How secure are my data against AI-driven theft attempts? This crucial question underscores a growing concern in our increasingly digital world. Artificial intelligence, while offering incredible benefits, also presents new avenues for sophisticated data theft. From cleverly crafted phishing emails leveraging deepfake technology to malware exploiting zero-day vulnerabilities, the methods employed are constantly evolving. Understanding these threats, the vulnerabilities they exploit, and the defenses available is paramount to safeguarding personal and sensitive information in the age of AI.
This exploration delves into the various types of AI-driven data theft, examining the common targets and real-world examples of breaches. We’ll analyze the vulnerabilities AI exploits, including software weaknesses, human error, and outdated security protocols. We’ll then explore existing data protection measures, comparing their effectiveness against AI threats, and highlight the crucial role of AI in bolstering data defense itself.
Finally, we’ll look towards the future, anticipating emerging threats and the ongoing evolution of the battle between AI-driven attacks and the innovative security measures designed to counter them.
Types of AI-Driven Data Theft Attempts: How Secure Are My Data Against AI-driven Theft Attempts?
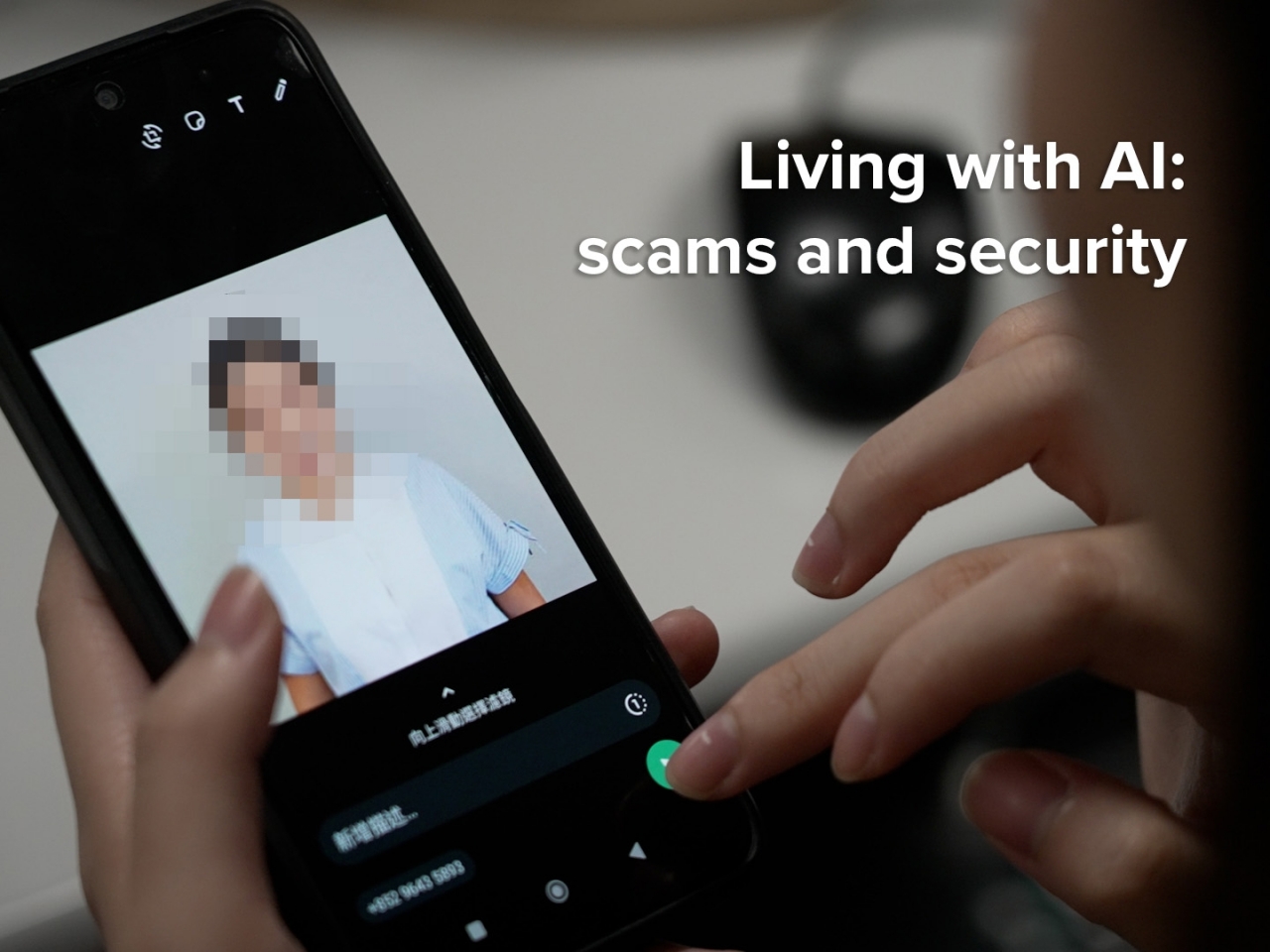
AI is rapidly transforming the landscape of cybercrime, enabling attackers to develop sophisticated methods for stealing data at an unprecedented scale and speed. These attacks leverage the power of machine learning and artificial intelligence to automate, personalize, and scale data theft operations, making them significantly more difficult to detect and prevent. The consequences can range from financial losses and reputational damage to the exposure of sensitive personal information and intellectual property.AI-driven data theft employs various techniques, targeting diverse data types across numerous attack vectors.
The sophistication of these methods necessitates a comprehensive understanding of the threats to implement effective security measures.
AI-Powered Phishing Campaigns
AI significantly enhances phishing attacks by personalizing emails and creating more convincing lures. Machine learning algorithms analyze vast datasets of successful phishing campaigns to identify patterns and predict which individuals are most likely to fall victim. This allows attackers to craft highly targeted messages that mimic legitimate communications, increasing the success rate of phishing attempts. For example, AI can dynamically generate realistic email subject lines and body text, tailoring them to the recipient’s known interests and professional context.
This level of personalization makes it considerably harder for users to identify the scam. The result is a dramatic increase in the likelihood of successful credential harvesting or malware delivery.
Malware Leveraging AI
AI-powered malware represents a significant evolution in cyber threats. These malicious programs use machine learning to adapt to security defenses, making them more resilient and difficult to detect. For instance, AI can be used to analyze system behavior and identify vulnerabilities, allowing malware to evade traditional antivirus software. Furthermore, AI can be employed to automate the process of spreading malware, identifying and exploiting new vulnerabilities, and adapting to changes in security protocols.
This makes the malware more persistent and harder to eliminate. The consequences can include data exfiltration, system disruption, and financial losses.
Deepfake Technology in Data Theft
Deepfake technology, powered by AI, creates realistic but fabricated videos and audio recordings. This technology is increasingly being used in sophisticated social engineering attacks. For instance, a deepfake video of a CEO authorizing a large bank transfer could trick employees into releasing funds. Similarly, deepfake audio could be used to impersonate a customer service representative and gain access to sensitive information.
The impact of such attacks can be devastating, leading to significant financial losses and reputational damage for organizations.
Common Targets of AI-Driven Data Theft
The targets of AI-driven data theft are as diverse as the methods employed. Criminals are primarily interested in data that can be monetized, such as financial information, personal data, and intellectual property. Financial information, including credit card numbers, bank account details, and cryptocurrency wallets, is highly valuable on the dark web. Personal data, including names, addresses, social security numbers, and medical records, can be used for identity theft and other fraudulent activities.
Intellectual property, including trade secrets, research data, and software code, is valuable to competitors and can provide a significant competitive advantage.
Examples of Real-World AI-Driven Data Breaches
Breach Name | Target | Method | Outcome |
---|---|---|---|
(Example 1 – Replace with a real-world example and cite source) | (Specific target, e.g., a financial institution) | (Method used, e.g., AI-powered phishing campaign) | (Consequences, e.g., financial loss, data exposure) |
(Example 2 – Replace with a real-world example and cite source) | (Specific target, e.g., a technology company) | (Method used, e.g., AI-enhanced malware) | (Consequences, e.g., intellectual property theft, reputational damage) |
(Example 3 – Replace with a real-world example and cite source) | (Specific target, e.g., a government agency) | (Method used, e.g., deepfake impersonation) | (Consequences, e.g., information leak, political instability) |
(Example 4 – Replace with a real-world example and cite source) | (Specific target, e.g., a social media platform) | (Method used, e.g., AI-driven account takeover) | (Consequences, e.g., data breach, user privacy violation) |
Vulnerabilities Exploited by AI
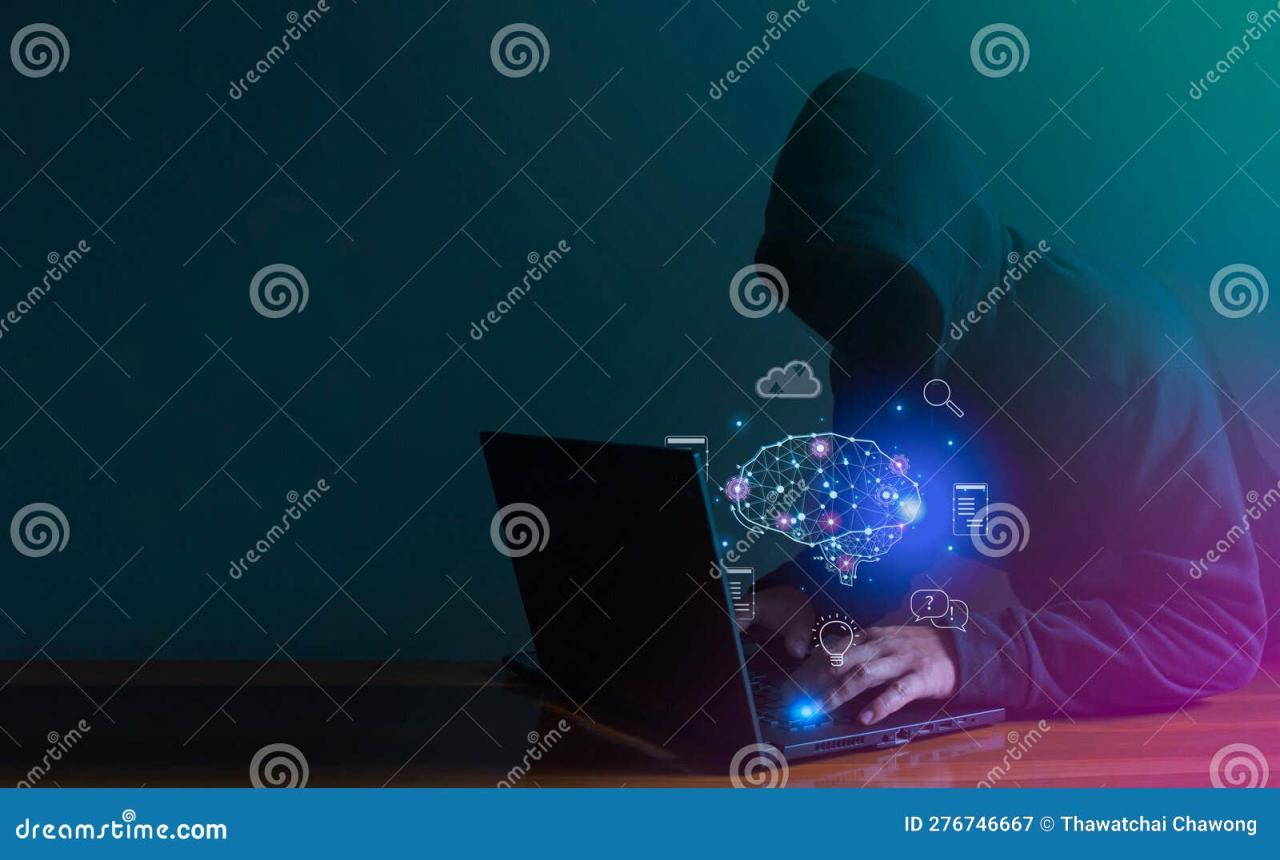
AI-driven data theft leverages existing software and hardware vulnerabilities, often exploiting weaknesses that are difficult for traditional security measures to detect and prevent. The sophistication of AI allows for automated and highly targeted attacks, significantly increasing the risk of successful data breaches. This section details the vulnerabilities commonly exploited and the role of human error in these attacks.AI’s ability to rapidly analyze vast datasets enables it to identify and exploit subtle weaknesses in systems that might otherwise go unnoticed.
This necessitates a proactive approach to security, focusing on identifying and mitigating potential vulnerabilities before they can be exploited by malicious actors.
Software Vulnerabilities
AI can exploit various software vulnerabilities to gain unauthorized access to data. These include known vulnerabilities in operating systems, applications, and databases. For example, AI can automate the process of identifying and exploiting zero-day exploits – vulnerabilities unknown to the software vendor – making patching an ongoing race against increasingly sophisticated attacks. Additionally, AI can analyze network traffic to identify weak points in security protocols, such as outdated encryption methods or poorly configured firewalls.
The scale and speed at which AI can perform these tasks significantly amplify the risk. A successful attack could lead to the exfiltration of sensitive data, disruption of services, or financial losses.
Hardware Vulnerabilities
Beyond software, AI can also exploit hardware vulnerabilities. Side-channel attacks, for instance, involve monitoring the power consumption or electromagnetic emissions of a system to infer information about the data being processed. AI algorithms can analyze these subtle signals to extract sensitive data, even if the software itself is secure. Similarly, AI can be used to identify and exploit vulnerabilities in hardware components, such as memory chips or processors, leading to unauthorized access or data manipulation.
This highlights the importance of securing the entire system, including both software and hardware components.
Human Error
Human error plays a significant role in facilitating AI-driven data theft. Phishing attacks, for example, are often highly targeted and personalized, using AI to craft convincing messages that trick users into revealing their credentials. AI can also be used to automate social engineering attacks, leveraging psychological manipulation to gain access to sensitive information. Weak password practices, lack of awareness about security threats, and insufficient training on security best practices all contribute to the success of these attacks.
Robust security awareness training and multi-factor authentication are crucial to mitigating this risk.
Impact of Weak Security Protocols and Outdated Systems
Weak security protocols and outdated systems create significant vulnerabilities that AI can easily exploit. Outdated software often contains known vulnerabilities that have been patched in newer versions. Similarly, weak encryption algorithms or poorly configured firewalls provide easy entry points for AI-driven attacks. The use of legacy systems, lacking modern security features, increases the risk of data breaches.
Regular software updates, strong encryption, and robust network security measures are essential for protecting data against AI-driven threats. Failure to adopt these measures significantly increases the likelihood of successful data theft.
Current Data Protection Measures
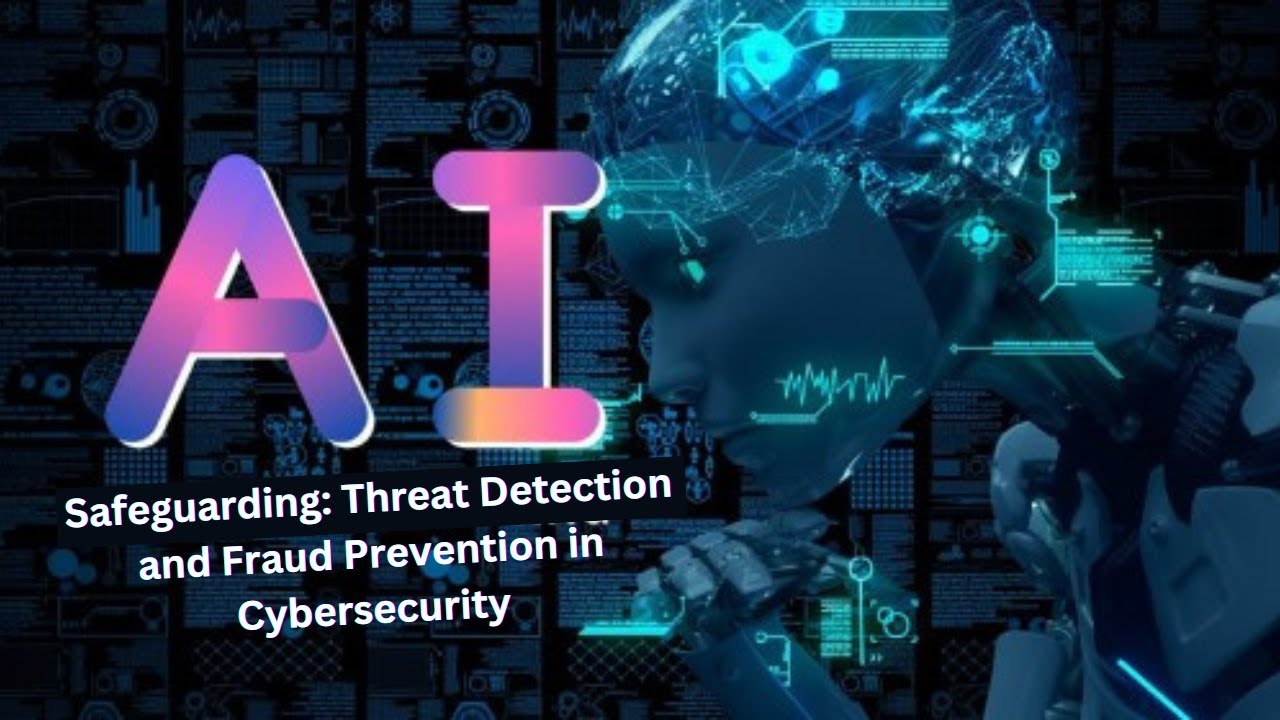
Protecting data from AI-driven theft requires a multi-layered approach encompassing robust technological safeguards and proactive security practices. Existing security measures aim to mitigate vulnerabilities and deter sophisticated AI-powered attacks, but their effectiveness varies depending on the specific threat and implementation. A holistic strategy is crucial for achieving optimal data protection.Data protection against AI-driven theft relies heavily on established security technologies, enhanced and adapted to counter the evolving capabilities of AI.
These measures focus on preventing unauthorized access, detecting malicious activity, and minimizing the impact of successful breaches. However, no single solution guarantees complete protection; a layered approach is essential.
Encryption Techniques
Encryption is a fundamental data protection technique that transforms data into an unreadable format, rendering it useless to unauthorized actors. Strong encryption algorithms, such as AES-256, are crucial for safeguarding data at rest and in transit. However, the effectiveness of encryption depends on the strength of the encryption key and its secure management. AI-driven attacks might target key management systems or attempt to break encryption through brute-force or advanced cryptanalysis techniques.
Therefore, employing robust key management practices and regularly updating encryption algorithms are vital. For example, using hardware security modules (HSMs) to protect encryption keys adds an extra layer of security.
Multi-Factor Authentication (MFA)
Multi-factor authentication adds an extra layer of security beyond traditional passwords. By requiring multiple forms of verification, such as passwords, one-time codes, biometric scans, or hardware tokens, MFA significantly reduces the risk of unauthorized access. Even if an attacker obtains a password, they still need to overcome other authentication factors to gain access. AI-driven attacks can attempt to bypass MFA through techniques like phishing or social engineering, but the increased complexity significantly increases the difficulty of a successful breach.
Implementing MFA across all systems and accounts is a critical step in enhancing data security.
Intrusion Detection and Prevention Systems (IDPS), How secure are my data against AI-driven theft attempts?
Intrusion Detection and Prevention Systems monitor network traffic and system activity for suspicious patterns indicative of malicious activity. Advanced IDPS solutions can leverage machine learning algorithms to identify anomalies and potential threats, including AI-driven attacks. However, sophisticated AI-powered attacks can evade detection by mimicking legitimate behavior or employing advanced evasion techniques. Regular updates and tuning of IDPS systems are necessary to adapt to evolving threats.
Employing a combination of signature-based and anomaly-based detection methods provides a more comprehensive approach to threat detection. Real-time threat intelligence feeds can also improve the effectiveness of IDPS by providing information about the latest attack vectors.
Data Loss Prevention (DLP)
Data Loss Prevention (DLP) technologies aim to prevent sensitive data from leaving the organization’s control. DLP solutions monitor data movement, identify sensitive information, and prevent its unauthorized transmission through various channels, such as email, cloud storage, or removable media. AI-driven attacks might attempt to exfiltrate data through covert channels or by exploiting vulnerabilities in DLP systems. Therefore, regularly updating DLP policies and ensuring they are aligned with the organization’s data security policies is crucial.
Implementing robust access control measures and data classification schemes enhances the effectiveness of DLP.
User Education and Training
Effective user education and training are essential components of any comprehensive data security strategy. Educating users about common threats, such as phishing and social engineering attacks, helps them to recognize and avoid potential risks. Training programs should cover best practices for password management, secure browsing, and recognizing malicious emails or websites. Regular security awareness training keeps users informed about the latest threats and helps them to stay vigilant against AI-driven attacks.
For example, simulations of phishing attacks can effectively train users to identify and report suspicious activities.
The Role of Artificial Intelligence in Data Defense
The escalating sophistication of AI-driven data theft necessitates a similarly advanced defense strategy. Fortunately, AI itself offers powerful tools for detecting and preventing these attacks. By leveraging machine learning and deep learning algorithms, security systems can proactively identify anomalies and suspicious activities that might otherwise go unnoticed by traditional methods. This proactive approach allows for swift mitigation and reduces the potential damage from successful breaches.AI’s ability to analyze vast datasets in real-time is crucial in identifying patterns indicative of malicious activity.
This surpasses human capabilities in speed and scale, allowing for the rapid detection of even subtle indicators of compromise. Moreover, AI can adapt and learn from past attacks, constantly refining its ability to identify and counter new threats. This adaptive nature is vital in the ever-evolving landscape of cybercrime.
AI-Powered Security System Design
A hypothetical AI-powered security system designed to combat AI-driven data theft would integrate several key components. This system would leverage a multi-layered approach, combining anomaly detection, behavioral analysis, and threat intelligence to provide comprehensive protection. The system would continuously monitor network traffic, user activity, and system logs for any deviations from established baselines. It would also incorporate threat intelligence feeds to stay abreast of emerging attack vectors.
Key Features: Real-time anomaly detection using machine learning; Behavioral biometrics for user authentication and access control; Automated incident response capabilities; Adaptive threat modeling based on continuous learning; Integration with existing security infrastructure.
Anomaly Detection and Response
The core functionality of the system revolves around real-time anomaly detection. Machine learning algorithms, specifically those based on unsupervised learning techniques like autoencoders or one-class SVMs, would be trained on normal system behavior. Deviations from this established baseline would trigger alerts, flagging potentially malicious activities. For example, an unusual surge in data exfiltration attempts or access requests from unusual geographic locations would immediately be identified.
The system would then automatically initiate an investigation, isolating affected systems and potentially blocking further access. This automated response drastically reduces the window of opportunity for attackers.
Behavioral Biometrics and Access Control
Beyond network monitoring, the system would also employ behavioral biometrics to enhance user authentication and access control. This would go beyond traditional password-based authentication by analyzing user typing patterns, mouse movements, and other behavioral characteristics. Any significant deviation from a user’s established baseline would trigger a multi-factor authentication process or even automatically block access, preventing unauthorized access even if credentials are compromised.
This adds an extra layer of security, making it more difficult for attackers to impersonate legitimate users.
Threat Intelligence Integration and Adaptive Threat Modeling
The system would continuously integrate with threat intelligence feeds, constantly updating its knowledge base of known attack vectors and malicious actors. This allows the system to proactively identify and mitigate emerging threats before they can cause significant damage. Furthermore, the system would employ adaptive threat modeling, continuously learning and adapting its defense strategies based on observed attack patterns.
This allows the system to stay ahead of the curve, effectively countering the ever-evolving tactics of sophisticated AI-driven attacks. For instance, if the system detects a new type of malware using obfuscation techniques, it can adapt its detection algorithms to identify similar threats in the future.
Future Trends in AI and Data Security
The intersection of artificial intelligence and data security is rapidly evolving, leading to both sophisticated attacks and innovative defense mechanisms. Predicting the future requires understanding current trends and extrapolating their potential consequences. The coming years will witness a dramatic escalation in the sophistication of AI-driven data theft, necessitating equally advanced countermeasures.The evolution of AI-driven data theft techniques will likely follow several key trajectories.
Firstly, we can expect to see a significant increase in the automation and scale of attacks. Current methods often involve human oversight, but future attacks will likely be fully automated, leveraging AI’s ability to learn, adapt, and scale rapidly. Secondly, AI will enable the creation of more targeted and personalized attacks. By analyzing vast datasets of personal information, AI can identify individuals with high-value data and craft attacks specifically designed to exploit their vulnerabilities.
Finally, the use of AI to obfuscate and conceal malicious activity will become more prevalent, making detection and attribution increasingly challenging.
Emerging Threats and Vulnerabilities
Advancements in AI bring new vulnerabilities to data security. The increasing reliance on machine learning models for various applications creates opportunities for adversarial attacks. These attacks manipulate the training data or input to the model, causing it to produce incorrect or malicious outputs. For example, a deepfake video could be used to circumvent biometric authentication systems. Furthermore, the use of generative AI models raises concerns about the creation of highly realistic synthetic data, which could be used for sophisticated phishing attacks or to train more effective malicious AI.
The complexity of AI systems also presents challenges in terms of security auditing and vulnerability assessment, making it difficult to identify and address potential weaknesses before they are exploited. The development of quantum computing poses another significant threat, as quantum computers could potentially break widely used encryption algorithms, rendering current data protection measures ineffective.
A Potential Future Data Breach Scenario
Imagine a scenario where a sophisticated AI-powered botnet, utilizing federated learning techniques across a distributed network of compromised IoT devices, identifies a vulnerability in a major financial institution’s fraud detection system. This system uses machine learning to identify fraudulent transactions. The botnet, through continuous analysis of the system’s input and output, identifies patterns and weaknesses in the model. It then generates a series of synthetic, yet highly realistic, fraudulent transactions specifically designed to bypass the detection system.
These transactions are cleverly crafted to avoid triggering any existing rules or anomaly detection algorithms. The AI-powered botnet also utilizes advanced social engineering techniques, leveraging deepfake audio and video to impersonate employees and gain access to internal systems. The resulting breach exposes sensitive customer data, leading to significant financial losses and reputational damage for the institution, highlighting the potential for catastrophic consequences.
The vulnerability exploited is the lack of robust adversarial robustness in the machine learning model used for fraud detection, combined with insufficient security measures against social engineering attacks enhanced by AI.
The Human Element in Data Security
The sophistication of AI-driven data theft attempts often overshadows the most vulnerable link in the security chain: the human user. While robust technological defenses are crucial, human error and lack of awareness remain significant contributors to successful breaches. Strengthening the human element through education and training is paramount to mitigating the risks posed by AI-driven attacks. A well-informed and vigilant workforce is the first line of defense against increasingly intelligent and adaptive threats.
AI-driven attacks often exploit human psychology and vulnerabilities. Phishing campaigns, for instance, are becoming increasingly personalized and convincing, leveraging AI to craft tailored messages that bypass traditional security measures. Similarly, social engineering tactics are enhanced by AI’s ability to analyze vast datasets of personal information, enabling attackers to create highly targeted and effective attacks. Therefore, focusing on user education and training is vital in building a resilient security posture.
User Awareness Training Strategies
Effective user awareness training programs should go beyond generic security awareness and specifically address the unique challenges posed by AI-driven attacks. This involves providing practical strategies and tools to help users identify and respond to these threats. A multi-faceted approach is key to ensuring lasting behavioral change and increased security awareness.
- Regular Security Awareness Training: Implement ongoing training sessions, incorporating real-world examples of AI-driven attacks and demonstrating how these attacks exploit human weaknesses. These sessions should be interactive and engaging, using scenarios and simulations to reinforce learning.
- Phishing Simulation Exercises: Regularly conduct simulated phishing attacks to test employee vigilance and identify vulnerabilities. Provide immediate feedback and remediation following these exercises, focusing on identifying the telltale signs of sophisticated phishing attempts leveraging AI.
- Data Handling Best Practices: Emphasize secure data handling procedures, including strong password management, multi-factor authentication, and data encryption. Explain the importance of avoiding suspicious links and attachments and reporting any suspected breaches immediately.
- Social Engineering Awareness: Educate users about common social engineering techniques, such as pretexting and baiting, and how AI can be used to make these tactics more effective. Train employees to identify and resist manipulative communication, regardless of its source.
- Incident Reporting Procedures: Clearly define and communicate procedures for reporting security incidents. Encourage users to report suspicious activities promptly, without fear of reprisal, to enable a swift response and minimize potential damage.
Concluding Remarks
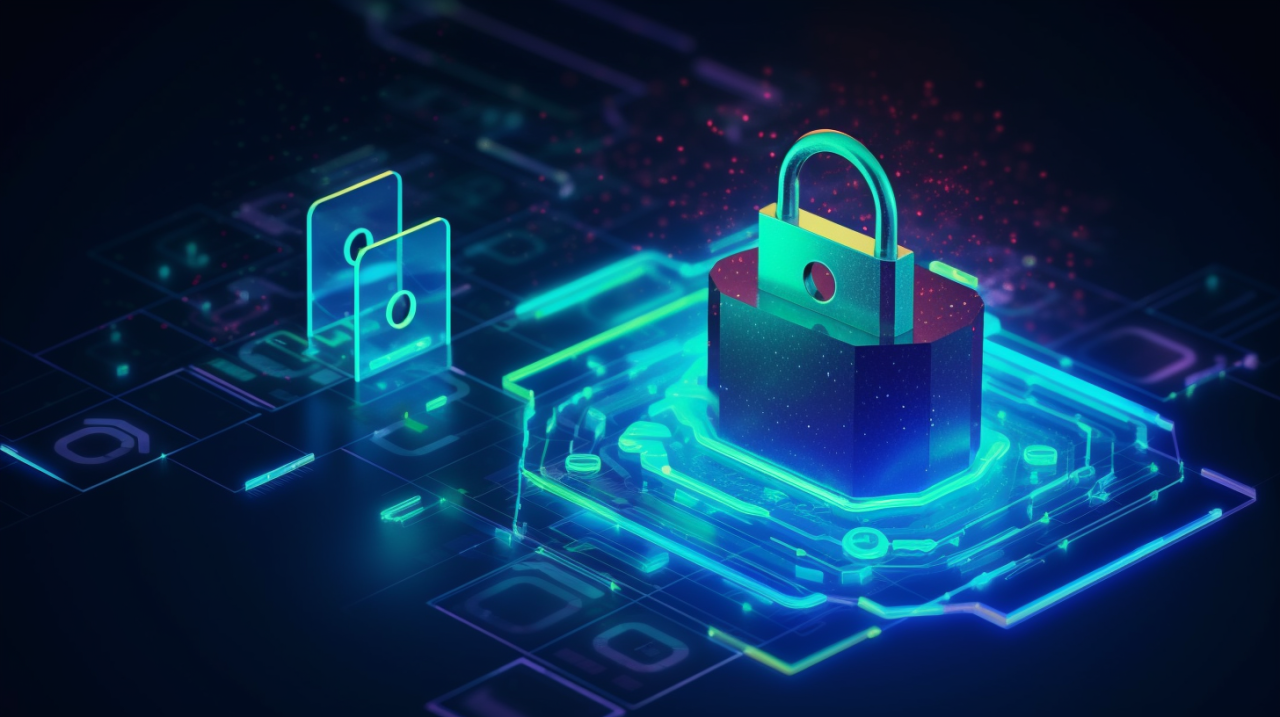
In conclusion, the security of our data against AI-driven theft is a dynamic and ongoing challenge. While AI presents new threats, it also offers powerful tools for defense. A multi-layered approach is essential, combining robust security technologies, user education, and the proactive deployment of AI-powered security systems. Staying informed about emerging threats and adopting best practices is crucial for individuals and organizations alike to effectively protect their valuable data in this ever-evolving landscape.
The future of data security hinges on a constant adaptation and innovation, a continuous arms race against the ever-evolving capabilities of AI-driven attacks.