How to integrate AI tools into existing UI UX design processes is no longer a futuristic concept; it’s a present-day reality reshaping the design landscape. This guide navigates the practical application of AI, exploring its potential to streamline workflows, enhance creativity, and ultimately deliver superior user experiences. We’ll delve into specific tools, address ethical considerations, and provide a roadmap for seamless integration into your existing design process, maximizing efficiency and innovation.
From leveraging AI for user research and prototyping to utilizing its power in design iteration and accessibility checks, we’ll cover the entire design lifecycle. We’ll examine both the benefits and potential pitfalls of AI integration, providing actionable strategies to mitigate risks and ensure human oversight remains central to the design process. By the end, you’ll have a clear understanding of how to harness the power of AI to elevate your UI/UX design capabilities.
Identifying Suitable AI Tools for UI/UX Design: How To Integrate AI Tools Into Existing UI UX Design Processes
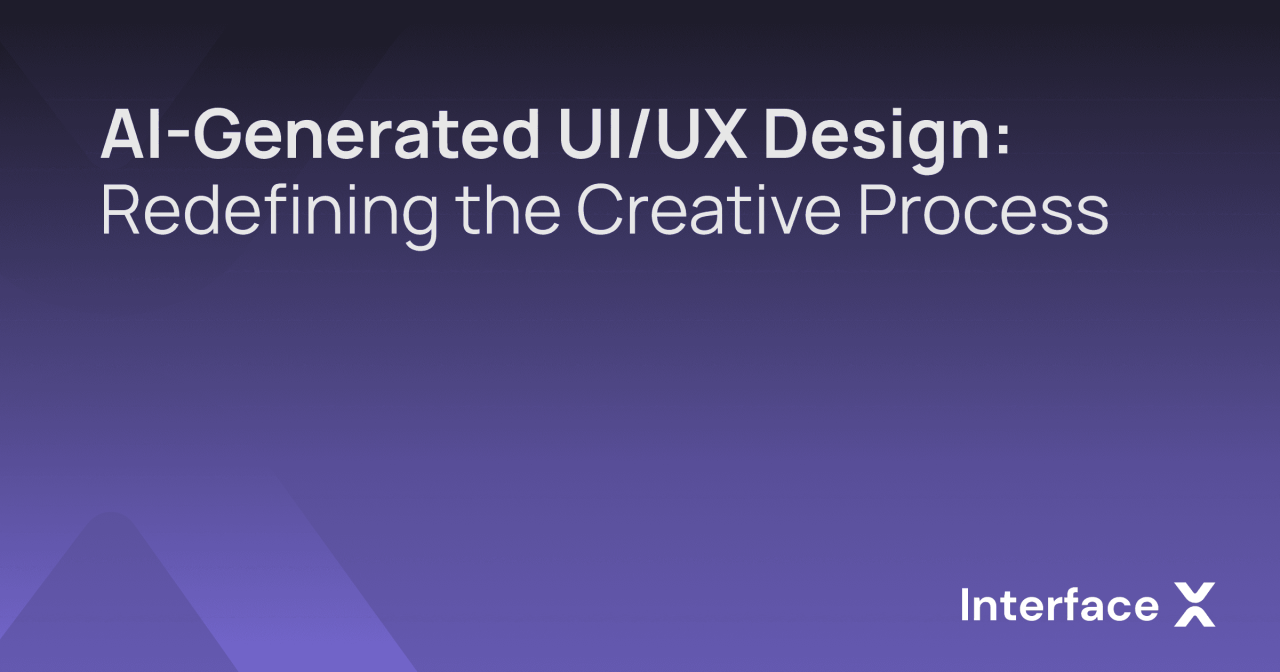
Integrating AI into UI/UX workflows offers significant potential for increased efficiency and creative exploration. However, selecting the right AI tools requires careful consideration of their capabilities and how well they align with specific design needs. This section will explore various AI tools categorized by function, analyze the strengths and weaknesses of prominent examples, and compare their cost-effectiveness for different team sizes.
AI Tools Categorized by Function
Choosing the appropriate AI tool hinges on understanding its specific functionalities within the UI/UX design process. The following table categorizes several AI tools based on their primary applications, highlighting key features and integration complexity.
Tool Name | Category | Key Features | Integration Difficulty |
---|---|---|---|
Midjourney | Image Generation | Generates high-quality images from text prompts; various art styles; versioning and upscaling options. | Easy (primarily standalone, but images can be easily integrated into design software) |
DALL-E 2 | Image Generation | Generates realistic and stylized images from text descriptions; image editing and variation capabilities; strong API integration. | Medium (API integration requires coding knowledge, but readily usable through the web interface) |
Figma | Prototyping & Collaboration | AI-powered features for design suggestions, content generation, and design system management; built-in collaboration features. | Easy (AI features integrated directly into the platform) |
Adobe Firefly | Image Generation & Editing | Generates images from text prompts; integrates seamlessly with Adobe Creative Cloud; supports various image editing tasks. | Easy (integrated within the Adobe ecosystem) |
Userlytics | User Research | AI-powered analysis of user testing sessions; identifies usability issues and areas for improvement; generates reports. | Medium (requires integration with existing user testing platforms) |
Strengths and Weaknesses of Prominent AI Tools
A deeper understanding of individual tools is crucial for effective integration. The following analysis examines three prominent AI tools: Midjourney, DALL-E 2, and Figma (with its AI features).
Tool | Strengths | Weaknesses |
---|---|---|
Midjourney | Exceptional image quality; diverse artistic styles; strong community support; relatively easy to use. | Limited control over specific details; reliance on text prompts; potential for unexpected outputs; subscription-based model. |
DALL-E 2 | Realistic and stylized image generation; powerful editing capabilities; strong API for integration; versatile applications. | Can be more complex to use than Midjourney; potential for bias in generated images; requires careful prompt engineering. |
Figma (AI features) | Seamless integration into existing workflow; improves design efficiency; assists with content generation and design system management. | Limited AI capabilities compared to dedicated image generation tools; reliance on Figma platform; ongoing development of features. |
Cost-Effectiveness Across Team Sizes
The cost-effectiveness of AI tools varies significantly depending on team size and specific needs. The following table provides a simplified cost comparison, acknowledging that pricing models can change.
Tool | Small Team (1-3 designers) | Medium Team (4-10 designers) | Large Team (10+ designers) |
---|---|---|---|
Midjourney | Cost-effective for occasional use | Potentially expensive for frequent use | Expensive; may require multiple subscriptions |
DALL-E 2 | Moderate cost; suitable for specific projects | Cost-effective with efficient usage | Requires careful budget planning; potentially expensive for extensive use |
Figma (AI features) | Cost-effective; included in existing subscription | Cost-effective; included in existing subscription | Cost-effective; included in existing subscription |
Integrating AI into the User Research Phase
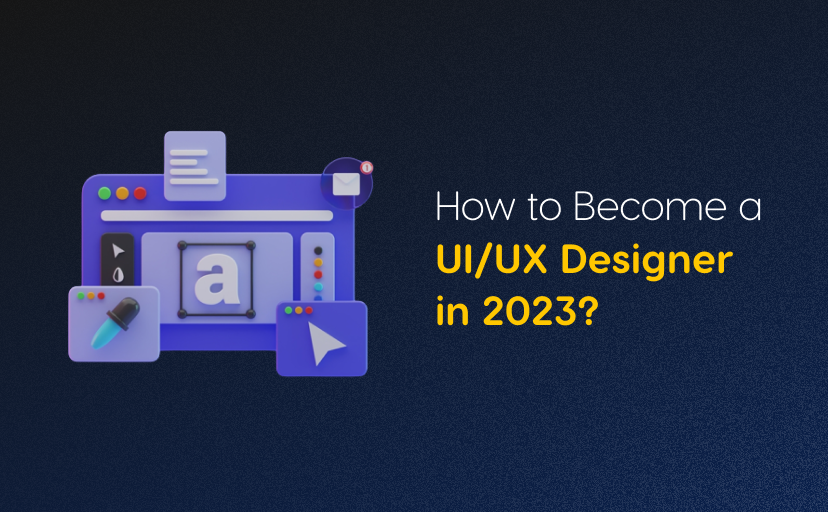
AI is rapidly transforming user research, offering powerful tools to analyze qualitative and quantitative data more efficiently and extract deeper insights. By integrating AI-powered solutions, UX designers can streamline their research processes, uncover hidden patterns in user behavior, and ultimately create more user-centered designs. This section will explore how AI can enhance various stages of the user research process, focusing on user interview analysis, sentiment analysis of feedback, and AI-driven persona generation.AI-powered tools significantly improve the efficiency and depth of user research analysis.
Manual analysis of user interviews, surveys, and feedback can be time-consuming and prone to subjective interpretation. AI offers a solution by automating parts of this process, allowing researchers to focus on higher-level strategic analysis.
AI-Enhanced User Interview Analysis
AI tools can transcribe audio and video recordings of user interviews, automatically identify key themes and topics, and even flag potential pain points or areas of frustration. Natural Language Processing (NLP) algorithms analyze the transcribed text, identifying recurring s, phrases, and sentiments. This allows researchers to quickly summarize large amounts of interview data, identifying key trends and patterns that might otherwise be missed.
For instance, a tool might identify the repeated mention of “difficult navigation” across multiple interviews, highlighting a crucial area for design improvement. This automated analysis accelerates the research process and reduces the risk of human bias in interpretation.
Sentiment Analysis of User Feedback
Sentiment analysis, also known as opinion mining, is a powerful AI technique used to determine the emotional tone behind user feedback. By analyzing text data from surveys, online reviews, or social media posts, AI can classify feedback as positive, negative, or neutral. This provides valuable insights into user satisfaction and helps identify areas needing improvement. For example, analyzing customer reviews of a mobile app might reveal a high proportion of negative sentiment related to the app’s loading speed, indicating a critical area for optimization.
This data-driven approach provides a more objective and comprehensive understanding of user sentiment compared to manual analysis. Tools can even identify the specific aspects of the product or service driving negative sentiment, enabling more targeted improvements.
AI-Driven Persona Generation
Creating accurate and representative user personas is crucial for effective design. AI can automate and enhance this process. By analyzing large datasets of user research data, including demographic information, behavioral patterns, and interview transcripts, AI algorithms can identify clusters of users with similar characteristics. This allows for the automated generation of personas, complete with detailed descriptions of their needs, goals, and pain points.
A step-by-step guide for incorporating AI-driven persona generation is as follows:
- Data Collection and Preparation: Gather relevant user data from various sources, such as surveys, interviews, and analytics platforms. Clean and prepare the data for AI processing, ensuring data consistency and accuracy.
- AI-Powered Clustering: Utilize AI clustering algorithms (e.g., k-means clustering) to group users with similar characteristics based on the collected data. The number of clusters will determine the number of personas generated.
- Persona Refinement: Review the clusters generated by the AI and refine them based on expert knowledge and additional qualitative insights. This ensures the personas accurately represent the user base.
- Persona Development: Develop detailed descriptions for each persona, including demographics, goals, motivations, frustrations, and technology usage patterns. Use the AI-generated clusters as a starting point, adding qualitative details to enrich the personas.
- Validation and Iteration: Validate the generated personas with further user research and iterative testing. This feedback loop ensures the accuracy and usefulness of the personas throughout the design process.
This AI-driven approach accelerates persona development and enhances their accuracy, leading to more effective and user-centered designs. For instance, instead of manually analyzing hundreds of survey responses to identify key user segments, AI can automate this process, providing researchers with a clear picture of distinct user groups and their needs, significantly reducing the time and effort involved.
AI-Powered Prototyping and Wireframing
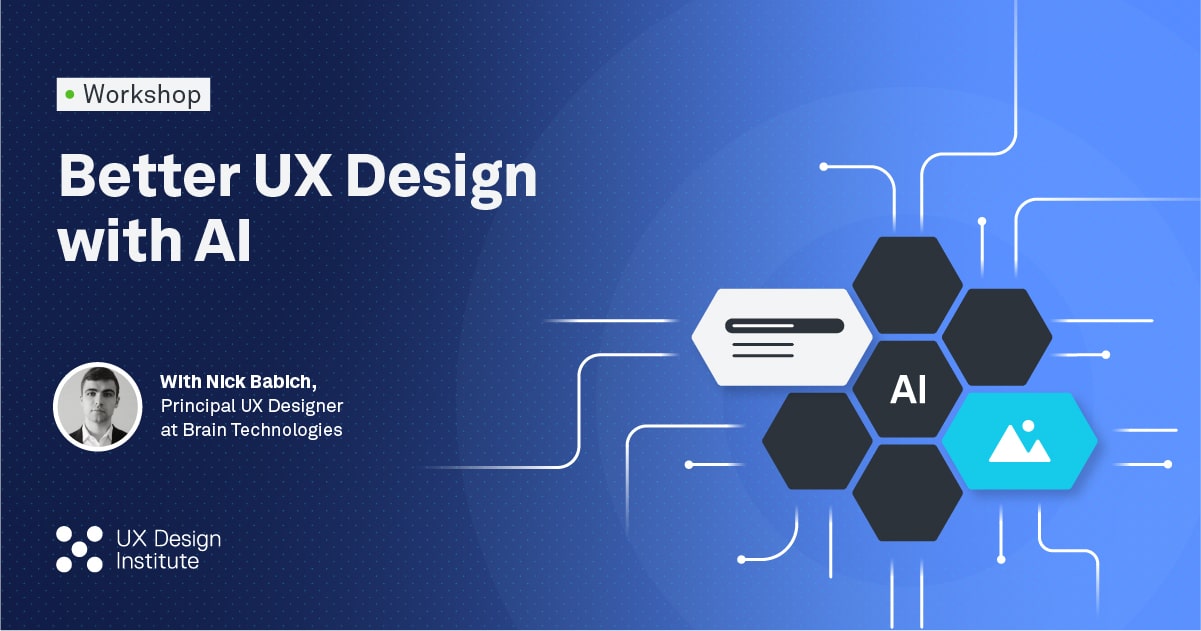
AI is rapidly transforming UI/UX design, offering powerful tools to streamline and enhance the prototyping and wireframing stages. These tools leverage machine learning to automate tasks, generate design variations, and accelerate the iterative design process, ultimately leading to faster development cycles and potentially improved user experiences. However, it’s crucial to understand both the benefits and limitations of incorporating AI into these critical phases of design.AI-powered prototyping and wireframing tools offer significant advantages over traditional methods.
The speed and efficiency gains are particularly notable. Manually creating multiple wireframes and prototypes can be time-consuming, whereas AI can generate numerous variations in a fraction of the time. This allows designers to explore a wider range of design options and rapidly test different approaches, leading to quicker iteration and improved design solutions. Furthermore, AI can analyze user data and feedback to suggest improvements and optimize designs, a process that is significantly more complex and time-consuming when performed manually.
However, the reliance on algorithms also presents limitations. AI tools may struggle with highly complex or nuanced design requirements, and the output may sometimes lack the creativity and subtle design elements that a human designer can provide. The potential for bias in the algorithms also needs careful consideration. For instance, if the training data reflects existing biases in design, the AI tool may perpetuate those biases in its generated designs.
AI-Assisted Generation of Design Variations Based on User Feedback, How to integrate AI tools into existing UI UX design processes
AI can significantly enhance the iterative design process by analyzing user feedback and generating multiple design variations accordingly. Imagine a scenario where user testing reveals a low click-through rate on a particular button. An AI-powered tool could analyze the user feedback (e.g., heatmaps, user comments, A/B test results), identify the problem areas, and automatically generate several alternative designs for that button—variations in size, color, placement, and wording.
The designer can then quickly compare these variations and select the most effective one based on the AI’s suggestions and their own expertise. This process is far more efficient than manually creating and testing multiple iterations. The AI essentially acts as an intelligent assistant, accelerating the process of design refinement and optimization. The process typically involves uploading user feedback data (e.g., from user testing platforms or surveys) into the AI tool.
The AI then processes this data, identifies patterns and insights, and uses them to generate variations of the existing design. The designer then reviews these variations, selects the most promising ones, and further refines them based on their judgment and design principles.
Integrating AI Tools for Automated Wireframe Generation
Integrating an AI tool into an existing design software, such as Figma or Sketch, typically involves using plugins or extensions. Many AI-powered design tools offer seamless integration with popular design platforms. For example, a plugin might allow designers to input basic requirements (e.g., the purpose of the screen, key elements to include, target user group) and the AI will then automatically generate a wireframe.
The designer can then further customize and refine the generated wireframe within the familiar interface of their preferred design software. This approach allows for a smooth transition and avoids the need to learn a completely new design tool. The process often begins by selecting the appropriate plugin or extension within the design software. Once installed, the designer inputs the necessary information and parameters.
The AI then processes this information and generates a wireframe. The designer can then use the design software’s editing capabilities to modify the generated wireframe to meet their specific needs and design preferences. This collaborative approach combines the speed and efficiency of AI with the creativity and control of human designers.
Leveraging AI in the Design and Iteration Phase
AI significantly accelerates and enhances the design and iteration process in UI/UX. By analyzing vast datasets of design patterns, user preferences, and accessibility guidelines, AI tools offer designers powerful capabilities to explore numerous design options efficiently and refine their creations based on data-driven insights. This leads to faster iteration cycles and ultimately, more effective and user-centered designs.AI assists designers by generating design options that adhere to specific constraints and user preferences.
This involves inputting parameters such as target audience demographics, brand guidelines, platform specifications (e.g., mobile vs. desktop), and desired functionality. The AI then proposes various design layouts, color palettes, typography choices, and interactive elements, all tailored to meet the predefined criteria. This significantly reduces the time spent on manual exploration and brainstorming, allowing designers to focus on higher-level creative decisions and strategic refinement.
AI-Powered Design Suggestion Generation
AI algorithms, trained on extensive design datasets, can analyze existing designs and identify patterns associated with high user engagement and positive feedback. This analysis allows the AI to suggest design elements, layouts, and interactions that are likely to resonate with the target audience. For instance, an AI tool might suggest specific button shapes, color combinations, or font styles known to improve click-through rates or task completion times.
This data-driven approach ensures that design decisions are informed by empirical evidence, rather than relying solely on intuition or subjective preferences. The process typically involves feeding the AI tool with design specifications, user data, and competitor analysis. The AI then generates a range of design options, each with its own rationale based on the input data. Designers can then review these suggestions, selecting the options that best align with the project’s overall goals.
Enhancing Visual Appeal and Accessibility with AI
AI can be instrumental in improving the visual appeal and accessibility of design elements. For example, AI-powered tools can automatically optimize images for web use, improving loading times and visual clarity. These tools can also analyze color palettes to ensure sufficient contrast for users with visual impairments, meeting WCAG guidelines for accessibility. Furthermore, AI can suggest font choices that enhance readability and overall aesthetic appeal, considering factors like font size, weight, and spacing.
Tools can also analyze layout designs for accessibility issues, flagging potential problems with navigation or information architecture.
Workflow for Integrating AI-Powered Design Suggestions
The integration of AI-powered design suggestions into the iterative design process can be visualized through a workflow diagram. Imagine a four-stage process:
1. Design Brief & Input
The initial phase involves defining the design brief, including target audience, functional requirements, and brand guidelines. This information is fed into the AI tool.
2. AI-Powered Design Generation
The AI processes the input and generates multiple design options, each with its underlying rationale.
3. Designer Review & Selection
Designers critically evaluate the AI-generated suggestions, selecting the options that best align with the project goals and user needs. This stage allows for human creativity and critical judgment to refine the AI’s suggestions.
4. Iteration & Refinement
Selected designs are iteratively refined based on user testing and feedback. The AI can be re-engaged to test modifications and suggest further improvements, leading to an optimized and user-centered final design.
Implementing AI for Accessibility and Inclusivity in UI/UX
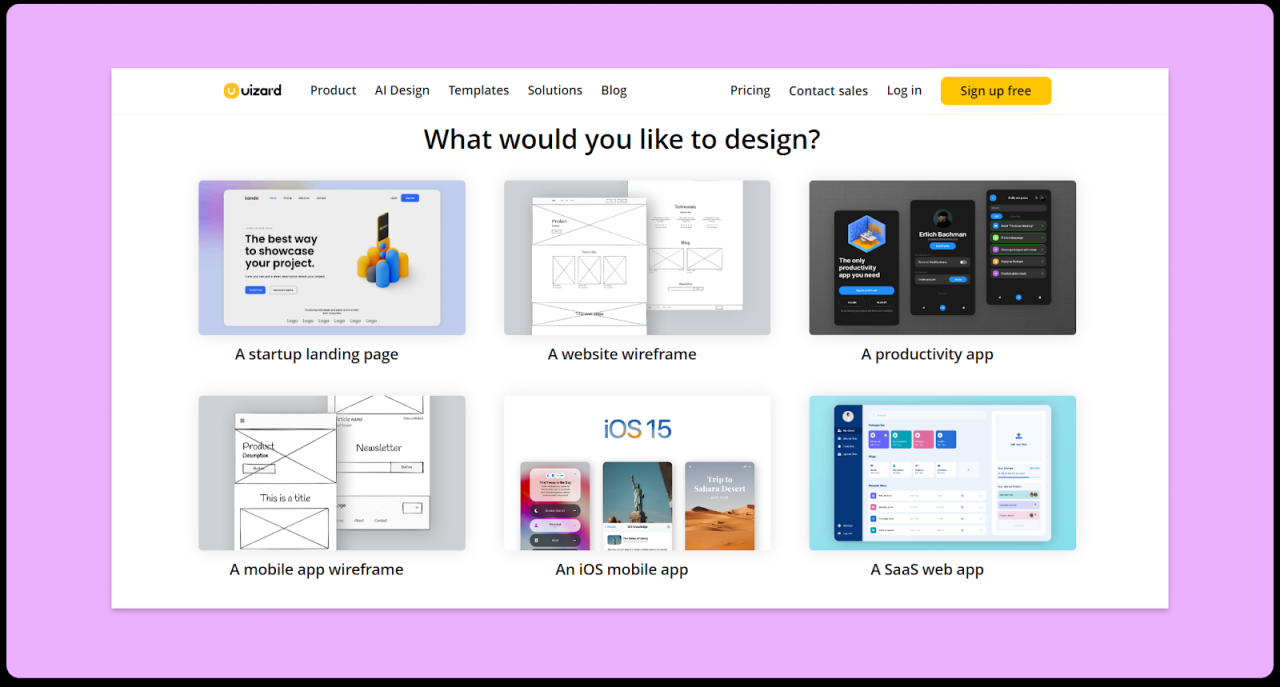
Integrating AI into UI/UX design offers a powerful opportunity to enhance accessibility and inclusivity, moving beyond traditional methods to create truly universal design experiences. AI tools can automate tasks previously requiring significant manual effort, leading to more efficient and effective accessibility implementations. Furthermore, AI’s ability to analyze vast datasets allows for the identification and mitigation of unconscious biases embedded within designs, fostering more inclusive and equitable user experiences.AI can significantly improve the accessibility and inclusivity of UI/UX design by automating accessibility checks and identifying biases.
This leads to designs that are more usable and enjoyable for a wider range of users, regardless of their abilities or backgrounds. The following sections detail specific applications of AI in achieving this.
Automated Accessibility Checks and WCAG Compliance
AI-powered tools can analyze designs for compliance with Web Content Accessibility Guidelines (WCAG) standards. These tools automatically assess aspects such as color contrast ratios, alternative text for images, keyboard navigation functionality, and proper heading structure. For example, an AI tool could analyze a website’s color palette and flag instances where the contrast between text and background colors falls below WCAG’s minimum requirements.
This automated analysis significantly reduces the time and effort required for manual accessibility audits, allowing designers to address accessibility issues proactively throughout the design process, not just as a final step. The speed and accuracy offered by AI ensures a higher degree of WCAG compliance, resulting in more accessible digital products.
AI-Driven Bias Detection and Mitigation in Design
AI algorithms can be trained on large datasets of user interactions and design elements to identify potential biases embedded in designs. For instance, AI can detect if imagery predominantly features one demographic group, potentially excluding others. Similarly, AI can analyze language used in microcopy and identify potentially discriminatory or exclusionary wording. By identifying these biases, designers can proactively address them, creating more inclusive and representative designs.
This process moves beyond simple compliance checks to a more nuanced understanding of how design choices can perpetuate existing inequalities. For example, an AI tool might flag a design that uses predominantly light-skinned models in its imagery, prompting the designer to consider a more diverse representation.
Checklist for Evaluating Accessibility Features of AI-Generated Designs
Before deploying AI-generated designs, a thorough review is crucial to ensure accessibility and inclusivity. This checklist aids in evaluating the output of AI design tools:
It’s essential to critically examine AI-generated designs, ensuring that the automation doesn’t inadvertently introduce accessibility problems or perpetuate existing biases. A thorough review process, guided by this checklist, is vital for responsible and effective use of AI in UI/UX design.
Aspect | Checklist Item | Example |
---|---|---|
Color Contrast | Verify sufficient contrast between text and background colors according to WCAG guidelines. | Check all text elements against WCAG AA or AAA contrast ratios using a tool like WebAIM’s contrast checker. |
Alternative Text | Ensure all non-text content (images, videos) has accurate and descriptive alternative text. | Verify that alternative text conveys the meaning and purpose of the image for screen reader users. |
Keyboard Navigation | Confirm all interactive elements are accessible via keyboard navigation. | Test navigation using only the Tab key to ensure all elements are reachable and have proper focus indicators. |
Heading Structure | Validate proper use of heading levels (H1-H6) to create a logical document structure. | Ensure headings are used consistently and logically to organize content for screen reader users. |
Form Accessibility | Check that forms are accessible, with clear labels, appropriate input types, and error handling. | Verify that form fields have clear labels and instructions, and that error messages are informative and easy to understand. |
Image Diversity | Assess the diversity of imagery used, ensuring representation of different genders, ethnicities, and abilities. | Review imagery to ensure it avoids stereotypical or exclusionary representations. |
Language Inclusivity | Review all text for inclusive language, avoiding jargon, slang, and potentially offensive terms. | Ensure language is clear, concise, and avoids assumptions about the user’s background or abilities. |
Addressing Ethical Considerations and Potential Challenges
Integrating AI into UI/UX design offers significant advantages, but it also introduces ethical concerns that require careful consideration. Ignoring these potential pitfalls can lead to biased designs, privacy violations, and a diminished user experience. A responsible approach necessitates proactive mitigation strategies and a commitment to human oversight throughout the design process.The use of AI in UI/UX design presents several ethical challenges that designers must actively address.
These challenges are not insurmountable, but require a thoughtful and proactive approach, ensuring ethical considerations are prioritized alongside functional and aesthetic goals.
Data Privacy Concerns in AI-Driven UI/UX Design
AI tools often rely on vast datasets for training and operation. This data may include sensitive user information, raising concerns about privacy and data security. For instance, an AI tool analyzing user behavior to personalize a website’s design could inadvertently collect and process personally identifiable information (PII) without explicit consent. To mitigate this risk, designers must ensure compliance with relevant data protection regulations (like GDPR and CCPA), implement robust data anonymization techniques, and obtain informed consent from users before collecting and using their data.
Transparency regarding data collection and usage practices is paramount to building trust and maintaining user confidence. This includes clearly outlining how user data is used to inform design decisions and providing users with control over their data.
Algorithmic Bias in AI-Generated Designs
AI algorithms are trained on data, and if that data reflects existing societal biases, the resulting AI-generated designs may perpetuate or even amplify those biases. For example, an AI tool trained on images primarily featuring individuals from a specific demographic might generate designs that inadvertently exclude or misrepresent other demographics. This can lead to designs that are not inclusive or accessible to all users.
To address this, designers must carefully curate the datasets used to train AI tools, ensuring representation across diverse demographics and avoiding skewed data that could lead to biased outcomes. Regular audits of AI-generated designs for potential bias are crucial, and human oversight is essential to identify and correct any biases that may emerge.
Maintaining Human Oversight and Creative Control
While AI tools can automate certain aspects of the UI/UX design process, human oversight remains crucial. Relying solely on AI-generated designs risks losing the human element—the empathy, creativity, and critical thinking that are essential for crafting truly user-centered designs. Human designers should retain ultimate control over the design process, using AI tools as aids rather than replacements. This involves critically evaluating AI-generated suggestions, ensuring they align with design goals, and incorporating human intuition and expertise to refine and enhance the final product.
The balance between AI assistance and human creativity is vital for creating innovative and ethically sound designs.
Mitigation Strategies for AI-Related Risks
Several strategies can help mitigate the risks associated with AI in UI/UX design. These include rigorous testing and validation of AI-generated designs to ensure they meet usability and accessibility standards; implementing robust security measures to protect user data; and establishing clear ethical guidelines and protocols for the use of AI in the design process. Furthermore, ongoing education and training for designers on the ethical implications of AI are essential to foster responsible and ethical practices.
Regular audits and reviews of AI tools and their outputs can help identify and address potential biases and vulnerabilities. Collaboration between designers, AI developers, and ethicists is vital to develop and implement effective mitigation strategies.
Final Wrap-Up
Integrating AI into your UI/UX design process isn’t about replacing human creativity but augmenting it. By strategically incorporating AI tools, designers can free themselves from tedious tasks, explore a wider range of design possibilities, and ultimately create more user-centered and inclusive products. Remember, ethical considerations and human oversight are paramount. Embrace the potential of AI, but always maintain a human-centered approach to ensure your designs remain innovative, effective, and responsible.