Future trends of AI and big data in the business world are reshaping industries at an unprecedented pace. From automating complex processes to predicting market shifts with startling accuracy, these technologies are no longer futuristic concepts; they’re driving today’s business decisions and shaping tomorrow’s competitive landscape. This exploration delves into the transformative impact of AI and big data across various sectors, examining both the immense potential and the critical challenges they present.
We’ll investigate how AI-powered automation is streamlining operations, the role of big data analytics in enhancing business intelligence, and the ethical considerations that must guide their implementation. We’ll also explore the evolving relationship between AI, big data, and the future workforce, considering the skills needed to thrive in this rapidly changing environment. This analysis provides a comprehensive overview, equipping readers with the knowledge to navigate the evolving world of AI and big data in business.
AI-Driven Automation in Business Processes: Future Trends Of AI And Big Data In The Business World
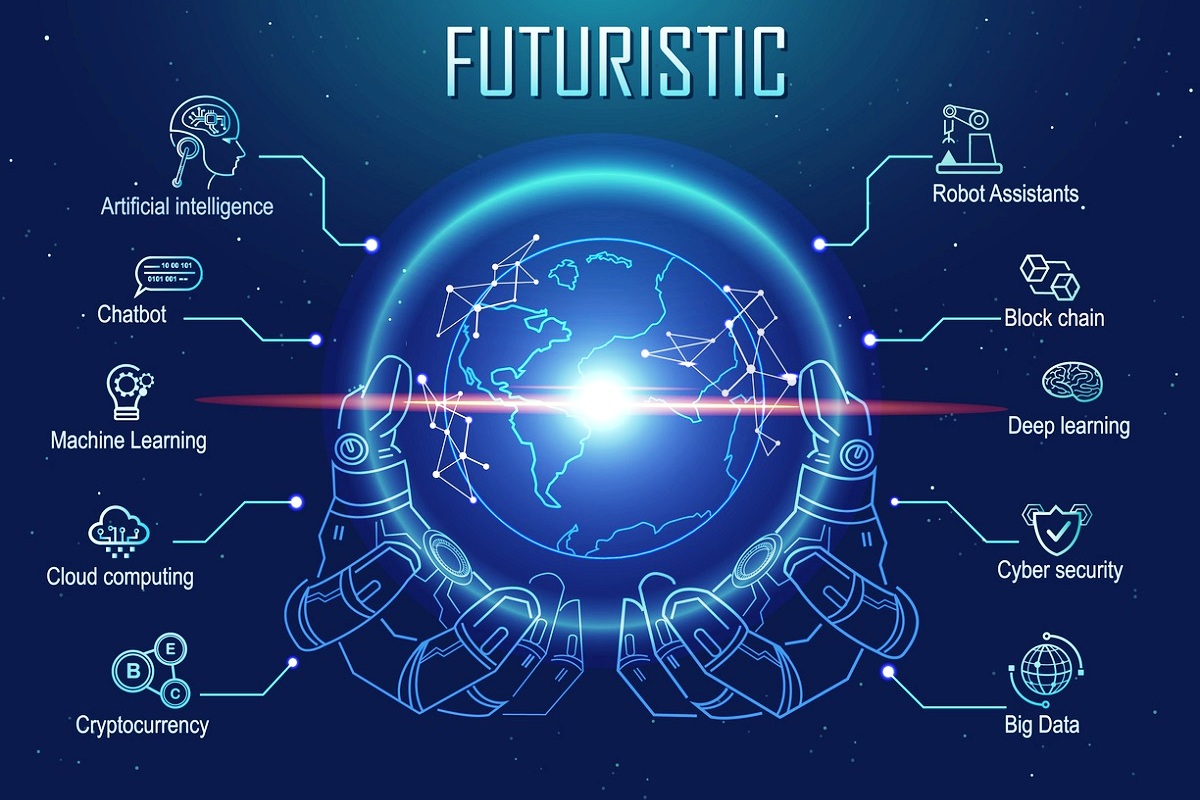
AI-driven automation is rapidly transforming business operations across diverse sectors, streamlining tasks, improving efficiency, and unlocking new levels of productivity. This transformation leverages machine learning, natural language processing, and robotic process automation (RPA) to automate previously manual or semi-manual processes, resulting in significant cost savings and enhanced operational agility.AI’s impact on streamlining operational tasks is profound. By automating repetitive, rule-based processes, businesses can free up human employees to focus on more strategic and creative endeavors.
This leads to increased employee satisfaction and a more engaged workforce. Furthermore, AI can process vast amounts of data far more quickly and accurately than humans, leading to faster decision-making and improved operational outcomes. This increased speed and accuracy extends across various departments, from finance and human resources to marketing and sales.
AI-Powered Automation Tools in Supply Chain Management and Customer Service
AI-powered automation tools are revolutionizing supply chain management and customer service. In supply chain management, AI algorithms optimize logistics, predict demand, and manage inventory more effectively. For example, AI-powered systems can analyze historical sales data, weather patterns, and economic indicators to forecast future demand with greater accuracy, minimizing stockouts and reducing warehousing costs. Similarly, in customer service, AI-powered chatbots provide instant support, answering frequently asked questions and resolving simple issues, freeing up human agents to handle more complex queries.
Tools like Amazon’s fulfillment network leverage AI extensively for inventory management and route optimization, showcasing the impact on efficiency and cost reduction. Customer service platforms frequently use AI-powered sentiment analysis to gauge customer satisfaction and identify areas for improvement.
Challenges of Implementing AI-Driven Automation and Strategies for Overcoming Them, Future trends of AI and big data in the business world
Implementing AI-driven automation presents several challenges. Data quality is paramount; AI algorithms require large, clean, and accurately labeled datasets to function effectively. Insufficient data or poor data quality can lead to inaccurate predictions and flawed automation. Furthermore, integrating AI systems with existing legacy systems can be complex and costly. Finally, concerns about job displacement and the need for employee retraining must be addressed proactively.Overcoming these challenges requires a phased approach.
Start with pilot projects to test the feasibility and effectiveness of AI solutions in specific areas. Invest in data quality initiatives to ensure the accuracy and reliability of the data used to train AI models. Partner with experienced AI vendors to navigate the complexities of integration and implementation. And finally, implement robust employee training and upskilling programs to prepare the workforce for the changing job landscape.
A holistic approach, encompassing technology, data, and people, is crucial for successful AI-driven automation.
Cost-Effectiveness of Traditional Methods vs. AI-Driven Automation
The following table compares the cost-effectiveness of traditional methods versus AI-driven automation across various aspects. Note that the specific figures will vary depending on the industry, specific application, and scale of implementation.
Aspect | Traditional Methods | AI-Driven Automation | Cost Comparison |
---|---|---|---|
Initial Investment | Lower | Higher (includes software, hardware, and implementation) | AI initially more expensive |
Operational Costs | Higher (labor, errors) | Lower (reduced labor, fewer errors) | AI lower over time |
Speed and Efficiency | Slower, prone to human error | Faster, more accurate | AI significantly faster and more efficient |
Scalability | Limited | Highly scalable | AI offers greater scalability |
Big Data Analytics for Business Intelligence
Big data analytics transforms raw data into actionable insights, significantly improving a business’s ability to understand its market, customers, and internal operations. This enhanced understanding directly fuels more effective decision-making across all levels of an organization, leading to improved efficiency, profitability, and competitive advantage. The sheer volume, velocity, and variety of data available today make sophisticated analytics essential for navigating the complexities of the modern business landscape.Big data analytics enhances decision-making by providing a more comprehensive and nuanced view of business realities.
Instead of relying on limited, potentially biased samples, businesses can leverage vast datasets to identify trends, patterns, and anomalies that would otherwise remain hidden. This granular level of insight allows for more accurate forecasting, risk assessment, and resource allocation, leading to more informed and strategic choices. For example, predicting customer churn with high accuracy allows for proactive intervention and retention strategies, while identifying supply chain bottlenecks enables optimization and cost savings.
Real-World Examples of Big Data Application in Market Analysis and Customer Segmentation
Netflix’s success is largely attributed to its sophisticated use of big data analytics. By analyzing viewing habits, preferences, and ratings of millions of users, Netflix generates personalized recommendations, significantly improving user engagement and retention. This data-driven approach allows them to optimize content creation and acquisition strategies, ensuring they invest in projects with the highest probability of success. Similarly, Amazon utilizes vast amounts of customer data to personalize product recommendations, optimize pricing strategies, and target marketing campaigns with unparalleled accuracy.
Their recommendation engine, powered by big data, is a key driver of their e-commerce dominance.
Data Mining and Predictive Modeling Techniques in Business
Data mining employs various techniques to uncover hidden patterns and relationships within large datasets. Common methods include association rule mining (identifying products frequently purchased together), clustering (grouping similar customers or products), and classification (predicting customer behavior based on past actions). Predictive modeling, often utilizing machine learning algorithms such as regression and classification models, builds mathematical models to forecast future outcomes.
For instance, a retailer might use predictive modeling to forecast sales based on historical data, seasonality, and promotional campaigns. These models are constantly refined and improved through iterative learning, leveraging the ever-growing volume of data. For example, logistic regression can predict the probability of a customer churning, while time series analysis can forecast future demand for a particular product.
Ethical Considerations of Big Data for Business Intelligence
The use of big data for business intelligence presents several ethical considerations that require careful attention.
- Data Privacy and Security: Protecting customer data from unauthorized access and misuse is paramount. Robust security measures and compliance with relevant data privacy regulations (e.g., GDPR, CCPA) are crucial.
- Bias and Discrimination: Algorithms trained on biased data can perpetuate and amplify existing societal inequalities. It is essential to ensure fairness and mitigate bias in data collection, processing, and model development.
- Transparency and Explainability: Understanding how big data analytics models arrive at their conclusions is important for building trust and accountability. Techniques for enhancing model transparency and explainability are vital.
- Data Ownership and Consent: Clearly defining data ownership and obtaining informed consent from individuals before collecting and using their data are essential ethical considerations.
- Data Security Breaches and Their Consequences: Robust security protocols are needed to prevent data breaches, which can have severe financial and reputational consequences for businesses.
The Rise of AI in Customer Relationship Management (CRM)
AI is rapidly transforming customer relationship management, moving beyond basic data storage to deliver personalized experiences and drive significant business growth. The integration of artificial intelligence is enabling businesses to understand customer behavior more deeply, anticipate needs, and proactively address concerns, ultimately fostering stronger customer relationships and increased loyalty. This shift is driven by the increasing availability of data and the sophistication of AI algorithms capable of processing and interpreting this information effectively.
AI’s role in personalizing customer experiences is multifaceted. By analyzing vast datasets encompassing customer interactions, purchase history, website activity, and social media engagement, AI algorithms can create detailed customer profiles. This granular understanding allows businesses to tailor marketing campaigns, product recommendations, and customer service interactions to individual preferences, leading to higher engagement and conversion rates. For example, an e-commerce platform might use AI to suggest products based on a customer’s past purchases and browsing history, while a financial institution could leverage AI to personalize investment advice based on individual risk tolerance and financial goals.
AI-Powered CRM Tools and Their Applications
AI-powered CRM tools are enhancing customer service interactions and lead generation through various functionalities. These tools automate repetitive tasks, freeing up human agents to focus on complex issues requiring empathy and nuanced understanding. Simultaneously, AI enhances the efficiency and effectiveness of customer service interactions.
For instance, AI-powered chatbots provide instant support, answering frequently asked questions and resolving simple issues 24/7. Predictive analytics tools identify potential churn risks, allowing businesses to proactively engage at-risk customers and prevent loss. Lead scoring algorithms prioritize leads based on their likelihood of conversion, helping sales teams focus their efforts on the most promising prospects. Examples of such tools include Salesforce Einstein, Microsoft Dynamics 365 AI, and HubSpot’s AI-powered features.
Data Privacy and Security Concerns in AI-Driven CRM
The use of AI in CRM presents both significant benefits and potential risks, particularly concerning data privacy and security. The collection and analysis of vast amounts of customer data necessitates robust security measures to prevent unauthorized access and data breaches. Compliance with data privacy regulations, such as GDPR and CCPA, is crucial. Furthermore, the use of AI algorithms raises concerns about algorithmic bias and the potential for discriminatory outcomes.
Transparency in AI decision-making processes is essential to build and maintain customer trust.
Mitigating these risks requires a multi-pronged approach. This includes implementing strong data encryption and access control mechanisms, regularly auditing data security practices, and ensuring algorithmic fairness and transparency. Investing in employee training on data privacy and security best practices is also crucial. Building trust requires clear communication with customers about how their data is being used and providing mechanisms for them to access and control their personal information.
Comparison of AI-Powered CRM Platforms
Platform | Key Features | Strengths |
---|---|---|
Salesforce Einstein | Predictive analytics, lead scoring, intelligent chatbots, automated workflows. | Extensive ecosystem, robust analytics, strong integration capabilities. |
Microsoft Dynamics 365 AI | Customer insights, sales forecasting, intelligent assistance for customer service, automated email responses. | Seamless integration with other Microsoft products, strong focus on business process automation. |
HubSpot CRM with AI | Lead scoring, sales forecasting, chatbot functionality, email scheduling and automation. | User-friendly interface, cost-effective option for small to medium-sized businesses, strong marketing automation capabilities. |
Predictive Analytics and Forecasting in Business
Predictive analytics leverages historical data, statistical algorithms, and machine learning techniques to identify the likelihood of future outcomes. This allows businesses to move beyond reactive decision-making and instead anticipate trends, proactively mitigate risks, and capitalize on opportunities. By understanding potential future scenarios, organizations can optimize resource allocation, improve operational efficiency, and gain a significant competitive advantage.Predictive analytics empowers businesses to anticipate future trends and make proactive decisions by analyzing historical data to identify patterns and relationships.
This enables informed predictions about future events, allowing businesses to adjust strategies and operations accordingly. For example, predicting customer churn allows a company to implement targeted retention strategies, while forecasting sales helps optimize inventory management and resource allocation. The accuracy of these predictions depends heavily on the quality and quantity of data used, as well as the sophistication of the analytical models employed.
Predictive Modeling for Sales Forecasting and Risk Management
Companies across various sectors utilize predictive modeling to enhance sales forecasting and mitigate potential risks. For instance, a retail company might use historical sales data, weather patterns, and marketing campaign performance to predict future sales for specific product lines. This enables optimized inventory management, preventing stockouts or overstocking. In the financial sector, predictive models analyze credit scores, transaction history, and market trends to assess the risk of loan defaults, enabling more informed lending decisions and reducing potential losses.
A successful example is Netflix, which uses predictive analytics to personalize recommendations and forecast content popularity, directly impacting its programming decisions and subscriber retention.
Statistical Methods and Machine Learning Algorithms in Predictive Analytics
A variety of statistical methods and machine learning algorithms are employed in predictive analytics, each with its own strengths and weaknesses. Common statistical techniques include regression analysis (linear, logistic, polynomial), time series analysis (ARIMA, exponential smoothing), and survival analysis (Kaplan-Meier, Cox proportional hazards). These methods are particularly useful for identifying relationships between variables and making predictions based on historical data.
Machine learning algorithms, such as decision trees, random forests, support vector machines (SVMs), and neural networks, are more complex and can handle large datasets with numerous variables. They excel at identifying non-linear relationships and patterns that might be missed by traditional statistical methods. The choice of method depends on the specific business problem, the nature of the data, and the desired level of accuracy.
Building a Predictive Model: A Step-by-Step Process
The process of building a predictive model typically involves several key steps. A well-defined process ensures the model is accurate, reliable, and addresses the specific business problem. The flowchart would visually represent the following steps:
1. Define the Business Problem
Clearly articulate the specific question the model aims to answer (e.g., predict customer churn, forecast sales).
2. Data Collection and Preparation
Gather relevant data from various sources, clean it, handle missing values, and transform it into a suitable format for modeling.
3. Feature Engineering
Select and engineer relevant variables (features) that are likely to be predictive of the target variable. This may involve creating new variables from existing ones or transforming variables to improve model performance.
4. Model Selection
Choose an appropriate statistical method or machine learning algorithm based on the nature of the data and the business problem.
5. Model Training and Evaluation
Train the chosen model using a portion of the data and evaluate its performance using appropriate metrics (e.g., accuracy, precision, recall, AUC). This step often involves techniques like cross-validation to ensure the model generalizes well to unseen data.
6. Model Deployment and Monitoring
Deploy the trained model to make predictions on new data and continuously monitor its performance to ensure it remains accurate and reliable over time. Regular retraining may be necessary to account for changes in the underlying data patterns.
AI and Big Data in Cybersecurity
The convergence of artificial intelligence (AI) and big data is revolutionizing cybersecurity, offering unprecedented capabilities to detect, prevent, and respond to increasingly sophisticated cyber threats. The sheer volume and velocity of data generated in today’s digital landscape makes manual threat detection nearly impossible. AI algorithms, however, can sift through this data, identifying patterns and anomalies indicative of malicious activity that would be missed by human analysts.
This proactive approach, coupled with the ability to automate responses, is crucial in mitigating the risk of data breaches and other cyberattacks.AI and big data are fundamentally changing how organizations approach cybersecurity. Big data provides the raw material – the massive datasets containing network traffic, user activity, and security logs – while AI provides the analytical engine to process and interpret this data, identifying threats in real-time and enabling rapid response.
This symbiotic relationship is transforming cybersecurity from a reactive to a proactive discipline.
AI-Powered Security Tools and Their Applications
Several AI-powered security tools are already deployed by organizations to enhance their cybersecurity posture. These tools leverage machine learning (ML) and deep learning (DL) algorithms to analyze vast datasets and identify malicious patterns. For example, intrusion detection systems (IDS) utilizing AI can analyze network traffic to identify suspicious activities, such as unauthorized access attempts or malware infections. Similarly, security information and event management (SIEM) systems integrated with AI can correlate security events from diverse sources, providing a comprehensive view of the organization’s security posture and enabling faster incident response.
Furthermore, AI-powered endpoint detection and response (EDR) solutions monitor endpoints for malicious behavior, enabling rapid detection and containment of threats. These tools are crucial for effective threat hunting and incident response.
Challenges in Implementing AI-Based Cybersecurity Solutions
While AI offers significant advantages in cybersecurity, its implementation also presents several challenges. One significant concern is the potential for adversarial attacks. Attackers can manipulate data or algorithms to evade detection, creating a constant arms race between security professionals and malicious actors. For instance, attackers might craft sophisticated malware that can adapt to AI-based detection mechanisms, making it difficult for security systems to identify and neutralize the threat.
Another challenge lies in the complexity of implementing and managing AI-based security solutions. These systems often require specialized expertise and significant computational resources, making them inaccessible to smaller organizations. Furthermore, the explainability of AI algorithms can be a concern. Understanding why an AI system flagged a particular event as malicious is crucial for building trust and ensuring effective incident response.
The lack of transparency in some AI algorithms can hinder this process.
Best Practices for Securing Data in the Age of AI and Big Data
Implementing robust cybersecurity measures is crucial in the era of AI and big data. The following best practices are essential for organizations to protect their data and systems:
- Implement a layered security approach: Employ multiple security layers, including firewalls, intrusion detection systems, and endpoint protection, to create a robust defense against cyberattacks. This layered approach mitigates the impact of successful attacks by preventing further compromise.
- Regularly update security software and systems: Keeping software and systems up-to-date is crucial to patching vulnerabilities and preventing attackers from exploiting known weaknesses. This includes operating systems, applications, and security tools.
- Employ strong authentication and access control measures: Implement strong passwords, multi-factor authentication, and robust access control mechanisms to limit access to sensitive data and systems. This minimizes the potential impact of compromised credentials.
- Invest in employee security awareness training: Educate employees about common cyber threats and best practices to prevent phishing attacks, malware infections, and other social engineering techniques. Human error remains a significant vulnerability.
- Regularly monitor and analyze security logs: Continuously monitor security logs for suspicious activity and use AI-powered tools to analyze these logs for patterns and anomalies that might indicate a security breach. Proactive monitoring is crucial for early threat detection.
- Develop and test incident response plans: Create and regularly test incident response plans to ensure a coordinated and effective response to security incidents. This plan should Artikel steps to contain, eradicate, and recover from attacks.
- Embrace data loss prevention (DLP) strategies: Implement DLP solutions to monitor and prevent sensitive data from leaving the organization’s control. This is crucial for protecting confidential information.
The Impact of AI on the Future Workforce
The integration of artificial intelligence (AI) and automation into business processes is fundamentally reshaping the future workforce. This transformation necessitates a proactive approach to adapting skills, creating new roles, and mitigating potential negative impacts on employment. Understanding these changes is crucial for businesses and individuals alike to navigate the evolving job market successfully.AI and automation will significantly alter job roles and the skills required, leading to both displacement and creation of new opportunities.
Routine and repetitive tasks are increasingly automated, impacting roles in manufacturing, data entry, and customer service. However, this shift also generates demand for professionals with skills to manage, maintain, and develop these AI systems. The demand for individuals skilled in data science, AI development, and AI ethics will continue to grow.
Transformation of Job Roles and Required Skills
The impact of AI on existing job roles is multifaceted. While some roles will be automated entirely, many others will be augmented, requiring employees to adapt and acquire new skills. For instance, accountants will need to master data analytics tools to interpret AI-generated insights, while customer service representatives will need to leverage AI-powered chatbots effectively and handle more complex customer interactions.
The shift necessitates a focus on critical thinking, problem-solving, creativity, and emotional intelligence – skills that are less easily automated. These human-centric skills will become increasingly valuable in the AI-driven workplace.
New Job Roles Created by AI and Big Data Technologies
The rise of AI and big data has already spurred the creation of numerous new job roles. Examples include AI ethicists, who are responsible for ensuring the ethical development and deployment of AI systems; data scientists, who extract insights from vast datasets; machine learning engineers, who build and maintain AI models; and AI trainers, who teach AI systems to perform specific tasks.
Furthermore, roles focused on AI-driven cybersecurity and the management of AI-powered systems are becoming increasingly prevalent. These new roles often require advanced technical skills and a deep understanding of AI principles.
Strategies for Workforce Reskilling and Upskilling
Addressing the skills gap created by AI-driven automation requires proactive strategies for workforce reskilling and upskilling. Businesses can invest in training programs that equip employees with the skills needed to work alongside AI, such as data analysis, programming, and AI literacy. Governments can play a crucial role by funding educational initiatives and promoting lifelong learning opportunities. Individual employees should also take the initiative to acquire new skills through online courses, boot camps, and professional development programs.
A collaborative approach involving businesses, governments, and individuals is essential to ensure a smooth transition to the AI-driven economy.
Potential Effects of AI on Employee Productivity and Job Satisfaction
AI has the potential to significantly enhance employee productivity by automating tedious tasks and freeing up time for more strategic work. For example, AI-powered tools can automate data entry, allowing administrative staff to focus on more complex tasks that require human judgment and creativity. However, the impact of AI on job satisfaction is more complex. While AI can reduce workload and improve efficiency, it can also lead to job displacement and feelings of anxiety about job security.
Companies need to implement strategies to mitigate these negative impacts, such as providing clear communication about the role of AI in the workplace, investing in employee training and development, and fostering a culture of collaboration between humans and AI systems. For instance, a company successfully implementing AI to automate routine tasks might see increased job satisfaction among employees who now have more time to focus on creative problem-solving and strategic planning, leading to improved morale and overall productivity.
Ethical Considerations of AI and Big Data in Business
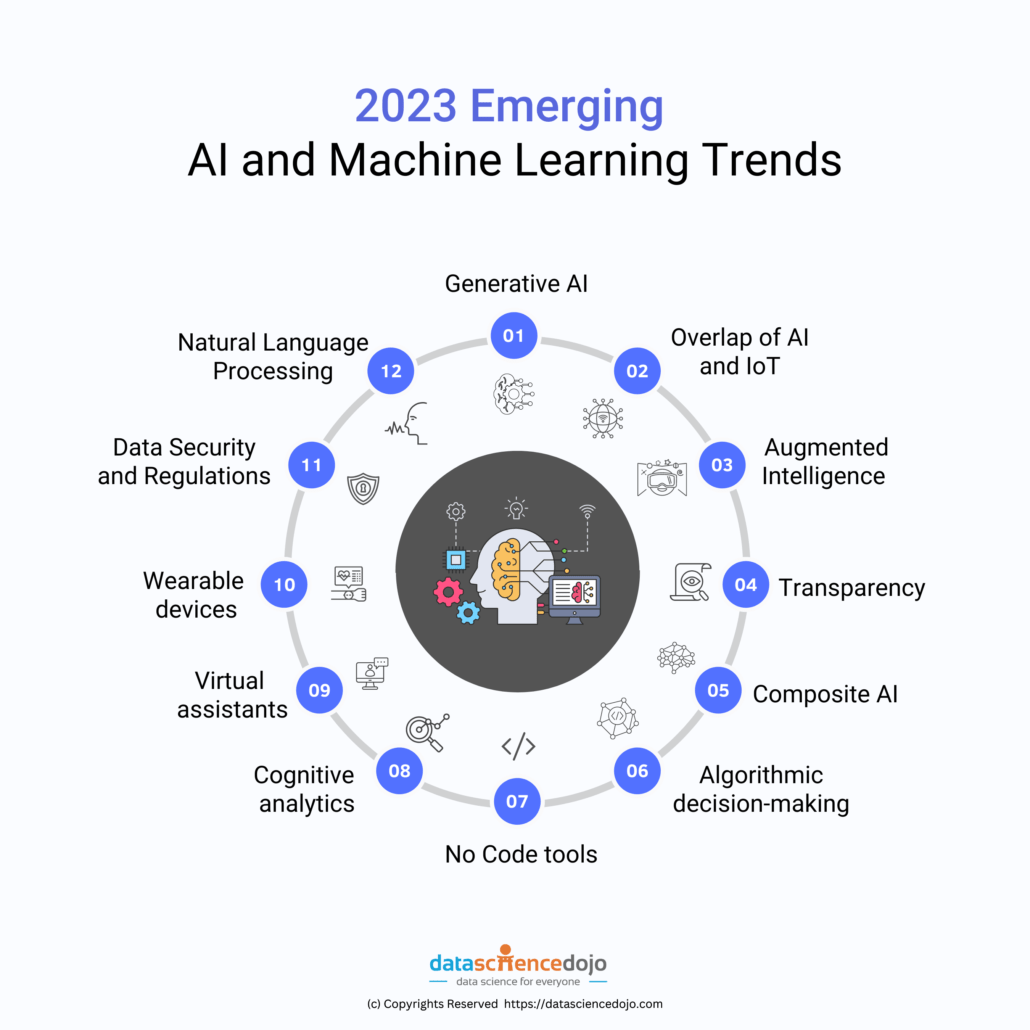
The increasing integration of artificial intelligence (AI) and big data into business operations presents significant ethical challenges. The potential for bias, lack of transparency, and breaches of privacy necessitates a proactive approach to responsible development and deployment. Addressing these ethical concerns is crucial not only for maintaining public trust but also for ensuring the long-term sustainability and success of AI-driven businesses.
Bias and Fairness in AI Algorithms
AI algorithms are trained on data, and if that data reflects existing societal biases, the resulting AI system will likely perpetuate and even amplify those biases. For example, facial recognition technology has been shown to be less accurate in identifying individuals with darker skin tones, leading to potential misidentification and unfair consequences in law enforcement and security applications. This highlights the critical need for careful data curation and algorithm design to mitigate bias and ensure fairness in AI-driven decision-making processes.
Addressing this requires a multi-faceted approach, including diverse datasets, algorithmic auditing, and ongoing monitoring for bias.
Data Privacy and Security
The collection and use of vast amounts of data inherent in big data analytics and AI applications raise serious privacy concerns. Businesses must ensure compliance with data protection regulations like GDPR and CCPA, which mandate transparency and user consent for data collection and processing. Data breaches, whether due to hacking or internal vulnerabilities, can have severe consequences, including financial losses, reputational damage, and legal liabilities.
Robust security measures, including encryption, access controls, and regular security audits, are essential to protect sensitive data. Furthermore, implementing privacy-enhancing technologies, such as differential privacy, can help balance data utility with individual privacy.
Transparency and Explainability in Algorithmic Decision-Making
Many AI systems, particularly deep learning models, operate as “black boxes,” making it difficult to understand how they arrive at their decisions. This lack of transparency can erode trust and make it challenging to identify and rectify errors or biases. The increasing use of AI in high-stakes decisions, such as loan applications or hiring processes, necessitates greater explainability. Techniques like LIME (Local Interpretable Model-agnostic Explanations) and SHAP (SHapley Additive exPlanations) are being developed to provide insights into the decision-making processes of complex AI models, enhancing transparency and accountability.
Establishing Ethical Guidelines and Regulations
The rapid advancement of AI and big data necessitates the development of clear ethical guidelines and regulations. These frameworks should address issues such as data privacy, algorithmic bias, transparency, and accountability. Collaboration between industry stakeholders, policymakers, and ethicists is crucial in shaping responsible AI development and deployment. Existing regulations need to be updated to address the unique challenges posed by AI, and new regulations may be necessary to cover emerging technologies and applications.
Self-regulation within industries can play a significant role, but government oversight is necessary to ensure consistent standards and accountability.
Best Practices for Responsible AI and Big Data Use
Implementing a robust ethical framework requires a multi-pronged approach. This includes establishing clear data governance policies, incorporating ethical considerations into the AI development lifecycle, conducting regular audits to assess bias and fairness, and providing ongoing training to employees on ethical AI principles. Prioritizing user privacy, ensuring data security, and promoting transparency in algorithmic decision-making are crucial elements of responsible AI and big data use.
Establishing independent oversight bodies to monitor and enforce ethical standards can further enhance accountability and build public trust. Regularly reviewing and updating these practices is also essential to adapt to the evolving landscape of AI and big data technologies.
Epilogue
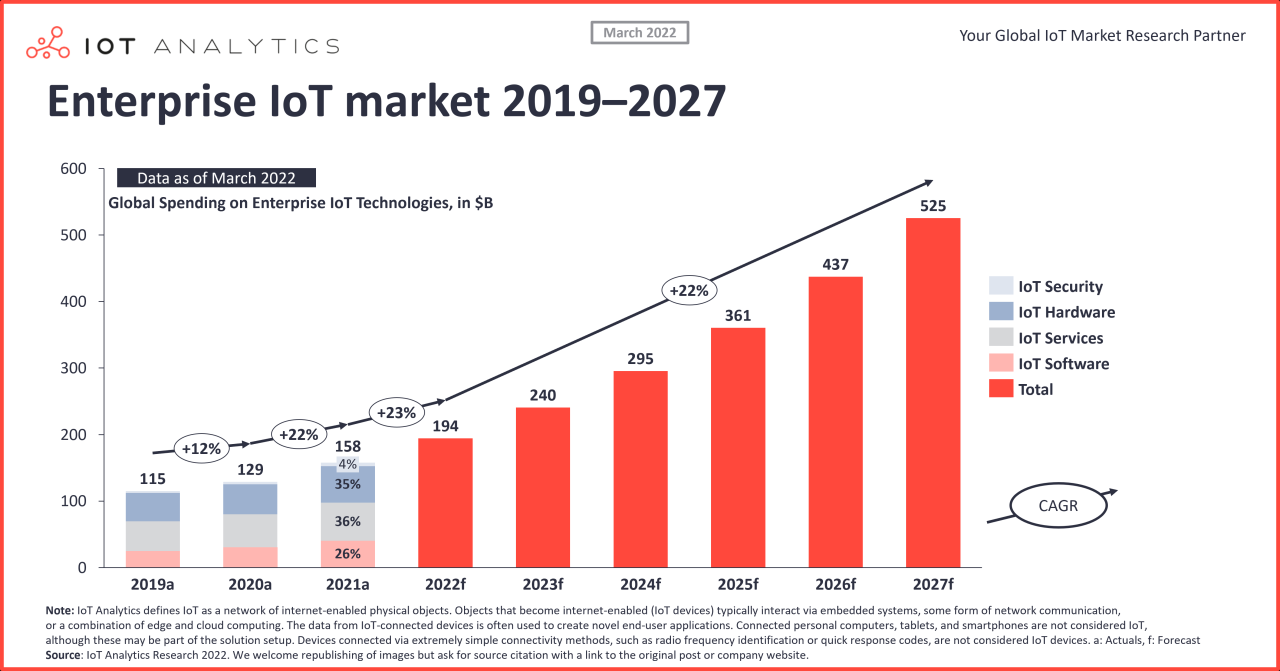
The integration of AI and big data is undeniably transforming the business world, presenting both immense opportunities and significant challenges. While AI-driven automation promises increased efficiency and predictive analytics offer strategic advantages, ethical considerations surrounding data privacy, algorithmic bias, and workforce displacement demand careful attention. Successfully navigating this technological revolution requires a proactive approach, embracing innovation while simultaneously addressing the ethical implications to ensure responsible and sustainable growth.
The future of business hinges on a strategic understanding and responsible implementation of these powerful technologies.