How AI assists in UI UX research and user testing is revolutionizing the design process. No longer confined to manual analysis and subjective interpretations, designers leverage AI’s power to glean deeper insights from user data, accelerating the creation of more intuitive and effective interfaces. From generating realistic user personas to automating A/B testing and predicting user behavior, AI streamlines workflows and enhances the accuracy of UX research, ultimately leading to better design outcomes.
This transformative technology allows for the analysis of vast datasets, identifying patterns and trends that might otherwise go unnoticed. This detailed analysis enables designers to make data-driven decisions, reducing reliance on guesswork and improving the overall user experience. However, ethical considerations surrounding data bias and responsible AI implementation remain crucial throughout this process.
AI-Powered User Persona Development
AI significantly enhances the creation and refinement of user personas, a cornerstone of effective UI/UX design. Traditional methods, while valuable, often struggle with the scale and depth of data required to represent diverse user groups accurately. AI’s ability to process vast datasets allows for the development of more nuanced and representative personas, leading to more informed design decisions.
This results in products and services that better resonate with target audiences.AI algorithms excel at identifying patterns and relationships within complex datasets, enabling the creation of user personas based on demographics, psychographics, and behavioral patterns. This goes beyond simple demographic segmentation, providing a richer understanding of user motivations, needs, and frustrations. By analyzing data from various sources – website analytics, social media activity, survey responses, and customer support interactions – AI can paint a detailed picture of different user segments, allowing designers to tailor their products to specific needs.
AI-Generated Persona Attributes Compared to Traditional Methods
The following table contrasts AI-generated personas with those developed through traditional research methods, highlighting the strengths and limitations of each approach. AI offers speed and scale, while traditional methods provide deeper qualitative insights, often requiring a more iterative and time-consuming process. The ideal approach often involves a hybrid model, leveraging the strengths of both.
Method | Persona Attribute | AI-Generated Data | Traditional Research Data |
---|---|---|---|
AI-Powered | Demographics (Age, Gender, Location) | Derived from large-scale datasets; precise statistical breakdowns. Example: 35-45 year old females residing in urban areas constitute 42% of the target audience. | Gathered from surveys and interviews; smaller sample size, potentially biased. Example: Interview data suggests a preference for mobile-first experiences among younger users. |
AI-Powered | Psychographics (Values, Attitudes, Lifestyle) | Identified through natural language processing (NLP) of social media posts and online reviews; reveals underlying motivations and preferences. Example: Analysis shows a strong correlation between environmentally conscious values and a preference for sustainable product packaging. | Derived from in-depth interviews and focus groups; provides rich qualitative data but may lack generalizability. Example: Focus group discussions reveal a desire for personalized product recommendations. |
AI-Powered | Behavioral Patterns (Website Usage, Purchase History) | Analyzes website analytics and transaction data; identifies user journeys and patterns. Example: AI identifies a significant drop-off rate during the checkout process on mobile devices. | Observed through usability testing and user journey mapping; provides insights into user behavior but may be limited by sample size and observation bias. Example: Usability testing reveals frustration with the complexity of the checkout form. |
AI-Powered | Goals and Frustrations | Extracted from user reviews, support tickets, and online forums; reveals unmet needs and pain points. Example: Sentiment analysis identifies negative feedback related to slow loading times and website navigation. | Uncovered through user interviews and surveys; provides detailed understanding of user needs but may require significant time and resources. Example: Interviews reveal a need for more intuitive search functionality. |
AI-Driven User Segmentation
AI algorithms, particularly clustering algorithms like k-means and hierarchical clustering, play a crucial role in segmenting users into meaningful groups. These algorithms analyze user data points across various dimensions (demographics, psychographics, behavior) to identify distinct clusters of users with similar characteristics. This allows researchers to create personas that accurately represent these distinct segments, rather than relying on broad generalizations.
For example, an e-commerce platform might use AI to identify three distinct user segments: budget-conscious shoppers, luxury buyers, and value-seeking bargain hunters. Each segment would then be represented by a unique persona with tailored needs and preferences. This granular segmentation allows for more targeted product development and marketing strategies.
AI in Usability Testing and Feedback Analysis
AI is revolutionizing usability testing and feedback analysis, offering significant improvements in efficiency and depth of insight. By automating previously manual tasks and leveraging advanced analytical capabilities, AI tools empower UX researchers to glean more meaningful data from user interactions and feedback, ultimately leading to better design decisions. This increased efficiency allows for more iterative testing and faster product development cycles.AI significantly enhances the analysis of user testing data, transforming how researchers understand user behavior and experience.
This involves automating the identification of critical moments during testing sessions, analyzing sentiment in user feedback, and summarizing key themes to provide actionable insights for design improvements.
Automated Analysis of User Testing Videos and Transcripts
AI algorithms can process user testing videos and transcripts, identifying key moments of frustration, confusion, or positive engagement. For example, facial expression analysis can detect signs of user struggle, while natural language processing (NLP) can identify negative s or phrases in verbal feedback. This automated analysis significantly reduces the time and effort required for manual review, allowing researchers to focus on interpreting the insights rather than simply gathering them.
Tools utilizing computer vision can pinpoint specific UI elements that users interact with poorly, highlighting areas requiring design changes. This level of detail provides a much more granular understanding of user behavior than traditional manual analysis.
AI-Driven Analysis of User Feedback from Surveys and Focus Groups
Several AI-powered tools are available to analyze user feedback from various sources like surveys and focus groups. These tools employ NLP and machine learning algorithms to identify recurring themes, sentiments (positive, negative, neutral), and key phrases within the data. For instance, a tool might summarize hundreds of survey responses into a concise report highlighting the most frequently mentioned issues and their overall sentiment.
This allows researchers to quickly grasp the overall user perception of the product or feature being tested, identifying areas for improvement and prioritizing design changes based on the intensity of user feedback. One example is using sentiment analysis to gauge the overall emotional response to a new feature – identifying overwhelmingly negative sentiment would clearly signal the need for a redesign.
Ethical Considerations of AI in User Data Interpretation
While AI offers powerful tools for analyzing user data, it’s crucial to address the ethical implications. AI algorithms are trained on data, and if that data reflects existing biases, the AI will perpetuate and potentially amplify those biases in its analysis. This could lead to skewed interpretations of user feedback and potentially discriminatory design choices. For example, an AI trained primarily on data from a specific demographic might misinterpret the needs and preferences of other demographics.
Transparency and careful consideration of potential biases in the training data and the AI’s output are essential to ensure ethical and fair analysis of user data. Regular audits of the AI’s performance and the data it uses are crucial to mitigating bias and ensuring responsible use. Furthermore, human oversight remains crucial to interpret the AI’s findings and ensure they align with ethical design principles.
AI-Driven A/B Testing and Optimization
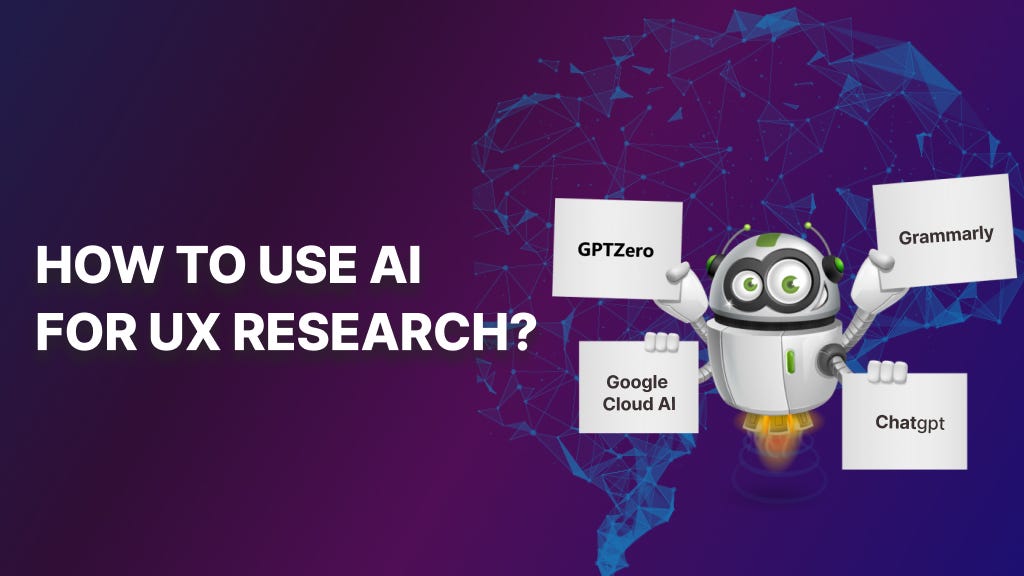
AI is revolutionizing A/B testing, moving beyond simple statistical analysis to offer powerful predictive capabilities and automation. This allows businesses to test more variations, faster, and with greater accuracy, ultimately leading to significant improvements in UI/UX and conversion rates. By leveraging machine learning algorithms, AI can analyze vast datasets, identify patterns, and predict the performance of different design iterations before they even go live.AI significantly streamlines the A/B testing process.
Instead of manually setting up tests, analyzing results, and iterating, AI can automate many of these steps. This frees up human resources to focus on higher-level strategic decisions and creative design exploration. The automation also allows for more frequent testing and faster iteration cycles, enabling a more agile approach to UI/UX optimization.
Automated A/B Testing Process
AI automates several key aspects of A/B testing. It can automatically select the best variations to test based on previous data and predicted performance, dynamically allocate traffic to maximize learning, and even automatically stop tests once a statistically significant winner is identified. This reduces the time and resources required for A/B testing, allowing for more experiments and a faster learning cycle.
Furthermore, AI can handle complex scenarios with multiple variations and numerous metrics simultaneously, providing a comprehensive understanding of design impact.
A/B Test Scenario: Website Landing Page Optimization
This scenario Artikels an A/B test to improve a website’s landing page conversion rate.
Hypotheses:
- Hypothesis 1: A landing page with a simplified form (fewer fields) will result in a higher conversion rate.
- Hypothesis 2: A landing page with a more compelling headline and hero image will result in a higher conversion rate.
Metrics:
- Conversion rate (percentage of visitors completing the desired action, e.g., filling out a form)
- Click-through rate (percentage of visitors clicking on a specific element, e.g., a call-to-action button)
- Average session duration
- Bounce rate (percentage of visitors leaving the page after viewing only one page)
Steps:
- Define Target Audience: Identify the specific demographic and behavioral characteristics of the target audience for the landing page.
- Create Variations: Design two or more variations of the landing page based on the hypotheses. Variation A will feature a simplified form, while Variation B will have a more compelling headline and hero image. A control group will also be used to compare performance.
- Implement A/B Testing Tool: Utilize an AI-powered A/B testing platform to manage the experiment and collect data.
- Allocate Traffic: Distribute website traffic evenly across the variations to ensure accurate data collection.
- Monitor and Analyze: Continuously monitor the performance of each variation. The AI platform will automatically analyze the data and identify statistically significant differences.
- Interpret Results: Based on the results, determine which variation performed best and implement the winning design.
Expected Outcomes: A statistically significant increase in conversion rate and click-through rate is expected for at least one of the variations compared to the control group.
Examples of Companies Using AI for A/B Testing
While specific details of internal AI-powered A/B testing processes are often proprietary, several companies publicly utilize AI-driven optimization platforms. These platforms often integrate machine learning to analyze data, predict outcomes, and automate aspects of the testing process. For instance, companies like Optimizely and AB Tasty offer AI-powered features within their A/B testing suites. While precise results aren’t always publicly shared due to competitive reasons, case studies often highlight significant improvements in conversion rates and user engagement achieved through the use of these AI-powered tools.
The general trend shows that AI-driven A/B testing leads to faster iteration cycles and more effective design optimization compared to traditional methods.
AI for Predictive UI/UX Design
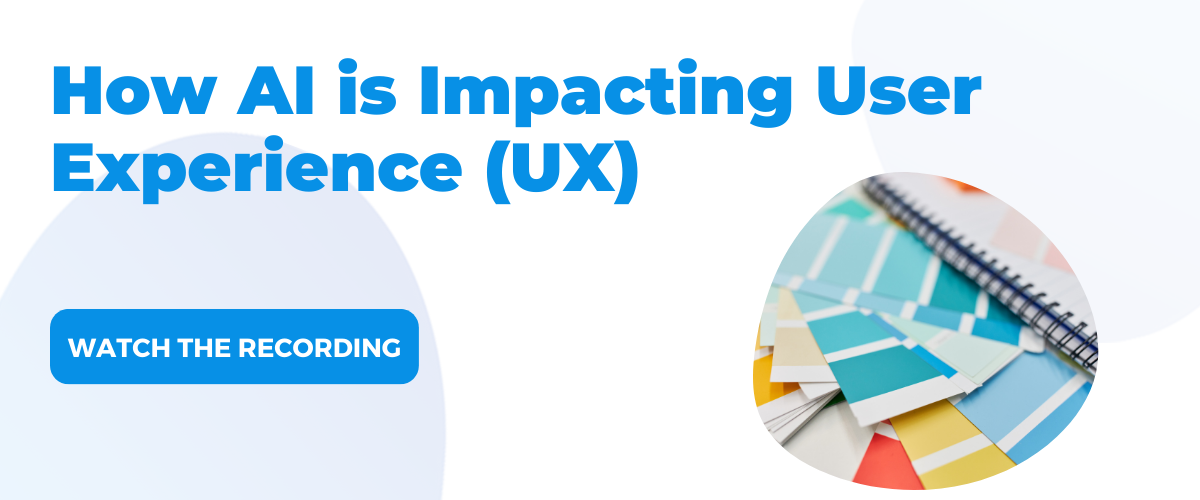
AI is rapidly transforming UI/UX design, moving beyond iterative testing and into the realm of predictive design. By leveraging machine learning algorithms trained on vast datasets of user interactions, AI can anticipate user behavior and preferences, leading to more intuitive and effective interfaces. This predictive capability allows designers to proactively address potential usability issues and personalize the user experience at scale, resulting in higher engagement and satisfaction.AI’s ability to predict user behavior stems from its capacity to analyze patterns and trends within user data.
This data can encompass various sources, including website analytics, user feedback, A/B testing results, and even eye-tracking studies. By identifying correlations between specific design elements and user actions, AI algorithms can build predictive models that forecast how users will interact with different interface designs. This allows designers to make data-driven decisions, optimizing the user experience before it even launches.
Personalized Interface Design Using AI
Imagine a financial planning application. Traditional design approaches would create a single interface for all users. However, using AI, the application could analyze individual user data—age, investment goals, risk tolerance, financial literacy—to generate a personalized interface. For a young user with high risk tolerance, the interface might prominently feature investment options with higher potential returns, visually represented with dynamic charts and graphs.
Conversely, an older user with a lower risk tolerance might see a more conservative interface emphasizing security and stability, with simpler data visualizations. This personalized approach ensures the interface is optimally tailored to each user’s needs and understanding, enhancing engagement and trust. The AI would continuously learn and adapt the interface based on ongoing user interactions, refining the personalization over time.
Challenges and Limitations of AI in Predictive UI/UX Design, How AI assists in UI UX research and user testing
The application of AI in predictive UI/UX design, while promising, faces several challenges. Firstly, the accuracy of AI predictions heavily relies on the quality and quantity of data used for training. Biased or incomplete datasets can lead to inaccurate predictions and potentially reinforce existing biases within the design. Secondly, the “black box” nature of some AI algorithms can make it difficult to understand the reasoning behind their predictions, hindering the designer’s ability to validate and interpret the results.
Transparency and explainability are crucial for building trust and ensuring responsible AI implementation. Thirdly, the ethical implications of using user data to personalize interfaces must be carefully considered. Data privacy and security are paramount, and users must be informed about how their data is being used and have control over their data. Finally, over-reliance on AI predictions could stifle creativity and intuition, potentially leading to homogenized and less innovative designs.
A balanced approach that combines AI-driven insights with human creativity is essential for achieving optimal results.
AI-Enhanced User Journey Mapping: How AI Assists In UI UX Research And User Testing
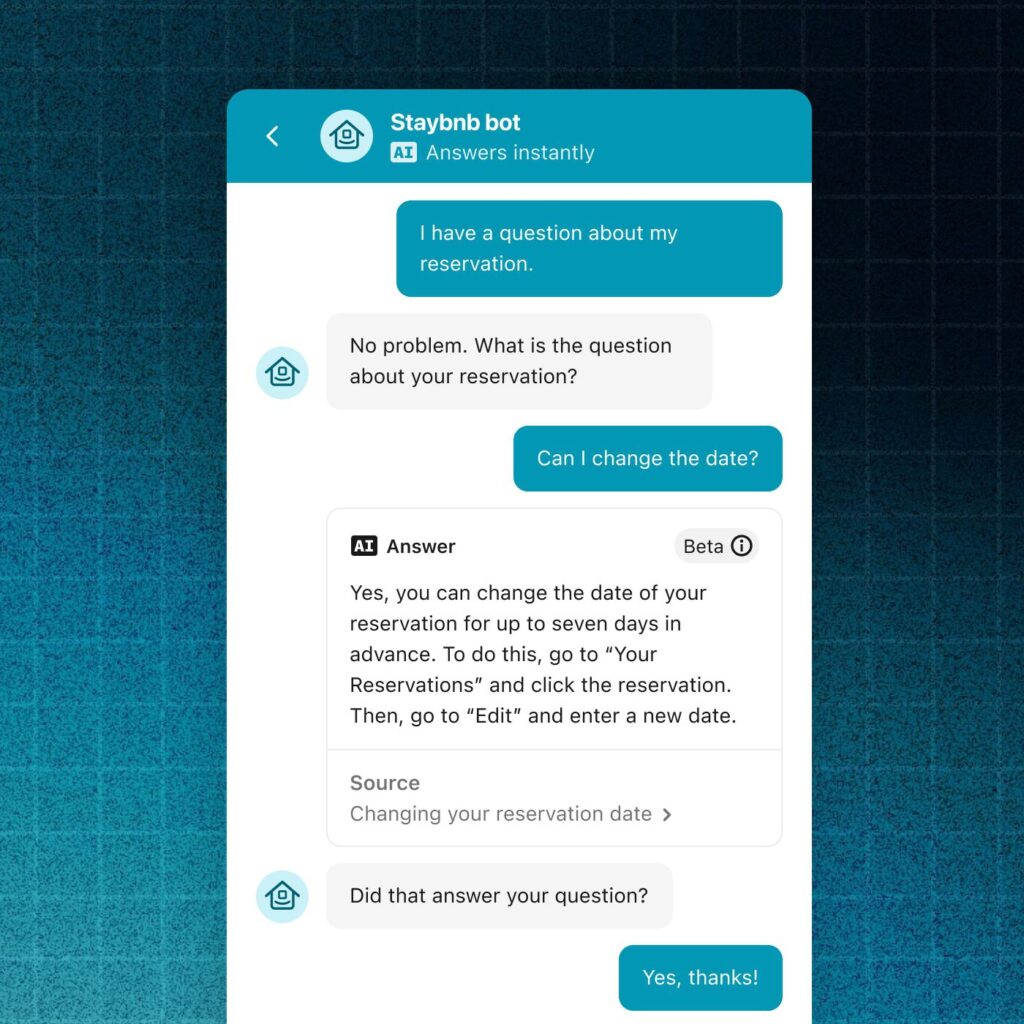
AI is revolutionizing user journey mapping by automating data analysis and providing deeper insights into user behavior. This allows UX researchers to create more accurate, comprehensive, and actionable maps, leading to improved user experiences and ultimately, increased business success. By leveraging AI’s capabilities, researchers can move beyond traditional, often time-consuming manual methods, uncovering hidden patterns and trends that would otherwise remain undetected.AI can analyze user data from multiple sources to build comprehensive user journey maps.
This includes website analytics (e.g., Google Analytics), app usage data (e.g., Firebase), CRM data, survey responses, social media interactions, and customer support logs. By consolidating this information, AI algorithms can identify key touchpoints, user pain points, and opportunities for optimization along the entire user journey. This holistic view provides a much richer understanding of the user experience than traditional methods, which often rely on a more limited dataset.
Comparison of AI-Driven and Traditional User Journey Mapping
Traditional user journey mapping typically involves manual data collection and analysis, often relying on user interviews, surveys, and observation. This process can be time-consuming, subjective, and prone to bias. AI-driven mapping, conversely, leverages machine learning algorithms to process large datasets, identifying patterns and insights that humans might miss. While AI offers speed and scale, it requires careful data preparation and validation to ensure accuracy and avoid misinterpretations.
The following table summarizes the key differences:
Feature | Traditional User Journey Mapping | AI-Driven User Journey Mapping |
---|---|---|
Data Sources | Limited to user interviews, surveys, observations | Multiple sources: website analytics, app usage data, surveys, CRM, social media |
Data Analysis | Manual, time-consuming, subjective | Automated, fast, objective (with proper validation) |
Insights | Potentially limited by sample size and researcher bias | Potentially more comprehensive and nuanced, revealing hidden patterns |
Cost | Relatively low initial cost, but high labor costs | Higher initial investment in technology and expertise, but lower ongoing costs |
Scalability | Difficult to scale for large user bases | Easily scalable to accommodate large datasets and user populations |
Example of an AI-Enhanced User Journey Map for a Food Delivery App
Let’s consider a food delivery app like Uber Eats. Using AI to analyze user data from app usage, order history, customer service interactions, and app store reviews, we can construct a detailed user journey map.Imagine an AI system identifying a significant drop-off in user engagement after the order placement stage. Further analysis reveals a high number of complaints regarding delayed deliveries and inaccurate order information.
This suggests a pain point in the order fulfillment and delivery process. The AI might also highlight that users who experience delays are significantly less likely to use the app again.The AI-generated user journey map would visually represent this, showing the user’s journey from searching for restaurants to receiving their food. The stage involving order fulfillment and delivery would be highlighted as a critical pain point.
Opportunities for improvement could include implementing real-time order tracking, improved communication with delivery drivers, and proactive notifications about potential delays. This granular level of insight, derived from AI-powered analysis, allows for targeted interventions to improve user satisfaction and retention. For example, the app could implement proactive notifications to users about potential delays, or a more robust customer support system to address order issues promptly.
The AI could even suggest personalized offers or discounts to compensate users for negative experiences, thereby mitigating churn.
AI in Accessibility and Inclusivity Testing
AI is revolutionizing accessibility and inclusivity testing in UI/UX design, automating processes previously reliant on manual effort and significantly expanding the scope of testing. This automation leads to more efficient identification of accessibility barriers, ultimately resulting in more inclusive and user-friendly digital products for a wider range of users. By leveraging AI’s capabilities, designers can ensure compliance with accessibility standards and create truly universal design experiences.AI algorithms can analyze websites and applications, identifying potential accessibility issues far more quickly and comprehensively than human testers alone.
This includes detecting problems with color contrast, keyboard navigation, screen reader compatibility, and other critical accessibility checkpoints. Furthermore, AI can analyze user behavior data to pinpoint areas of difficulty for users with disabilities, offering valuable insights for iterative design improvements. The integration of AI in this field is not simply about automation; it’s about augmenting human expertise and extending the reach of accessibility testing to a scale previously unimaginable.
AI-Powered Accessibility Testing Tools
Several AI-powered tools are emerging to automate accessibility testing. These tools use machine learning models trained on vast datasets of accessibility guidelines and best practices to analyze digital interfaces and flag potential problems. For example, some tools can automatically assess color contrast ratios against WCAG (Web Content Accessibility Guidelines) standards, alerting designers to areas needing improvement. Others can simulate screen reader interactions, identifying issues with screen reader compatibility and navigation.
Furthermore, AI can analyze images and videos for alt-text accuracy and completeness, ensuring that visually impaired users have access to the relevant information. These tools significantly reduce the time and resources required for comprehensive accessibility testing, allowing designers to address issues proactively and efficiently.
AI’s Role in Inclusive Design
Beyond automated testing, AI plays a crucial role in shaping more inclusive and user-friendly interfaces. By analyzing user data, including demographic information and user behavior patterns, AI can identify trends and patterns that indicate areas where the design might be excluding certain user groups. For example, AI could identify that a particular design element is difficult for users with motor impairments to interact with.
This data-driven approach allows designers to make informed decisions about design choices, ensuring that the interface is usable and enjoyable for all users, regardless of their abilities or backgrounds. AI can also be used to personalize the user experience, tailoring the interface to meet the specific needs and preferences of individual users, promoting inclusivity and accessibility at a granular level.
This personalized approach ensures that the digital experience is truly accessible and engaging for every user.
Closure
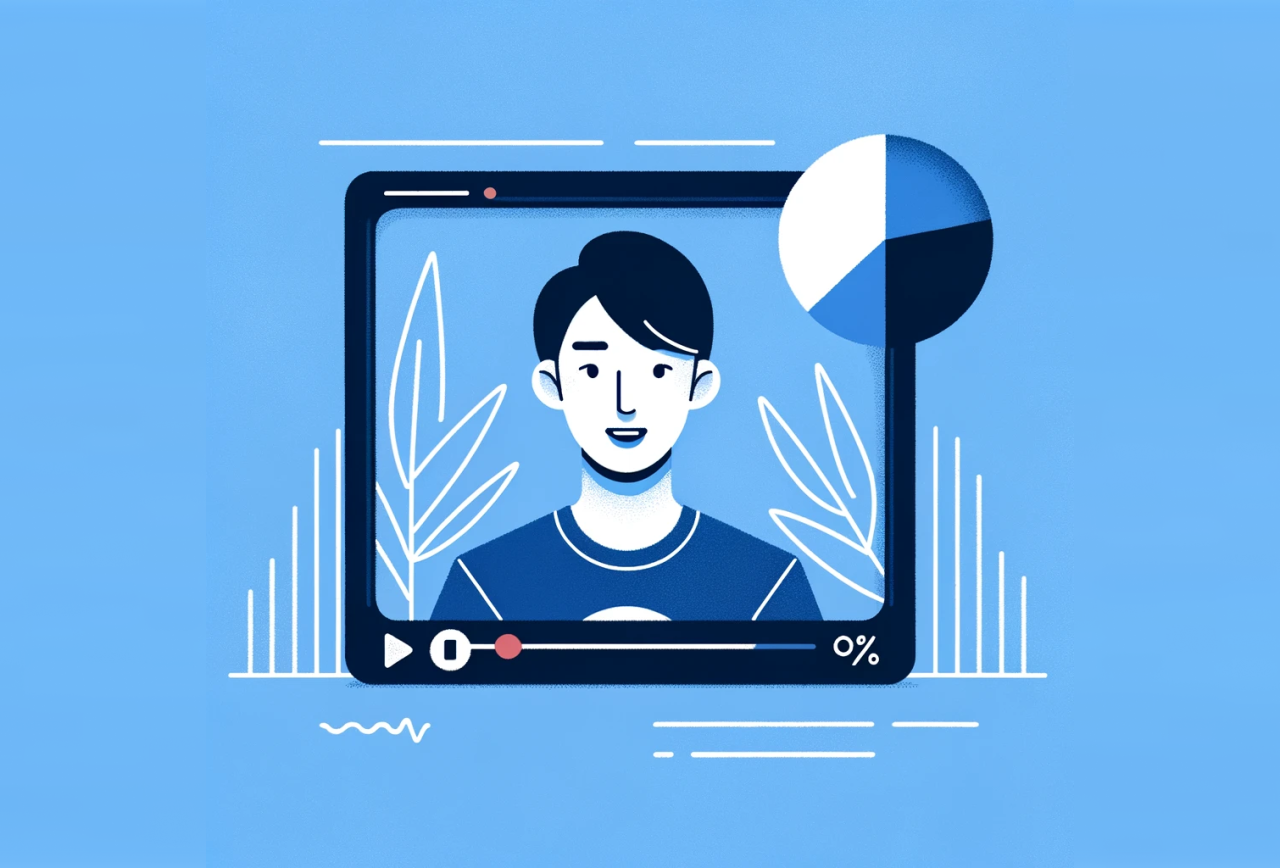
The integration of AI in UI/UX research and user testing marks a significant leap forward in design methodologies. By automating tedious tasks, providing deeper data analysis, and offering predictive capabilities, AI empowers designers to create truly user-centric products. While challenges and ethical considerations remain, the potential for improved user experiences through AI-driven insights is undeniable. The future of UI/UX is undeniably intertwined with the innovative applications of artificial intelligence.