveals a transformative shift in video production. No longer confined to complex, manual processes, stabilization now leverages the power of artificial intelligence, offering unprecedented accuracy and efficiency. This exploration delves into the algorithms, software, applications, and future potential of AI-powered video stabilization, examining its impact across various fields and addressing its current limitations.
From deep learning algorithms analyzing pixel movements to sophisticated software packages offering real-time adjustments, AI is revolutionizing how we achieve smooth, professional-looking video. We’ll compare leading software, dissect their features, and assess the hardware requirements for optimal performance. Furthermore, we’ll examine the application of AI stabilization in professional filmmaking, sports broadcasting, surveillance, and virtual reality, highlighting both its successes and its ongoing challenges.
AI-Powered Video Stabilization
Video stabilization has evolved significantly, transitioning from simple image-based techniques to sophisticated AI-driven algorithms. Early methods relied on identifying and compensating for camera motion using basic mathematical calculations applied to individual frames. These methods, while effective in certain scenarios, often struggled with complex movements or low-light conditions, leading to blurry or distorted results. The advent of AI, particularly deep learning, revolutionized the field, enabling far more robust and accurate stabilization.AI-based video stabilization leverages the power of machine learning to analyze video sequences and identify subtle movements and distortions that traditional methods miss.
These algorithms learn from vast datasets of stabilized and unstabilized videos, enabling them to predict and correct for a wide range of camera movements, including shakes, jitters, and rotations. The core principle lies in the ability of AI models to identify patterns and relationships within the video frames that indicate motion, and then apply corresponding transformations to create a smoother, more stable output.
This process is far more nuanced and adaptive than older methods, resulting in significantly improved video quality.
AI Algorithms in Video Stabilization
Several AI algorithms are commonly employed in video stabilization. Deep learning, a subset of machine learning, is particularly effective due to its ability to learn complex patterns from large datasets. Convolutional Neural Networks (CNNs) are a prominent type of deep learning architecture frequently used in this context. CNNs excel at processing visual data, enabling them to effectively analyze the spatial relationships between pixels in video frames and identify motion patterns.
These networks can learn to differentiate between intentional camera movements (like panning or zooming) and unwanted shakes or vibrations, applying stabilization only where necessary. For example, a CNN might be trained on a massive dataset of shaky footage paired with its stabilized counterpart, learning to map the unstable frames to their stabilized versions. This learned mapping is then applied to new, unseen footage to achieve stabilization.
Other algorithms, like recurrent neural networks (RNNs), can be used to incorporate temporal information from consecutive frames, improving the accuracy and smoothness of the stabilization process. The choice of algorithm often depends on factors like computational resources and the desired level of stabilization quality.
Key Features and Capabilities of AI Stabilization Software
AI-powered video stabilization software has revolutionized post-production, offering significantly improved results compared to traditional methods. These advancements stem from sophisticated algorithms that analyze video frames to identify and correct for camera shake, resulting in smoother, more professional-looking footage. This section delves into the key features and capabilities of these tools, comparing their performance and exploring the impact of hardware on processing.
Comparison of AI-Powered Video Stabilization Software
Several AI-powered video stabilization software packages are available, each with strengths and weaknesses. A direct comparison across all available options is difficult due to the constantly evolving nature of the software and the lack of standardized benchmarking tests. However, we can illustrate typical differences using a representative sample. The following table offers a simplified comparison, focusing on key performance indicators.
Note that actual performance can vary based on factors such as video resolution, content complexity, and hardware specifications.
Feature | Software A (Example) | Software B (Example) | Software C (Example) |
---|---|---|---|
Processing Speed (approx. time for 1-minute 1080p video) | 5-10 minutes | 2-5 minutes | 10-20 minutes |
Stabilization Accuracy (Subjective Rating: 1-5, 5 being best) | 4 | 4.5 | 3.5 |
Supported Video Formats | MP4, MOV, AVI, WMV | MP4, MOV, AVI, MKV, ProRes | MP4, MOV |
GPU Acceleration Support | Yes | Yes | No |
Advanced Features (Object Tracking, Perspective Correction) | Yes | Yes | No |
Advanced Features of AI Stabilization Tools
Beyond basic stabilization, many AI-powered tools offer advanced features that significantly enhance video quality and workflow efficiency. Object tracking, for instance, allows for smooth stabilization even when the camera follows a moving subject, maintaining focus and minimizing unwanted blurring. Perspective correction addresses distortions caused by tilting or angling the camera, resulting in a more natural and visually appealing final product.
Noise reduction further refines the video by minimizing grain and other artifacts, improving overall clarity. These advanced capabilities contribute to a more polished and professional final result.
Impact of Hardware Specifications on Performance
The performance of AI-based video stabilization is heavily influenced by the capabilities of the underlying hardware. A more powerful CPU (Central Processing Unit) is crucial for handling the complex calculations involved in analyzing and processing video frames. A dedicated GPU (Graphics Processing Unit) accelerates the process considerably, significantly reducing processing time, particularly for high-resolution videos. Sufficient RAM (Random Access Memory) is essential to hold the video data and intermediate processing results, preventing bottlenecks and ensuring smooth operation.
For example, stabilizing a 4K video will require significantly more processing power and RAM compared to a 720p video. A system with a high-end CPU, a powerful GPU, and ample RAM will deliver significantly faster processing times and better stabilization quality compared to a system with less powerful components. Users should expect longer processing times and potentially lower quality results on older or less powerful hardware.
Applications of AI Video Stabilization in Various Fields
AI video stabilization has transcended its initial niche applications, becoming an indispensable tool across numerous sectors demanding high-quality, smooth video content. Its ability to correct shaky footage, regardless of the source, has significantly improved the visual experience and opened new creative possibilities. This section explores the impact of AI stabilization in diverse fields, highlighting its practical benefits and showcasing specific examples.AI video stabilization offers significant advantages across a wide range of applications.
Its efficiency in processing large volumes of video data, coupled with its ability to handle complex stabilization challenges, makes it a valuable asset in professional and consumer contexts alike. The accuracy and speed provided by AI algorithms far surpasses traditional methods, leading to improved workflows and higher quality final products.
AI Video Stabilization in Professional Filmmaking and Video Production
The integration of AI-powered stabilization tools has revolutionized professional filmmaking and video production. Previously, achieving perfectly smooth shots often required extensive and costly post-production work, involving manual stabilization techniques and specialized equipment. Now, AI algorithms can automatically analyze footage and apply sophisticated stabilization techniques, resulting in smoother videos with minimal effort. This allows filmmakers to focus on creative aspects, rather than being bogged down by technical challenges.
For example, handheld shots, often characterized by unintentional camera movement, can now be stabilized to a professional level, providing a more polished and cinematic look. This technology also enables the creation of visually stunning shots previously deemed impossible due to limitations in camera stabilization equipment. The use of gimbals and other stabilization equipment can be minimized or even eliminated in certain scenarios, leading to increased flexibility and efficiency on set.
AI Stabilization in Sports Broadcasting, Surveillance, and Drone Footage
The benefits of AI video stabilization extend beyond traditional filmmaking. In sports broadcasting, shaky camera footage, particularly from handheld cameras or those mounted on moving vehicles, is a common issue. AI stabilization algorithms can significantly improve the viewing experience by smoothing out these imperfections, providing viewers with a clearer and more stable view of the action. Similarly, in surveillance applications, AI stabilization ensures that recordings remain clear and easily viewable, even when captured from unstable platforms or in challenging environments.
The ability to maintain a stable view of a scene is crucial for effective monitoring and analysis. Drone footage, often characterized by significant vibrations and camera movements, benefits greatly from AI stabilization. The technology can smooth out the jerky movements, revealing details that would otherwise be lost, enhancing the overall quality and usability of the footage. For instance, a drone surveying a construction site can now produce stabilized footage for easier progress tracking and quality control.
AI Stabilization in Virtual Reality (VR) and Augmented Reality (AR) Video Content, Exploring AI’s capabilities in video editing stabilization
The immersive nature of VR and AR experiences demands high-quality video content free from any jarring movements or distortions. AI video stabilization plays a crucial role in ensuring a smooth and comfortable viewing experience. Any instability in the video can cause motion sickness or disorientation in the user, diminishing the overall enjoyment and effectiveness of the VR/AR application.
AI stabilization algorithms help mitigate these issues by smoothing out camera movement and correcting any distortions, resulting in more realistic and immersive experiences. This is especially important in applications where user movement is a key component, such as VR gaming or AR training simulations. The ability to provide a stable visual perspective, even during dynamic movements, is essential for creating compelling and engaging VR/AR content.
Limitations and Challenges of AI Video Stabilization
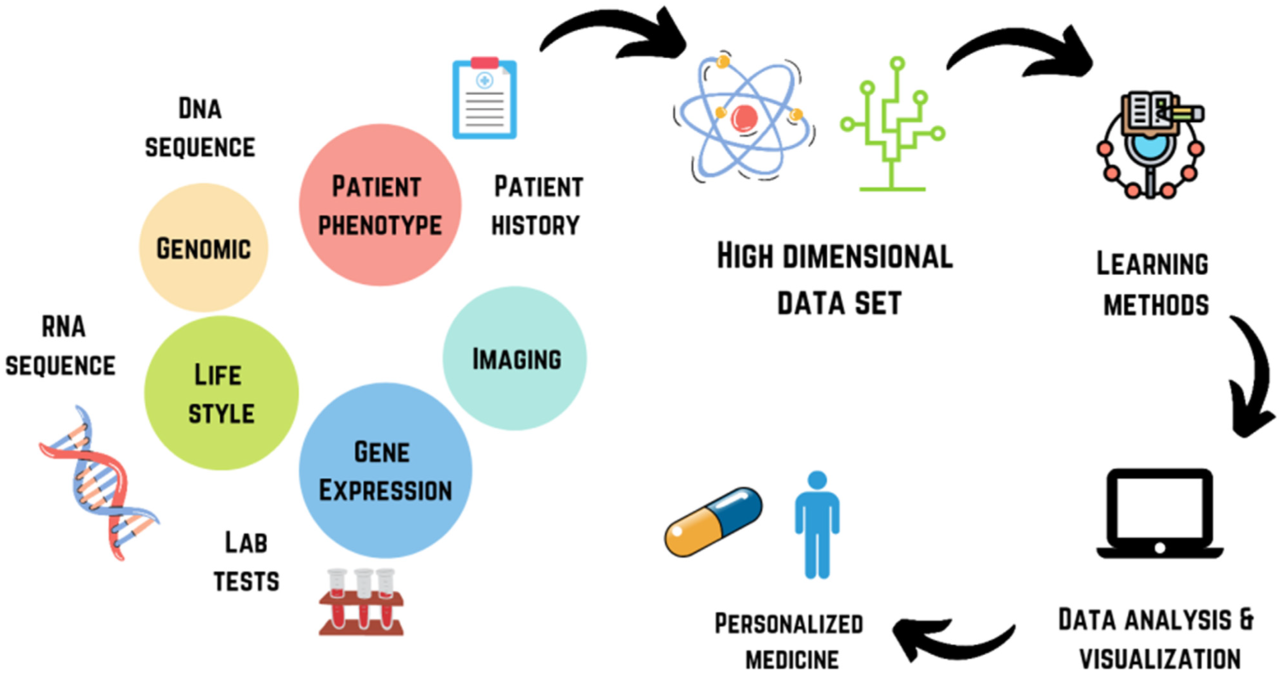
AI-powered video stabilization offers significant advancements in video editing, but it’s not without its limitations. Current technology faces hurdles in processing certain types of footage and requires substantial computational resources. Furthermore, inherent biases within the algorithms can lead to inaccuracies in the final stabilized video. Understanding these limitations is crucial for effectively utilizing AI stabilization tools and managing expectations.
Several factors currently restrict the capabilities of AI video stabilization. These limitations stem from the inherent complexities of analyzing and manipulating video data, particularly when dealing with challenging conditions. The effectiveness of AI stabilization is often directly related to the quality and characteristics of the input video.
Extreme Camera Movement and Low-Light Conditions
Handling extreme camera movements, such as rapid panning, tilting, or significant shaking, remains a challenge for AI stabilization algorithms. These movements often exceed the capabilities of the algorithms to accurately track stable points within the video frame, resulting in artifacts or distortions in the stabilized output. Similarly, low-light conditions present significant difficulties. The reduced amount of light impacts the image quality, making it harder for the AI to accurately identify and track features necessary for effective stabilization.
This often leads to blurry or noisy stabilized videos, even with advanced algorithms. For example, footage shot handheld at night with significant camera shake will likely yield less satisfactory results compared to footage shot in bright daylight with minimal movement.
Computational Cost and Resource Requirements
AI-based video stabilization is computationally intensive. The algorithms require significant processing power to analyze video frames, identify motion vectors, and generate stabilized output. This translates to higher computational costs, especially when processing high-resolution videos or long video clips. High-end hardware, such as powerful GPUs, is often necessary to achieve real-time or near real-time stabilization. The resource demands can be a significant barrier for users with limited computing resources, potentially hindering accessibility to this technology for individuals or smaller organizations.
For instance, processing a 4K video using advanced AI stabilization techniques might take several hours on a standard consumer-grade computer, while a high-end workstation might complete the same task in a fraction of the time.
Potential Biases and Inaccuracies
AI algorithms for video stabilization are trained on large datasets of video footage. If these datasets contain biases, such as overrepresentation of certain types of camera movements or scene content, the resulting algorithms may exhibit similar biases. This can lead to inaccuracies in stabilization, particularly for videos that deviate from the characteristics of the training data. For example, an algorithm trained primarily on videos of people walking might struggle to effectively stabilize footage of a rapidly moving vehicle.
Additionally, inaccuracies can arise from limitations in the algorithms’ ability to accurately interpret complex scenes or differentiate between relevant and irrelevant motion within the frame. This can result in unintended distortions or artifacts in the stabilized video.
Future Trends and Developments in AI Video Stabilization
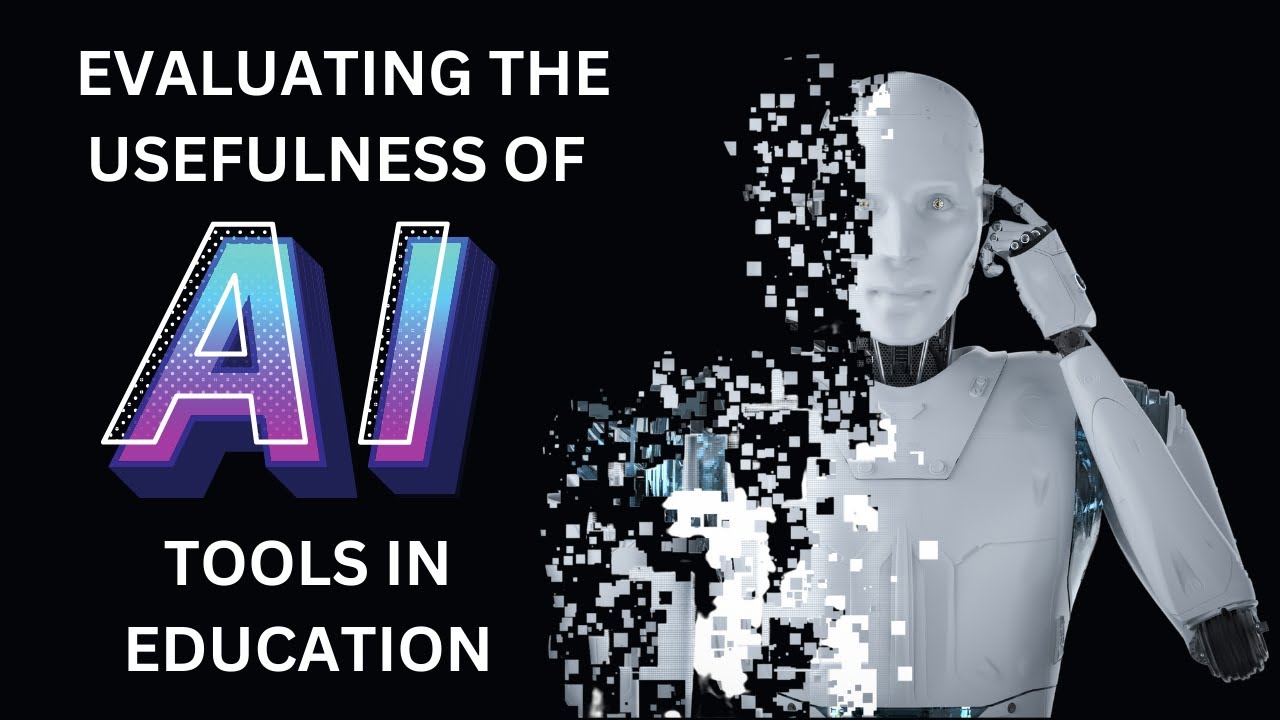
AI video stabilization is a rapidly evolving field, driven by advancements in machine learning and computer vision. Current techniques, while impressive, still face limitations in handling extreme motion or complex scenes. Future developments promise smoother, more efficient, and versatile video stabilization solutions, transforming the video editing landscape.The next generation of AI video stabilization will likely focus on increasing processing speed, improving accuracy, and expanding the range of scenarios it can effectively handle.
This will involve refining existing algorithms and exploring new approaches to tackle the challenges that remain.
Real-Time Stabilization and Complex Scene Handling
Real-time AI video stabilization, already emerging in some applications, will become increasingly prevalent. This capability will be crucial for live streaming, virtual reality, and augmented reality applications where immediate feedback is essential. Improvements will focus on reducing latency, enabling smooth, jitter-free video even with significant camera movement. For example, imagine live sports broadcasts where the camera operator needs to quickly pan and zoom, and the AI can instantly stabilize the footage without noticeable delay.
Handling complex scenes, such as those with rapid changes in lighting or significant parallax, will also see advancements. This will involve more sophisticated algorithms capable of identifying and compensating for multiple motion vectors within a single frame, leading to a more natural and realistic stabilized result. Think of a drone shot flying through a dense forest; current algorithms struggle, but future systems will likely achieve significantly better stabilization.
Integration with Other Video Editing Tools and Workflows
AI video stabilization will become seamlessly integrated into existing video editing software suites and workflows. This means that stabilization will no longer be a separate, standalone process, but rather an automated feature embedded within the editing process itself. For instance, a user could import footage, and the software would automatically apply stabilization as part of the initial import and analysis phase.
This integration will also extend to other AI-powered editing tools, such as AI-powered color grading and noise reduction. A hypothetical scenario could involve an AI that automatically analyzes footage, stabilizes it, color corrects it, and even removes unwanted objects or artifacts, all within a single, streamlined workflow. This would dramatically reduce the time and effort required for post-production.
Impact of Future Advancements on Video Production
The advancements in AI video stabilization will significantly impact video production across various sectors. Consider the scenario of a documentary filmmaker shooting footage in challenging conditions, like a bustling city street or a rough terrain. Currently, stabilizing this footage requires extensive manual work and specialized software, often resulting in a significant time investment. With future AI video stabilization, the filmmaker could shoot with greater freedom, knowing that the stabilization process will be largely automated and highly effective.
This would allow for greater creativity and flexibility in capturing footage, potentially leading to more dynamic and engaging documentaries. Furthermore, the cost of post-production would decrease significantly, making high-quality video production more accessible to independent filmmakers and smaller production houses. The increased efficiency would also benefit professional studios, allowing them to handle larger volumes of footage and meet tighter deadlines.
Illustrative Examples of AI Stabilization in Action
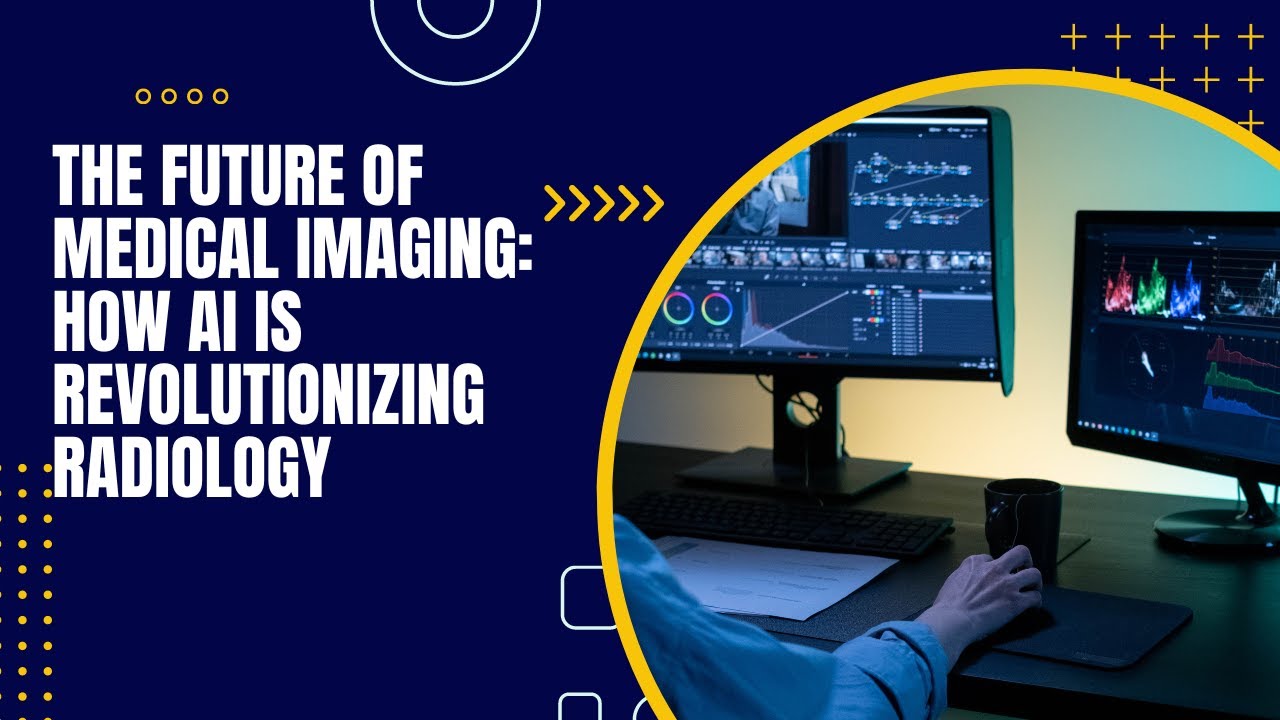
AI video stabilization has revolutionized the way we handle shaky footage, transforming amateur recordings into professional-looking videos. The algorithms behind this technology are sophisticated, capable of handling various challenges that traditional methods struggle with. Below are three examples illustrating the power of AI in stabilizing video footage under different conditions.
-
Shaky Handheld Footage: A Tourist’s Adventure
This example features a video clip recorded by a tourist using a handheld smartphone camera while walking through a bustling marketplace. The original footage exhibits significant shake and jitter, making it difficult to follow the action. The camera movement is erratic, with both vertical and horizontal instability. Applying AI stabilization significantly reduces the shake, resulting in a smoother, more watchable video.
- Before: The original footage is highly unstable, with noticeable blurring and jumpiness, making it difficult to focus on the subject matter.
- After: The stabilized video is remarkably smoother. The camera movement is significantly reduced, allowing viewers to easily follow the action and appreciate the details of the marketplace. The improvement is evident in the reduced blur and the overall clarity of the scene. Key improvements include a substantial reduction in camera shake, improved image sharpness, and a more professional look.
-
Extreme Camera Movement: Action Sports Highlights
The second example showcases a video clip of an extreme sports event, such as a mountain bike race down a steep slope. The original footage is characterized by extreme camera movement, including rapid pans, tilts, and sudden jolts. Traditional stabilization techniques often fail with this level of instability, resulting in artifacts or unnatural smoothing. However, advanced AI stabilization algorithms can effectively compensate for this aggressive camera movement, producing a surprisingly stable final product.
- Before: The original video is incredibly shaky, with rapid and unpredictable camera movements that make it nearly impossible to follow the action. The viewer experiences motion sickness due to the extreme camera instability.
- After: AI stabilization dramatically reduces the extreme camera movement, providing a much smoother and more watchable video. While some minor residual movement might remain, the overall improvement in stability is remarkable, allowing viewers to fully appreciate the speed and skill of the athlete. Key improvements include drastically reduced motion sickness, improved clarity of action, and better visual tracking of the subject.
-
Low-Resolution Video: Archival Footage Enhancement
The third example involves a low-resolution video clip, possibly archival footage from an older film or videotape. The original footage suffers from both low resolution and significant shake. AI stabilization, in this case, plays a dual role. It not only stabilizes the video but also enhances its visual quality by interpolating missing details and reducing noise.
The results are particularly impressive, transforming a grainy, unstable clip into a relatively clearer and smoother version.
- Before: The original footage is grainy, blurry, and highly unstable, making it difficult to discern details and follow the action. The low resolution significantly limits its usability.
- After: AI stabilization significantly reduces the shake, improving the overall clarity of the footage. While the low resolution remains a factor, the stabilization dramatically improves watchability. The improved stability allows for a more focused viewing experience, making the historical content more accessible. Key improvements include a noticeable reduction in shake, enhanced visual clarity despite low resolution, and improved overall watchability.
Conclusion: Exploring AI’s Capabilities In Video Editing Stabilization
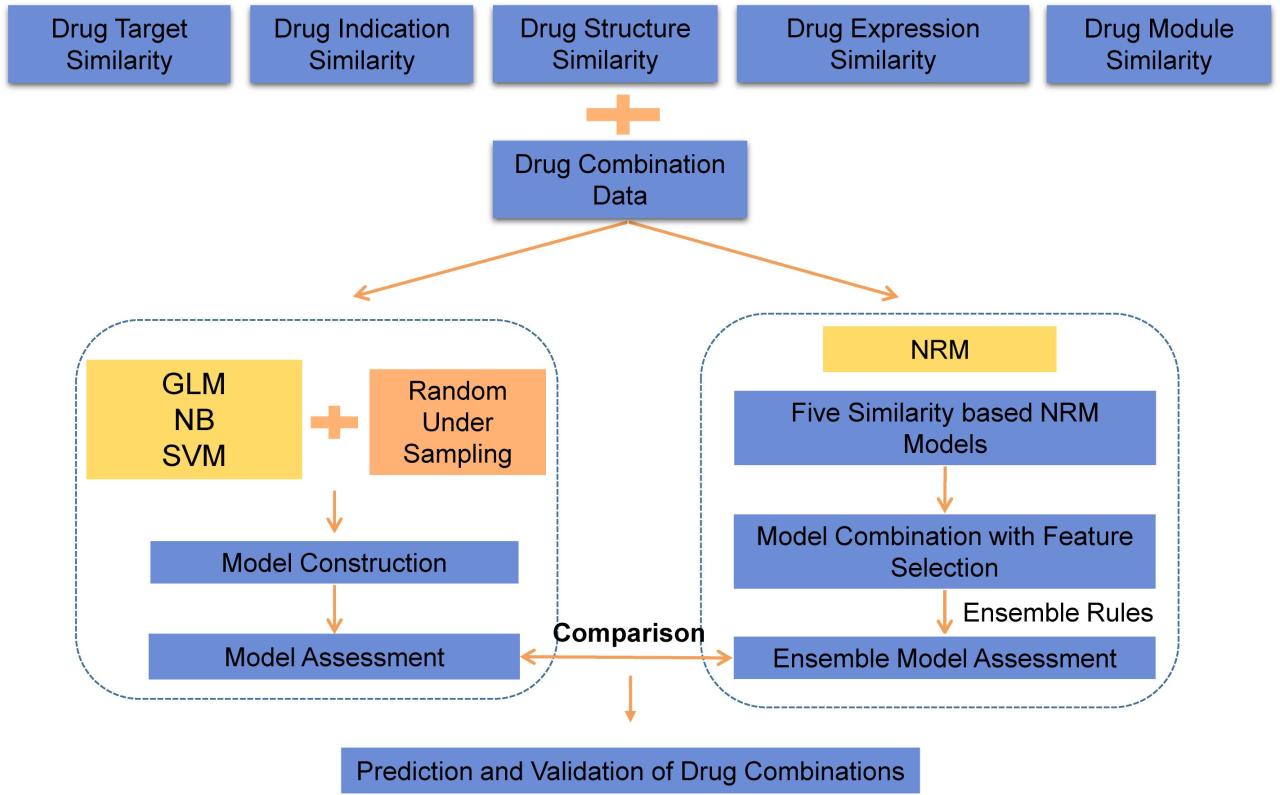
AI-powered video stabilization represents a significant leap forward in video editing technology. While current limitations exist, the rapid advancements in AI suggest a future where seamless, high-quality stabilization is achievable for everyone, regardless of skill level or hardware constraints. The integration of AI stabilization into broader video workflows promises to further streamline the editing process, freeing up creators to focus on the creative aspects of their work.
The potential for real-time stabilization and enhanced handling of complex scenes hints at an exciting evolution in video production.