How AI algorithms shape our online experiences and daily choices is no longer a futuristic fantasy; it’s the present reality. From the videos we watch on YouTube to the products we buy online, artificial intelligence subtly yet powerfully influences our decisions. This pervasive influence extends beyond entertainment, impacting our social interactions, news consumption, and even our daily routines. Understanding how these algorithms function, their ethical implications, and their potential for both benefit and harm is crucial in navigating the increasingly AI-driven world we inhabit.
This exploration delves into the intricate ways AI algorithms personalize our online experiences, examining recommendation systems, targeted advertising, and their impact on social media. We’ll uncover how these algorithms collect and utilize our data, shaping our perceptions and influencing the information we access. Further, we’ll investigate the ethical considerations surrounding AI’s role in our daily choices, from smart home devices to navigation apps, and discuss strategies for mitigating potential negative consequences and promoting responsible AI development.
AI Algorithms in Personalized Content Delivery
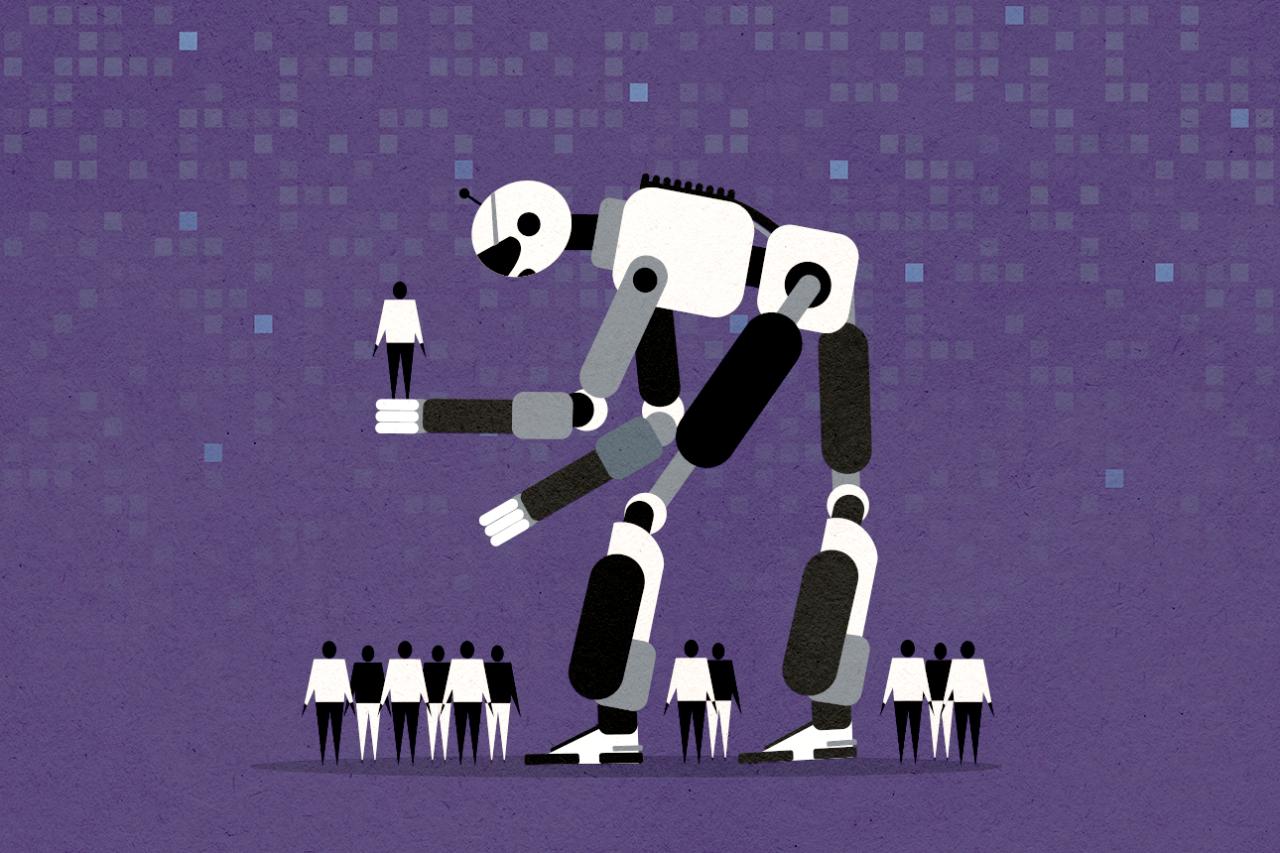
The rise of artificial intelligence has profoundly reshaped how we consume online content. AI algorithms, particularly recommendation systems, are central to this transformation, tailoring our digital experiences to an unprecedented degree. These systems analyze vast amounts of user data to predict preferences and deliver personalized content feeds across various platforms, influencing everything from the videos we watch to the news we read and the products we buy.
This personalization, while offering convenience and tailored experiences, also raises important questions about algorithmic bias, filter bubbles, and the potential erosion of diverse perspectives.
Recommendation systems utilize sophisticated algorithms to analyze user data and predict future preferences. This data encompasses a wide range of information, including viewing history, search queries, likes, shares, comments, and even the time spent interacting with specific content. On platforms like YouTube, Netflix, and social media sites, these algorithms continuously learn and adapt, refining their predictions based on user interactions.
For example, YouTube’s algorithm considers factors like watch time, click-through rates, and engagement with similar videos to suggest relevant content. Netflix leverages viewing history, ratings, and genre preferences to personalize its recommendations, while social media platforms use a combination of user interactions, friend networks, and content popularity to curate personalized newsfeeds.
Comparison of Recommendation System Algorithms Across Platforms
Different platforms employ unique algorithms tailored to their specific content and user base. While the underlying principles are similar, the implementation and data utilized vary significantly.
Platform | Algorithm Type | Data Used | Personalization Methods |
---|---|---|---|
YouTube | Collaborative filtering, content-based filtering, contextual bandits | Watch history, search history, likes/dislikes, comments, engagement metrics, demographics | Personalized recommendations, tailored homepages, autoplay suggestions |
Netflix | Collaborative filtering, content-based filtering, knowledge-based systems | Viewing history, ratings, genre preferences, search history, device usage | Personalized recommendations, genre-specific lists, curated collections |
EdgeRank (now evolved), machine learning models | User interactions (likes, shares, comments), friend connections, content popularity, demographics, advertising data | Personalized newsfeed, targeted advertising, friend suggestions |
The Impact of Filter Bubbles and Echo Chambers
The highly personalized nature of content delivery can lead to the formation of filter bubbles and echo chambers. Filter bubbles restrict users’ exposure to information that challenges their existing beliefs or perspectives, creating a limited and potentially biased view of the world. Echo chambers amplify this effect by surrounding users with like-minded individuals and reinforcing their pre-existing biases. This can lead to polarization, decreased empathy, and a reduced capacity for critical thinking.
For example, a user primarily consuming news from sources aligned with their political views may become increasingly entrenched in their beliefs, with little exposure to alternative perspectives.
A Hypothetical Algorithm for Minimizing Filter Bubbles
Designing an algorithm that balances personalization with exposure to diverse viewpoints is a significant challenge. A hypothetical algorithm could incorporate several key features to mitigate the effects of filter bubbles and echo chambers. It would prioritize relevance while also introducing users to content outside their typical consumption patterns. This could involve incorporating a “diversity score” that measures the range of viewpoints presented to a user.
The algorithm would also employ techniques to surface content from sources that challenge the user’s pre-existing beliefs, while carefully managing the rate at which this happens to avoid overwhelming the user.
For instance, a weighted ranking system could be implemented. Content relevance would be weighted heavily, but a smaller weight could be assigned to the diversity score. This would ensure that relevant content remains prioritized, while simultaneously introducing diverse perspectives gradually. Furthermore, the algorithm could analyze the user’s engagement with diverse content to adapt the balance between personalization and diversity over time, providing a more nuanced and adaptive approach to content delivery.
AI’s Influence on Online Advertising
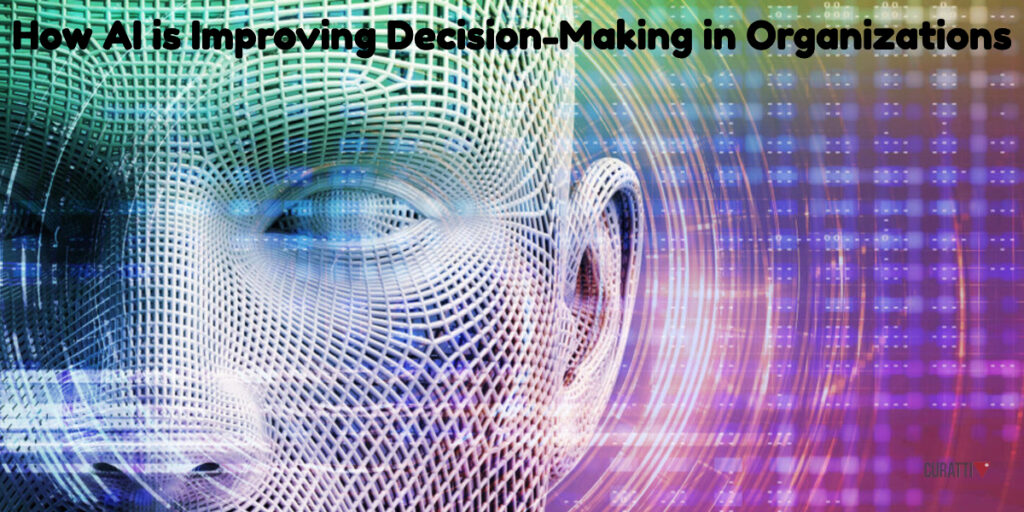
AI has fundamentally reshaped the landscape of online advertising, moving beyond broad demographic targeting to highly personalized campaigns that leverage vast amounts of user data. This shift has significantly increased advertising effectiveness for businesses while simultaneously raising significant ethical concerns regarding user privacy and potential manipulation.
AI-Powered Targeted Advertising: Data Collection and Profiling
AI-powered targeted advertising relies on sophisticated algorithms that analyze massive datasets to create detailed user profiles. This data collection involves tracking user behavior across various online platforms, including websites, social media, and mobile apps. Information gathered can include browsing history, search queries, social media interactions, location data, demographics, and purchase history. This data is then processed using machine learning techniques to identify patterns and predict user preferences, interests, and purchasing behaviors.
These predictions are used to create highly specific user profiles, allowing advertisers to target individuals with tailored ads that are more likely to resonate with them. For example, an algorithm might identify a user interested in sustainable living based on their online searches and social media activity, subsequently displaying ads for eco-friendly products. The sophistication of these algorithms allows for micro-targeting, reaching extremely specific segments of the population with remarkable precision.
Ethical Implications of Targeted Advertising
The ethical implications of AI-driven targeted advertising are complex and multifaceted. A primary concern revolves around user privacy. The extensive data collection practices inherent in personalized advertising raise questions about transparency and consent. Users are often unaware of the extent to which their online activities are being monitored and used to create detailed profiles. This lack of transparency can lead to feelings of unease and a sense of being manipulated.
Furthermore, the potential for manipulation is a significant ethical concern. Targeted advertising can exploit psychological vulnerabilities and biases, subtly influencing user choices without their conscious awareness. For instance, repeated exposure to ads for a particular product can create a sense of need or desire, even if the user had no prior interest in that product. This raises questions about the fairness and autonomy of individual decision-making in a digitally mediated environment.
Comparison of AI-Driven and Traditional Advertising
The effectiveness of AI-driven advertising compared to traditional methods is demonstrably superior in many aspects. This is primarily due to the precision targeting and personalization capabilities afforded by AI.
- Targeting Precision: AI allows for highly specific targeting, reaching individuals most likely to be interested in a product or service, unlike traditional methods which often rely on broader demographic categories.
- Cost-Effectiveness: By focusing resources on the most receptive audience segments, AI-driven advertising can significantly improve ROI compared to traditional advertising, which often involves broader, less targeted campaigns.
- Measurable Results: AI-powered advertising platforms provide detailed analytics and real-time performance tracking, allowing advertisers to optimize campaigns and measure their effectiveness precisely.
- Personalization: AI enables highly personalized ad experiences, tailoring messaging and creative assets to resonate with individual users, leading to increased engagement and conversion rates.
- Automation: AI automates many aspects of the advertising process, from campaign creation and optimization to bidding and targeting, freeing up human resources for other tasks.
Fictional Scenario Illustrating Negative Consequences
Imagine Sarah, a young woman struggling with body image issues. Through sophisticated AI-powered tracking of her online activity, she is relentlessly targeted with ads for weight-loss supplements and cosmetic procedures. These ads, cleverly disguised as helpful information or user testimonials, constantly reinforce her insecurities, leading to increased anxiety and a potentially unhealthy obsession with her appearance. This constant barrage of personalized advertising, designed to exploit her vulnerabilities, significantly contributes to her deteriorating mental health.
This fictional scenario highlights the potential for AI-driven advertising to exacerbate existing societal problems and cause significant harm if not implemented responsibly and ethically.
AI and Social Media Interactions: How AI Algorithms Shape Our Online Experiences And Daily Choices
AI algorithms are deeply embedded within the architecture of modern social media platforms, profoundly shaping how users interact, information disseminates, and online communities evolve. These algorithms, designed to optimize user engagement, often prioritize factors like content virality and user retention, leading to both beneficial and detrimental consequences. Understanding the multifaceted influence of AI on social media is crucial for navigating the digital landscape effectively.AI algorithms significantly influence the visibility and spread of information on social media platforms through sophisticated ranking systems and recommendation engines.
These systems analyze vast amounts of data, including user preferences, past interactions, and network connections, to determine which content is displayed prominently in users’ feeds. This process, while aiming to personalize the user experience, can inadvertently create filter bubbles and echo chambers, limiting exposure to diverse perspectives and potentially reinforcing existing biases. The algorithms’ focus on engagement metrics, such as likes, shares, and comments, can also inadvertently incentivize the creation and dissemination of sensational or emotionally charged content, regardless of its factual accuracy.
AI’s Role in Shaping Social Interactions and Online Communities, How AI algorithms shape our online experiences and daily choices
AI algorithms subtly but significantly shape social interactions and online communities. For example, the algorithms’ prioritization of certain types of content can influence the types of conversations that take place within online communities. Content that is deemed more “engaging” by the algorithm, even if controversial or divisive, is often given greater visibility, leading to more polarized discussions and potentially escalating conflicts.
Furthermore, the recommendation systems can inadvertently isolate users within echo chambers, surrounding them with like-minded individuals and limiting exposure to dissenting opinions. This can lead to the reinforcement of existing beliefs and hinder constructive dialogue. Conversely, AI can facilitate the formation of online communities around shared interests, connecting users who might not otherwise have found each other.
AI’s Application in Detecting and Mitigating Misinformation and Harmful Content
Social media platforms are increasingly leveraging AI algorithms to detect and mitigate the spread of misinformation and harmful content. These algorithms analyze text, images, and videos to identify patterns associated with disinformation campaigns, hate speech, and other forms of harmful content. For instance, algorithms can flag posts containing known false narratives or identify accounts engaged in coordinated disinformation efforts.
However, these systems are not foolproof and can be susceptible to manipulation. Furthermore, the definition of “misinformation” and “harmful content” can be subjective and culturally dependent, raising ethical considerations regarding censorship and freedom of speech. Facebook, for example, employs AI to detect and remove hate speech and terrorist propaganda, while Twitter utilizes algorithms to identify and flag potentially misleading information.
Potential Solutions to Address the Negative Impacts of AI on Social Media Engagement
Addressing the negative impacts of AI on social media requires a multi-pronged approach. Improved algorithm transparency is crucial; users should have a better understanding of how these systems work and the factors influencing content visibility. Promoting media literacy and critical thinking skills can empower users to evaluate information critically and resist manipulation. Furthermore, developing more robust and nuanced AI algorithms that prioritize accuracy and diversity of information, rather than solely focusing on engagement, is essential.
Finally, fostering collaboration between social media platforms, researchers, and policymakers is vital to establishing effective guidelines and regulations for AI-driven content moderation. This collaborative effort should focus on balancing the need to protect users from harmful content with the principles of free speech and open dialogue.
AI’s Role in Shaping Daily Choices
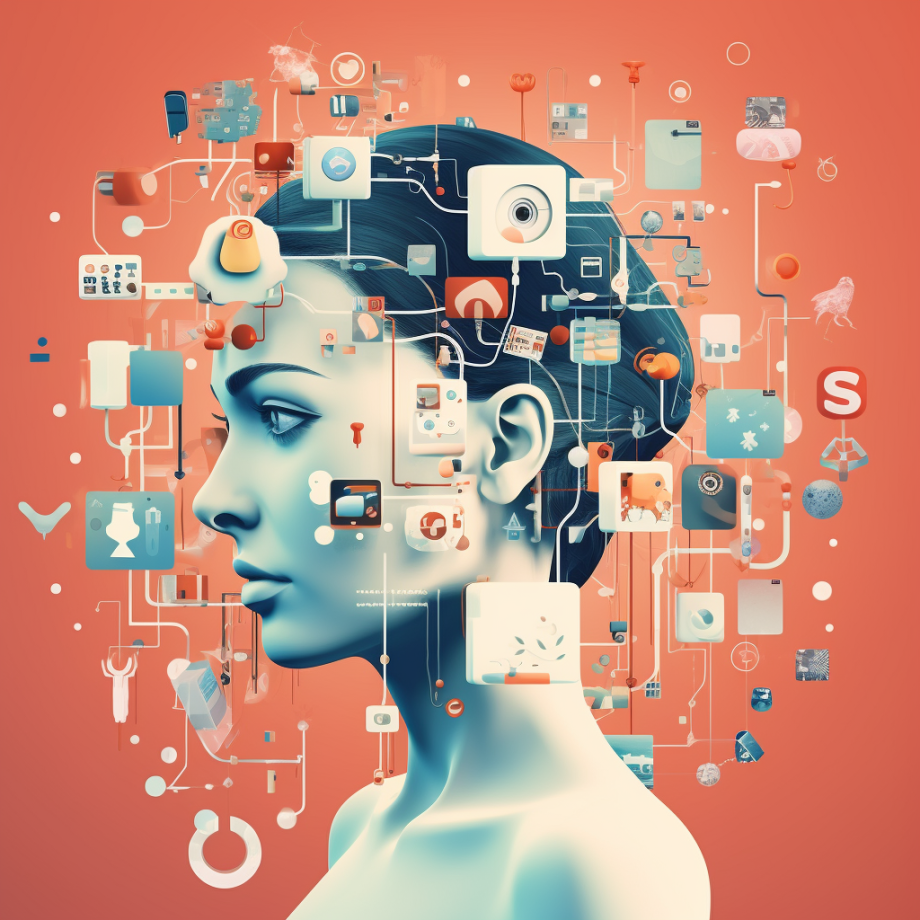
Artificial intelligence (AI) is no longer a futuristic concept; it’s deeply interwoven into the fabric of our daily lives, subtly yet powerfully influencing our choices and shaping our routines. From the moment we wake up to the time we go to sleep, AI algorithms are actively involved in our decision-making processes, often without us even realizing it. This pervasive influence stems from the increasing sophistication of AI-powered tools and their integration into various aspects of modern life.AI-powered virtual assistants and smart home devices significantly impact daily routines and decision-making processes.
These systems learn user preferences and habits over time, proactively offering suggestions and automating tasks. This personalized approach streamlines daily activities, reducing cognitive load and freeing up time for other pursuits. For instance, a smart home system might automatically adjust the thermostat based on occupancy and preferred temperature settings, while a virtual assistant could schedule appointments, set reminders, and even order groceries based on past purchasing behavior.
This automation, driven by AI algorithms, simplifies daily life and influences the choices we make regarding time management and resource allocation.
AI’s Influence on Purchasing Decisions
AI algorithms are pervasive in e-commerce, significantly impacting purchasing decisions through targeted promotions and product recommendations. These algorithms analyze vast datasets of user behavior, including browsing history, purchase history, and demographics, to predict preferences and tailor recommendations accordingly. For example, an online retailer might suggest products similar to those a user has viewed or purchased in the past, or it might display targeted advertisements based on the user’s interests and browsing behavior.
This personalized approach increases the likelihood of purchase, driving sales and shaping consumer choices by influencing what products are prominently displayed and considered. Furthermore, dynamic pricing, also driven by AI, adjusts prices based on demand and other factors, further impacting purchasing decisions.
AI’s Impact on Travel Choices and Route Planning
Navigation apps, heavily reliant on AI algorithms, fundamentally alter travel choices and route planning. These apps consider real-time traffic conditions, road closures, and alternative routes to provide users with the most efficient and fastest paths. By analyzing vast amounts of data from various sources, including GPS signals, traffic cameras, and user reports, AI algorithms optimize routes, influencing the decisions users make regarding their mode of transportation, departure times, and overall travel plans.
This optimization not only saves time but also impacts fuel consumption and environmental impact, indirectly influencing decisions related to sustainability and cost-effectiveness.
Visual Representation of AI Influence on Daily Choices
Imagine a flowchart depicting the influence of a smart home device on a user’s daily routine. The process begins with Data Collection, where the smart home system gathers information on user behavior: temperature preferences, sleep patterns (from a smart bed), appliance usage, and light preferences. This data is then processed through an AI Algorithm, which analyzes the data to identify patterns and preferences.
The algorithm uses this information to generate Personalized Recommendations, such as automatically adjusting the lighting and temperature at specific times or suggesting energy-saving measures. These recommendations then directly influence the user’s Daily Choices, leading to automated adjustments in their environment and ultimately shaping their daily routine. The loop then repeats, with the smart home system continuously learning and adapting to the user’s behavior, creating a dynamic feedback loop that continuously refines the AI’s understanding of the user and its ability to influence their choices.
The final stage is User Feedback, where the user’s actions (accepting or rejecting recommendations) further inform the AI, completing the cycle. This iterative process demonstrates the ongoing influence of AI on daily decisions.
The Impact of AI on Online Privacy
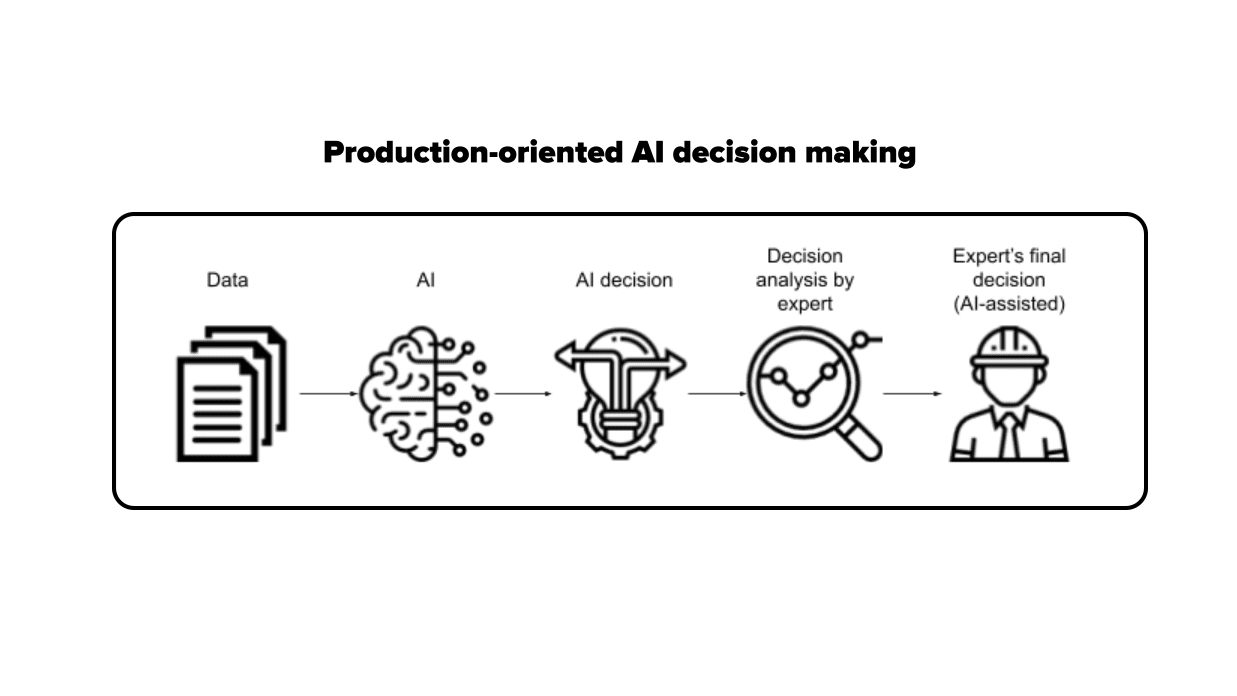
The increasing reliance on AI algorithms to personalize online experiences presents significant challenges to user privacy. The vast quantities of data collected to fuel these algorithms, ranging from browsing history and location data to social media interactions and purchasing habits, create a rich profile of individual users. This detailed portrait, while beneficial for targeted advertising and personalized content, simultaneously increases the vulnerability of individuals to data breaches and misuse.
The potential consequences range from identity theft and financial fraud to targeted manipulation and discrimination.The sheer scale and complexity of AI systems make it difficult to ensure data security and prevent unauthorized access. Data breaches, whether accidental or malicious, can expose sensitive personal information to third parties, leading to significant harm. Furthermore, the opaque nature of many AI algorithms, often described as “black boxes,” makes it challenging to understand how data is being used and processed, hindering effective oversight and accountability.
Data Collection Practices and Potential Misuse
AI algorithms thrive on data. The more data they process, the more accurate and effective they become. This insatiable appetite for data, however, raises serious privacy concerns. Companies collect vast amounts of user data, often without explicit or informed consent, blurring the lines between legitimate data collection for service improvement and exploitative data harvesting for profit maximization. This data can be misused for purposes beyond the user’s understanding or consent, such as creating detailed psychological profiles for targeted advertising or manipulating user behavior.
For example, a social media platform might use AI to identify users susceptible to misinformation campaigns, targeting them with specific content designed to influence their opinions or actions. The lack of transparency in data usage further exacerbates these concerns.
Challenges in Regulating AI Algorithms for Privacy Protection
Regulating AI algorithms to protect user privacy is a complex undertaking. The rapid pace of technological advancement often outstrips the ability of lawmakers and regulatory bodies to establish effective oversight mechanisms. Furthermore, the global nature of the internet makes it difficult to enforce regulations consistently across different jurisdictions. AI algorithms are often deployed across multiple countries, making it challenging to determine which laws apply and how to ensure compliance.
The technical complexity of AI systems also presents a significant hurdle. Regulators need to possess the technical expertise to understand how these algorithms work and to assess their potential impact on privacy. The lack of standardization and interoperability across different AI systems further complicates the regulatory landscape.
Approaches to Online Privacy Protection in AI-Driven Systems
Several approaches are being explored to mitigate the privacy risks associated with AI-driven systems. These strategies aim to balance the benefits of personalized services with the need to protect user privacy.
- Data Minimization: Collecting only the minimum amount of data necessary to provide a service. This reduces the potential impact of a data breach.
- Differential Privacy: Adding carefully designed noise to datasets to prevent the identification of individual users while preserving the overall statistical properties of the data.
- Federated Learning: Training AI models on decentralized data sources without directly sharing the data itself. This preserves the privacy of individual users while allowing for the development of accurate and effective AI models.
- Homomorphic Encryption: Performing computations on encrypted data without decrypting it. This allows for data processing while maintaining confidentiality.
- Enhanced User Control and Transparency: Providing users with greater control over their data, including the ability to access, modify, and delete their data, and ensuring greater transparency in how their data is used.
A Hypothetical System for Transparent and User-Controlled Data Usage
A hypothetical system for transparent and user-controlled data usage in AI-powered online services could incorporate several key features. Users would have granular control over the types of data collected and how it is used. They could selectively grant or revoke access to specific data points, enabling personalized experiences while maintaining privacy. The system would employ robust security measures to protect data from unauthorized access and breaches.
Furthermore, it would provide users with clear and understandable explanations of how their data is being used, allowing them to make informed decisions. Built-in mechanisms for data auditing and accountability would ensure compliance with privacy regulations and prevent misuse. Finally, the system would incorporate user-friendly interfaces that empower individuals to manage their data effectively. This approach would foster trust and empower users to actively participate in shaping their online experiences while safeguarding their privacy.
Wrap-Up
The pervasive influence of AI algorithms on our online experiences and daily choices is undeniable. While offering personalized content and streamlined experiences, these algorithms also present significant ethical and societal challenges. Understanding the mechanisms behind these algorithms, their data collection practices, and their impact on our decision-making processes is paramount. Moving forward, a collaborative effort involving developers, policymakers, and users is essential to ensure responsible AI development that prioritizes user privacy, combats misinformation, and fosters a more equitable and informed digital landscape.
Only through critical engagement and proactive measures can we harness the power of AI while mitigating its potential risks.