The ethical considerations of increasingly prevalent AI technologies demand urgent attention. As artificial intelligence rapidly integrates into every facet of modern life, from healthcare and finance to law enforcement and warfare, a critical examination of its potential societal impact becomes paramount. This exploration delves into the complex moral dilemmas posed by algorithmic bias, privacy violations, job displacement, autonomous weapons, and the environmental consequences of AI development.
Understanding these challenges is crucial for navigating the future responsibly and ensuring AI benefits humanity equitably.
This analysis will unpack the multifaceted ethical implications of AI, offering a comprehensive overview of current debates and proposing potential solutions. We will examine real-world examples of AI’s impact, explore existing regulatory frameworks, and suggest strategies for mitigating potential harms. The goal is to foster informed discussion and responsible innovation in the field of artificial intelligence.
Bias and Discrimination in AI
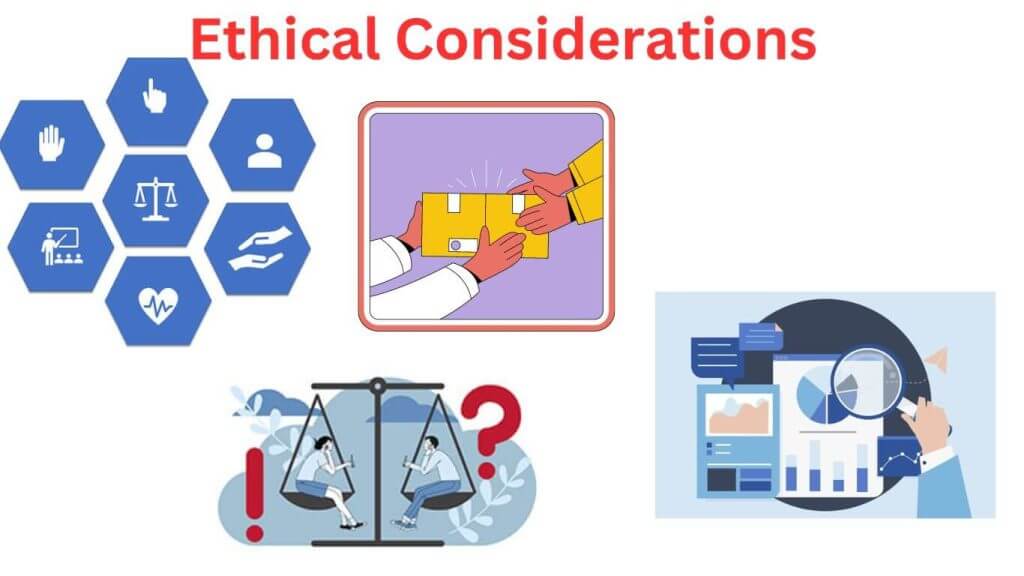
The increasing prevalence of artificial intelligence (AI) systems across various sectors raises significant ethical concerns, particularly regarding bias and discrimination. Algorithmic bias, stemming from flawed data or design, can perpetuate and exacerbate existing societal inequalities, leading to unfair or discriminatory outcomes for certain groups. Understanding the nature of this bias, the methods for its detection and mitigation, and the development of robust fairness frameworks are crucial for responsible AI development and deployment.
Algorithmic Bias and Societal Inequalities
Algorithmic bias arises when AI systems reflect and amplify existing societal biases present in the data used to train them. This can manifest in various ways, such as biased predictions, unfair resource allocation, and discriminatory decision-making. For example, if a facial recognition system is trained primarily on images of individuals from a specific demographic, it may perform poorly when identifying individuals from other demographics, potentially leading to misidentification and wrongful accusations.
Similarly, biased algorithms used in loan applications or hiring processes can disproportionately disadvantage certain groups based on race, gender, or socioeconomic status, further entrenching existing inequalities. The consequences of such biases can be severe, impacting individuals’ access to opportunities, resources, and justice.
Identifying and Mitigating Bias in AI Training Data
Several methods can be employed to identify and mitigate bias in AI training data. Firstly, careful data auditing is essential to uncover potential biases. This involves examining the data for imbalances in representation across different demographic groups and identifying any systematic errors or biases. Techniques like statistical analysis and visualization can be used to detect such imbalances. Secondly, data preprocessing techniques can be applied to rebalance the dataset and address identified biases.
This might involve oversampling underrepresented groups, undersampling overrepresented groups, or using techniques like data augmentation to create synthetic data that addresses imbalances. Thirdly, the choice of algorithms and model architectures can influence the extent to which biases are reflected in the final system. Using algorithms that are less susceptible to bias, and incorporating fairness constraints into the model training process, can help to mitigate bias.
Real-World Examples of AI Bias Leading to Discriminatory Outcomes
Numerous real-world applications illustrate the consequences of AI bias. For example, studies have shown that facial recognition systems exhibit higher error rates for individuals with darker skin tones, potentially leading to misidentification and wrongful arrests. Similarly, AI-powered loan applications have been found to discriminate against applicants from certain racial or socioeconomic backgrounds, denying them access to credit. In the criminal justice system, AI-based risk assessment tools have been criticized for perpetuating racial biases, leading to disproportionately harsher sentences for minority groups.
These examples highlight the urgent need for robust methods to identify and mitigate bias in AI systems.
Framework for Evaluating the Fairness and Equity of AI Systems
A comprehensive framework for evaluating the fairness and equity of AI systems should encompass multiple dimensions. This includes assessing the accuracy and fairness of predictions across different demographic groups, analyzing the impact of the system on various stakeholders, and examining the transparency and explainability of the system’s decision-making process. Key metrics for evaluation could include demographic parity, equal opportunity, and predictive rate parity.
Furthermore, the framework should incorporate mechanisms for continuous monitoring and auditing of the system’s performance, allowing for prompt identification and mitigation of emerging biases. Stakeholder engagement and feedback mechanisms are also crucial for ensuring that the system is aligned with societal values and does not perpetuate harm.
Bias Mitigation Techniques
Technique | Strengths | Weaknesses | Example |
---|---|---|---|
Data Preprocessing (Re-weighting) | Relatively simple to implement; can improve overall fairness | May not fully address complex biases; can lead to information loss | Adjusting weights in the training data to over-represent underrepresented groups. |
Adversarial Debiasing | Can effectively mitigate bias without sacrificing accuracy | Can be computationally expensive; requires careful design and tuning | Training a separate model to identify and counteract bias in the main model. |
Fairness-Aware Algorithms | Incorporates fairness constraints directly into the model training process | Can be complex to implement; may require specialized expertise | Using algorithms that explicitly optimize for fairness metrics, such as equal opportunity. |
Explainable AI (XAI) | Increases transparency and allows for better understanding of model decisions | Can be challenging to implement for complex models; interpretability may be limited | Using techniques like LIME or SHAP to explain individual predictions. |
Privacy and Surveillance
The increasing prevalence of AI technologies, particularly in surveillance applications, raises significant ethical concerns regarding individual privacy and the potential for misuse. AI-powered surveillance systems, such as facial recognition and predictive policing algorithms, offer enhanced security capabilities, but they also present substantial risks to fundamental rights and freedoms. This section explores the ethical implications of these technologies, examining the challenges of balancing security needs with individual privacy rights and highlighting potential abuses and necessary regulatory frameworks.AI-powered surveillance technologies are transforming the landscape of security and law enforcement.
Facial recognition systems, for instance, can identify individuals in real-time from video feeds, while predictive policing algorithms use data analysis to anticipate potential crime hotspots. These technologies promise increased efficiency and effectiveness in crime prevention and detection. However, their deployment necessitates a careful consideration of the ethical implications associated with the potential erosion of privacy and the risk of biased outcomes.
Ethical Implications of AI-Powered Surveillance
The ethical implications of AI-powered surveillance are multifaceted. The potential for mass surveillance, where individuals are constantly monitored without their knowledge or consent, raises serious concerns about the chilling effect on freedom of expression and assembly. The use of facial recognition technology, for example, can be employed to track individuals’ movements and activities, potentially leading to the creation of comprehensive behavioral profiles.
Furthermore, the accuracy and fairness of these systems are questionable, particularly concerning their impact on marginalized communities. Inaccurate or biased algorithms can lead to misidentification and wrongful accusations, exacerbating existing societal inequalities.
Balancing Security Concerns with Individual Privacy Rights, The ethical considerations of increasingly prevalent AI technologies
Balancing security concerns with individual privacy rights in the age of AI is a complex challenge. While enhanced security measures are undoubtedly beneficial, the potential for abuse and the erosion of privacy necessitates a cautious approach. Effective regulations and robust oversight mechanisms are crucial to ensure that AI-powered surveillance technologies are deployed responsibly and ethically. This requires a clear definition of acceptable uses of these technologies, coupled with stringent data protection measures and mechanisms for accountability and redress.
The challenge lies in finding a balance that ensures public safety without sacrificing fundamental rights and freedoms.
Potential Abuses of AI Surveillance Technologies
Several documented instances demonstrate the potential for abuse of AI surveillance technologies. In some cases, facial recognition systems have been shown to exhibit racial and gender biases, leading to disproportionate targeting of certain groups. Predictive policing algorithms, which often rely on historical crime data, can perpetuate existing biases and lead to increased policing in already marginalized communities. The lack of transparency and accountability in the development and deployment of these systems further exacerbates the risks of misuse.
Examples include the use of facial recognition technology to track protesters or to monitor individuals without their knowledge or consent.
Legal and Regulatory Frameworks Addressing AI Privacy Concerns
Several legal and regulatory frameworks are emerging to address privacy concerns related to AI. The European Union’s General Data Protection Regulation (GDPR) provides a comprehensive framework for data protection, including provisions related to automated decision-making and profiling. Other jurisdictions are developing similar regulations, often focusing on transparency, data minimization, and accountability. However, the rapid pace of technological development poses a challenge for regulators, who must adapt quickly to address emerging issues.
International cooperation is also essential to establish consistent standards and prevent regulatory arbitrage.
Best Practices for Responsible Data Collection and Use in AI Systems
Responsible data collection and use are crucial for mitigating the ethical risks associated with AI-powered surveillance. Best practices include:
- Obtaining informed consent before collecting and using personal data.
- Implementing data minimization principles, collecting only the data necessary for the intended purpose.
- Ensuring data accuracy and security through appropriate technical and organizational measures.
- Regularly auditing AI systems for bias and ensuring fairness and transparency.
- Establishing mechanisms for accountability and redress in case of errors or misuse.
These best practices, combined with robust legal and regulatory frameworks, are essential for ensuring that AI-powered surveillance technologies are deployed responsibly and ethically, protecting individual privacy rights while maintaining public safety.
Job Displacement and Economic Inequality
The increasing prevalence of AI technologies presents a complex challenge: while offering unprecedented productivity gains, it simultaneously threatens widespread job displacement and exacerbates existing economic inequalities. Understanding the nuanced interplay between AI-driven automation and the labor market is crucial for developing effective mitigation strategies and ensuring a just transition to an AI-powered future. This section explores the potential benefits and drawbacks of AI automation across various sectors, analyzes its impact on income inequality, and proposes a plan for mitigating the negative consequences for workers and communities.AI-driven automation offers significant potential benefits, such as increased efficiency, reduced production costs, and the creation of new, higher-skilled jobs.
However, these benefits are not evenly distributed. The drawbacks include the displacement of workers in various sectors, particularly those involving routine or repetitive tasks, leading to unemployment and potentially widening the gap between the wealthy and the working class. This necessitates a proactive approach to manage the transition and ensure a fairer distribution of the economic gains generated by AI.
AI Automation’s Impact Across Sectors
The impact of AI-driven automation varies significantly across different sectors. Sectors heavily reliant on manual or repetitive tasks, such as manufacturing, transportation, and customer service, are particularly vulnerable to job displacement. For example, the rise of automated vehicles threatens the livelihoods of millions of truck drivers and taxi drivers globally. Conversely, sectors requiring high levels of creativity, critical thinking, and complex problem-solving, such as healthcare, education, and research, are likely to experience less disruption and potentially see the creation of new roles supporting AI systems.
However, even in these sectors, AI could automate certain tasks, requiring adaptation and reskilling of the workforce.
AI’s Influence on Income Inequality
The introduction of AI technologies has the potential to significantly exacerbate existing income inequality. While AI-driven automation can boost overall productivity and economic growth, the benefits are often concentrated among a small segment of the population, primarily those who own or control the AI systems and reap the profits from increased efficiency. This creates a widening gap between high-skilled, high-income workers who manage and develop AI systems and low-skilled, low-income workers whose jobs are automated.
The resulting unemployment and wage stagnation among lower-skilled workers can lead to social unrest and economic instability. For instance, the automation of factory jobs in developed countries has already contributed to the decline of the manufacturing sector’s workforce and the growth of income inequality.
Economic and Social Consequences of Widespread Job Displacement
Widespread job displacement due to AI can have severe economic and social consequences. Increased unemployment leads to decreased consumer spending, reduced tax revenue, and increased demand for social welfare programs. The social consequences can include increased poverty, social unrest, and a decline in overall well-being. Studies have shown a correlation between job losses due to automation and increased rates of mental health issues and social isolation.
For example, the decline of coal mining communities due to automation has demonstrated the profound and long-lasting social and economic impact of job displacement on entire communities.
Mitigating the Negative Economic Impacts of AI
Mitigating the negative economic impacts of AI requires a multi-pronged approach involving government policies, industry initiatives, and individual adaptation. This includes investing heavily in education and retraining programs to equip workers with the skills needed for the jobs of the future. Furthermore, exploring alternative economic models, such as universal basic income (UBI), could provide a safety net for those displaced by automation.
Finally, strong regulations are needed to ensure responsible AI development and deployment, preventing the exacerbation of existing inequalities. Examples of successful initiatives include government-funded retraining programs focusing on in-demand AI-related skills, and company-led programs providing upskilling opportunities for their existing workforce.
Strategies for a Just Transition to an AI-Driven Economy
The transition to an AI-driven economy requires careful planning and proactive strategies to ensure a just and equitable outcome.
- Invest in Education and Retraining: Develop comprehensive programs to reskill and upskill workers displaced by automation, focusing on skills in high demand in the AI-driven economy.
- Promote Lifelong Learning: Create a culture of continuous learning and adaptation, enabling workers to acquire new skills throughout their careers.
- Explore Alternative Economic Models: Consider implementing policies like UBI or negative income tax to provide a safety net for those displaced by automation.
- Support Small and Medium-Sized Enterprises (SMEs): Provide resources and support to SMEs to help them adopt AI technologies and remain competitive.
- Regulate AI Development and Deployment: Implement regulations to ensure responsible AI development and deployment, minimizing the risk of job displacement and bias.
- Invest in Infrastructure: Develop the necessary infrastructure to support the AI-driven economy, including high-speed internet access and advanced computing capabilities.
- Foster Collaboration: Encourage collaboration between government, industry, and academia to develop effective strategies for managing the transition.
Autonomous Weapons Systems
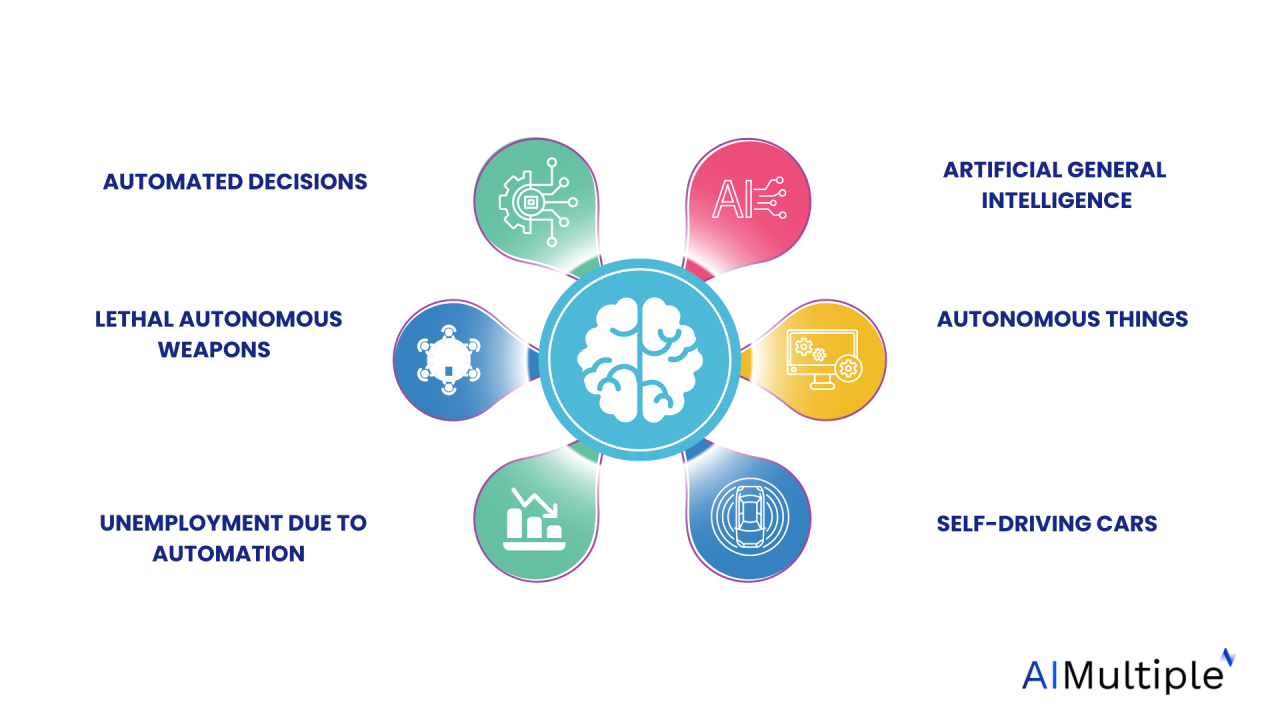
The development and deployment of lethal autonomous weapons systems (LAWS), also known as killer robots, present a complex web of ethical dilemmas that challenge our understanding of warfare, accountability, and the very nature of humanity. These systems, capable of selecting and engaging targets without human intervention, raise profound questions about the future of conflict and the potential for catastrophic unintended consequences.
This section will delve into the key ethical considerations surrounding LAWS, examining the challenges of establishing responsibility, the arguments for and against international regulation, and the potential for humanitarian crises.
Accountability and Responsibility for LAWS Actions
Establishing clear lines of accountability for the actions of LAWS presents a significant hurdle. Unlike traditional warfare, where individual soldiers and commanders can be held responsible for their actions, the decentralized nature and autonomous decision-making capabilities of LAWS blur these lines. Determining who is ultimately responsible—the programmers, the manufacturers, the deploying state, or the AI itself—remains a contentious and unresolved issue.
This lack of clear accountability raises serious concerns about the potential for misuse and the difficulty in holding anyone accountable for harm caused by these weapons. The absence of human judgment in the targeting and engagement process introduces a high risk of errors and unintended harm, making the pursuit of accountability crucial. Legal frameworks and international agreements will need significant revision to adequately address this challenge.
Arguments For and Against International Regulation or Prohibition of LAWS
The debate surrounding the international regulation or prohibition of LAWS is fiercely contested. Proponents of a ban argue that the inherent unpredictability and potential for escalation of LAWS pose an unacceptable risk to global security and human life. They emphasize the ethical implications of delegating life-or-death decisions to machines, potentially violating fundamental human rights. Conversely, those who oppose a ban argue that LAWS could offer advantages in terms of precision, reduced collateral damage, and the protection of soldiers from harm.
They also highlight the potential for LAWS to deter aggression and maintain stability in certain conflict scenarios. However, this perspective often fails to adequately address the crucial issues of accountability and the potential for unintended consequences. The ongoing international discussions and negotiations on this topic reflect the profound division of opinion and the urgency of finding a global consensus.
Potential Scenarios Leading to Unintended Consequences or Humanitarian Crises
Several scenarios illustrate the potential for LAWS to lead to unintended consequences and humanitarian crises. A malfunctioning system could, for instance, mistakenly target civilians, leading to mass casualties. The use of LAWS in complex or ambiguous environments could result in escalating conflicts, as the absence of human judgment may lead to misinterpretations and miscalculations. Moreover, the potential for autonomous weapons to fall into the wrong hands—terrorist organizations or rogue states—presents a grave threat to global security.
The development of sophisticated countermeasures could also lead to an arms race, increasing instability and escalating the risk of widespread conflict. The potential for autonomous systems to be used for targeted assassinations or other forms of extrajudicial killings further heightens ethical concerns.
Technical and Ethical Considerations in LAWS Safety Protocols
The design and implementation of robust safety protocols for LAWS are paramount. Technically, this involves developing fail-safes, verification mechanisms, and human-in-the-loop controls to ensure that the systems operate as intended and minimize the risk of unintended harm. Ethically, this requires careful consideration of the values and principles that should guide the development and deployment of these weapons. Transparency, accountability, and the protection of human life should be central to the design process.
The creation of independent oversight bodies to monitor the development and use of LAWS is also crucial. The challenge lies in balancing the desire for effective military capabilities with the need to safeguard against the catastrophic risks associated with these powerful technologies. This requires a multi-faceted approach that integrates technological safeguards with strong ethical guidelines and international regulations.
Transparency and Explainability
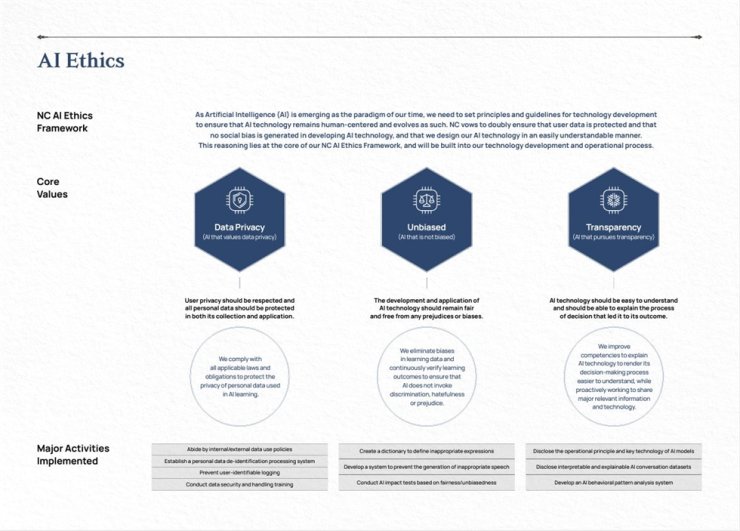
The increasing prevalence of AI systems in high-stakes decision-making necessitates a critical examination of transparency and explainability. These principles are crucial for building trust, ensuring accountability, and identifying potential biases or errors within AI models. Without understanding how an AI system arrives at a particular conclusion, it becomes difficult to assess its reliability and fairness, especially in areas like healthcare, finance, and criminal justice.The Importance of Transparency and Explainability in High-Stakes Decision-Making Contexts.
Transparency in AI refers to the ability to understand the internal workings of a model, while explainability focuses on providing comprehensible explanations for its decisions. In high-stakes situations, such as loan applications or medical diagnoses, understanding the reasoning behind an AI’s decision is paramount. This allows for human oversight, the identification of potential biases, and the opportunity to correct errors or improve the system’s performance.
Lack of transparency can lead to mistrust, unfair outcomes, and difficulty in identifying and rectifying systemic issues.
Challenges in Making Complex AI Models Understandable to Humans
Many advanced AI models, particularly deep learning networks, are notoriously “black boxes.” Their complexity arises from the intricate interactions of numerous layers and parameters, making it difficult to trace the decision-making process. The sheer number of variables and the non-linear nature of these models make it challenging to extract meaningful explanations. Furthermore, the data used to train these models often contains implicit biases that are difficult to detect and isolate, further complicating the process of understanding their output.
For example, a facial recognition system trained on a dataset predominantly featuring light-skinned individuals might exhibit lower accuracy when identifying individuals with darker skin tones, a bias that might be difficult to discern from simply analyzing the model’s architecture or weights.
Techniques for Enhancing Transparency and Explainability
Several techniques aim to improve the transparency and explainability of AI systems. These include: Local Interpretable Model-agnostic Explanations (LIME), which approximates the behavior of a complex model locally around a specific prediction; SHapley Additive exPlanations (SHAP), which uses game theory to assign importance scores to input features; and attention mechanisms, which highlight the parts of the input data that the model focuses on during decision-making.
These methods provide insights into the factors influencing AI decisions, enabling humans to understand and interpret the model’s behavior more effectively. For instance, in medical image analysis, attention mechanisms can highlight the specific regions of an image that contributed to a diagnosis, improving the confidence and understanding of medical professionals.
A Method for Evaluating the Level of Transparency and Explainability
A comprehensive evaluation of transparency and explainability should consider several factors. A scoring system could be developed, incorporating metrics such as: (1) the complexity of the model’s architecture, (2) the availability of documentation and internal workings, (3) the ease of interpreting explanations generated by XAI techniques, (4) the accuracy of these explanations, and (5) the ability of human experts to understand and validate the model’s decisions.
Each factor could be assigned a score (e.g., on a scale of 1-5), with a weighted average determining the overall transparency and explainability score. This approach would provide a quantitative assessment of the model’s interpretability and facilitate comparison across different AI systems.
Comparison of Different Approaches to Explainable AI (XAI)
Approach | Strengths | Limitations | Example Application |
---|---|---|---|
LIME | Model-agnostic, locally interpretable | Approximation can be inaccurate, depends on the quality of local surrogate model | Credit risk assessment |
SHAP | Provides feature importance scores, based on game theory | Computationally expensive for large datasets | Medical diagnosis |
Attention Mechanisms | Provides insights into model’s focus, directly incorporated into model architecture | Not applicable to all model types, interpretation may still be challenging | Image classification |
Rule Extraction | Provides easily understandable rules, works well with simpler models | Difficult to apply to complex models, may oversimplify the decision process | Fraud detection |
Environmental Impact of AI
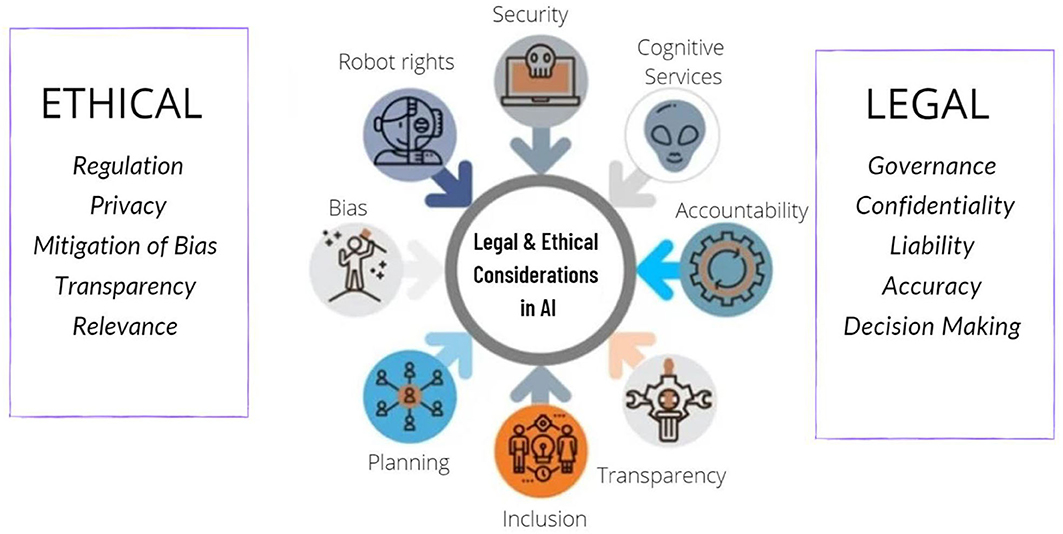
The rapid advancement and deployment of artificial intelligence (AI) technologies are increasingly raising concerns about their environmental footprint. While AI offers solutions to various environmental challenges, its development and operation consume significant resources, leading to substantial energy consumption and waste generation. Understanding this dual nature – AI’s potential for environmental good and its inherent environmental cost – is crucial for responsible innovation and deployment.The environmental footprint of AI encompasses several key areas.
Energy consumption is a major factor, particularly with the rise of large language models and deep learning algorithms that require immense computational power. The manufacturing of AI hardware, including data centers and specialized chips, also contributes significantly to resource depletion and pollution. Furthermore, the disposal of obsolete hardware generates electronic waste (e-waste), posing significant environmental and health risks if not managed properly.
These environmental impacts must be carefully considered alongside the benefits AI offers.
AI’s Energy Consumption and Waste Generation
The training of sophisticated AI models, especially deep learning models, demands substantial computational power, translating to high energy consumption. Data centers housing these models require massive amounts of electricity, often sourced from non-renewable energy sources. For example, the training of a single large language model can consume as much energy as several hundred homes over a year. This energy demand contributes to greenhouse gas emissions and exacerbates climate change.
Furthermore, the manufacturing process of AI hardware, involving the extraction of rare earth minerals and complex manufacturing processes, contributes to pollution and resource depletion. The eventual disposal of these devices adds to the growing problem of e-waste, which contains hazardous materials requiring careful and specialized recycling.
AI’s Potential for Environmental Sustainability
Despite its own environmental impact, AI holds significant potential for contributing to environmental sustainability. AI-powered climate modeling can improve our understanding of climate change, enabling more accurate predictions and informing mitigation strategies. AI can optimize resource management in various sectors, such as agriculture and transportation, leading to reduced resource consumption and waste. For example, AI-driven precision agriculture can optimize irrigation and fertilizer use, reducing water consumption and minimizing environmental damage.
Similarly, AI-powered smart grids can improve energy distribution efficiency, reducing energy waste and promoting the integration of renewable energy sources.
Environmentally Conscious AI Development Practices
Several strategies are being explored to mitigate the environmental impact of AI. These include the development of more energy-efficient algorithms, the use of renewable energy sources to power data centers, and the implementation of sustainable hardware manufacturing processes. Researchers are actively working on developing algorithms that require less computational power while maintaining performance. Initiatives are underway to transition data centers to renewable energy sources, reducing their carbon footprint.
The adoption of circular economy principles in the design and manufacturing of AI hardware can also minimize waste generation and resource consumption. Companies are increasingly adopting carbon offsetting programs to neutralize the environmental impact of their AI operations.
Strategies for Reducing the Environmental Impact of AI
Reducing the environmental impact of AI requires a multi-pronged approach. This includes investing in research and development of energy-efficient algorithms and hardware, promoting the use of renewable energy sources for data centers, implementing robust e-waste management systems, and developing stricter environmental regulations for the AI industry. Furthermore, encouraging the development and adoption of AI applications that directly contribute to environmental sustainability is crucial.
This requires collaboration between researchers, policymakers, and industry stakeholders to establish clear guidelines and incentives for environmentally responsible AI development and deployment. Public awareness campaigns can also play a significant role in promoting sustainable AI practices.
Lifecycle of an AI System: Environmental Considerations
The environmental impact of an AI system spans its entire lifecycle, from design and manufacturing to deployment and disposal. During the design phase, choices regarding algorithm efficiency and hardware specifications significantly influence energy consumption. The manufacturing phase involves resource extraction, processing, and assembly, generating waste and emissions. Deployment involves energy consumption for operation and maintenance. Finally, disposal generates e-waste, posing environmental and health risks.
Each stage requires careful consideration of its environmental footprint to minimize its impact. Adopting a circular economy approach, emphasizing reuse, repair, and recycling, is crucial in minimizing the environmental impact throughout the entire lifecycle.
Closing Notes: The Ethical Considerations Of Increasingly Prevalent AI Technologies
The ethical considerations surrounding increasingly prevalent AI technologies are not merely theoretical; they are deeply intertwined with the present and future of society. Addressing bias, protecting privacy, mitigating job displacement, regulating autonomous weapons, and minimizing environmental impact are not optional but essential for harnessing AI’s potential while safeguarding human values. A collaborative effort involving policymakers, researchers, developers, and the public is crucial to ensure that AI serves humanity ethically and equitably.
The path forward requires ongoing dialogue, robust regulation, and a commitment to responsible innovation.