The ethical implications of AI-generated design assets are rapidly becoming a central concern for designers, businesses, and consumers alike. As artificial intelligence increasingly permeates the creative landscape, questions of copyright, bias, job displacement, and environmental impact demand careful consideration. This exploration delves into the complex moral and legal ramifications of utilizing AI in design, examining the potential benefits and drawbacks of this transformative technology.
From the murky legal waters surrounding ownership of AI-generated work to the subtle biases embedded within algorithms, the challenges are multifaceted. We will investigate how AI-generated designs might inadvertently perpetuate harmful stereotypes or exclude underrepresented groups, and explore strategies for mitigating these risks. Furthermore, we’ll analyze the evolving role of human designers in an AI-driven world, considering both the potential for job displacement and the emergence of new collaborative opportunities.
Copyright and Ownership of AI-Generated Designs: The Ethical Implications Of AI-generated Design Assets
The burgeoning field of AI-generated design assets presents a significant legal challenge: determining copyright and ownership. Unlike traditional design work, where the creator holds clear copyright, the lack of a human author in AI-generated designs creates a complex legal grey area with far-reaching implications for designers and businesses alike. This section will explore the uncertainties surrounding ownership and potential legal disputes arising from the use of AI-generated assets.The legal landscape surrounding AI-generated designs is largely uncharted territory.
Current copyright laws are primarily designed for human authorship, focusing on the originality and creative expression of the creator. AI, however, lacks the capacity for independent thought and creative intention, raising questions about whether AI-generated outputs can be considered “works of authorship” eligible for copyright protection. This ambiguity creates significant risk for those who create, use, or distribute such assets.
Copyright Protection for AI-Generated Designs
Several scenarios highlight the unclear ownership of AI-generated designs. Consider a designer using an AI tool to generate a logo. Does the copyright belong to the designer who prompted the AI, the company that developed the AI software, or does the work fall into the public domain? Similarly, if two different users input similar prompts into the same AI tool, resulting in near-identical designs, who holds the copyright, and can one sue the other for infringement?
These are just a few of the many scenarios that lack clear legal precedent.
Potential Legal Disputes Involving AI-Generated Designs
The potential for legal disputes is substantial. Businesses using AI-generated assets in marketing campaigns or product designs could face infringement claims if the designs are found to be substantially similar to existing copyrighted works. Conversely, designers who invest time and effort prompting AI tools could find their work infringed upon if others use similar prompts to generate comparable designs.
These disputes could involve costly litigation, potentially impacting the viability of businesses and the careers of designers.
Implications for Designers and Businesses
For designers, the uncertainty surrounding copyright presents a significant challenge. The ability to easily generate designs using AI could lead to a devaluation of their skills and expertise, while simultaneously increasing the risk of legal repercussions for using or distributing AI-generated assets. Businesses face the risk of unknowingly infringing on copyright, leading to financial penalties and reputational damage.
Clear guidelines and legal frameworks are urgently needed to navigate this evolving landscape.
Comparison of Traditional and AI-Generated Design Copyright
Aspect | Traditional Design | AI-Generated Design | Legal Uncertainty |
---|---|---|---|
Authorship | Clearly defined human creator | Unclear; potentially the AI developer, the user, or no one | High |
Originality | Requires originality and creative expression | Originality is debatable; depends on the level of user input and AI’s capabilities | High |
Copyright Ownership | Creator typically owns copyright | No clear legal precedent; potential for multiple claims | Very High |
Infringement | Clear legal framework for determining infringement | Lack of clear framework; difficult to prove or defend against infringement claims | High |
Bias and Representation in AI-Generated Designs
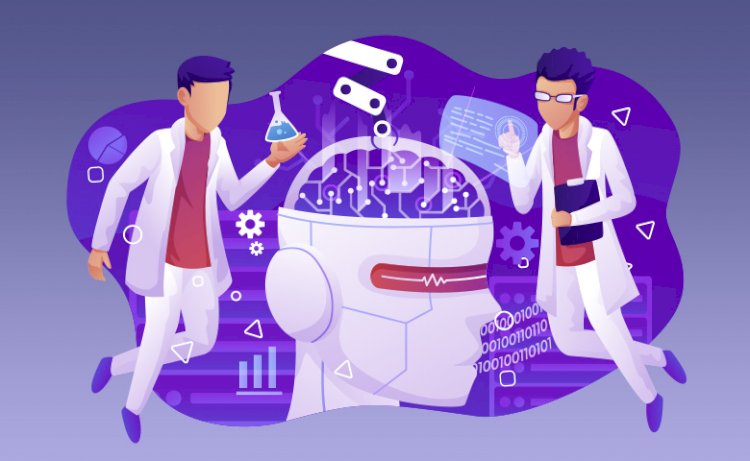
The increasing use of AI in design raises critical concerns about bias and representation. AI models are trained on vast datasets, and if these datasets reflect existing societal biases, the AI will inevitably perpetuate and even amplify those biases in its generated designs. Understanding and mitigating these biases is crucial for ensuring fairness, inclusivity, and ethical responsibility in the application of AI design tools.AI-generated designs are susceptible to biases present in the training data.
These biases can stem from various sources, including underrepresentation of certain demographics in the dataset, skewed data reflecting societal prejudices, or even biases introduced during the data collection and curation process. For instance, if a dataset primarily features images of Caucasian faces for a portrait generator, the AI will likely struggle to accurately generate images of individuals from other ethnic backgrounds, resulting in outputs that reinforce existing racial biases.
Similarly, if a dataset for architectural design predominantly includes images of traditional Western-style buildings, the AI might struggle to generate designs that reflect other architectural styles or cultural preferences, potentially leading to a homogenization of design aesthetics.
Manifestations of Bias in AI-Generated Designs
Biases embedded in training data manifest in several ways within AI-generated designs. One common manifestation is the skewed representation of certain demographics. For example, an AI-powered fashion design tool trained on a dataset predominantly featuring thin, white models might consistently generate designs that cater only to this specific body type and skin tone, effectively excluding individuals with different body shapes, sizes, and skin colors.
Another manifestation is the perpetuation of harmful stereotypes. An AI-generated image depicting a specific profession might consistently associate it with a particular gender or race, thereby reinforcing existing societal stereotypes. For instance, an AI-generated image depicting a doctor might disproportionately show a male doctor, thus reinforcing the stereotype of the male-dominated medical profession. Furthermore, AI could inadvertently exclude certain groups entirely from its generated outputs, such as by failing to generate designs that cater to individuals with disabilities.
This could range from clothing designs that don’t accommodate wheelchair users to architectural designs that neglect accessibility features.
Ethical Responsibility of Developers in Mitigating Bias
Developers bear a significant ethical responsibility to mitigate bias in AI design tools. This requires a multi-pronged approach starting with careful curation of training datasets. This involves actively seeking out and incorporating diverse and representative data to ensure a balanced representation of different demographics, ethnicities, body types, and abilities. Moreover, developers should employ techniques to identify and correct existing biases within the training data.
This could involve using bias detection algorithms to pinpoint potential biases and then implementing strategies to counteract them, such as data augmentation or re-weighting of samples. Furthermore, ongoing monitoring and evaluation of the AI’s outputs are essential to detect any emergent biases that may not have been apparent during the initial training phase. Transparency in the development process, including open documentation of the datasets and algorithms used, is also crucial for accountability and building public trust.
Strategies for Ensuring Diverse and Inclusive Representation
Ensuring diverse and inclusive representation in AI-generated design assets necessitates a proactive approach. One crucial strategy is the creation of diverse and representative training datasets. This involves actively seeking out and including data from underrepresented groups and ensuring that the dataset reflects the actual diversity of the population. Another important strategy is the use of bias mitigation techniques during the training process.
This includes employing algorithms designed to reduce bias, such as adversarial debiasing or fairness-aware learning. Furthermore, involving diverse teams of designers and experts in the development process is essential to identify and address potential biases from multiple perspectives. Finally, incorporating user feedback mechanisms allows for ongoing evaluation and improvement of the AI’s outputs, ensuring that the generated designs truly reflect the needs and preferences of a diverse user base.
This continuous feedback loop is vital for ensuring the ongoing fairness and inclusivity of AI-generated designs.
The Impact on Human Designers and the Creative Industry
The rise of AI-generated design assets presents a complex challenge to the creative industry, sparking debates about job displacement and the evolution of design roles. While concerns about automation replacing human designers are valid, the integration of AI also presents opportunities for enhanced creativity and new avenues for professional growth. Understanding the potential impacts, both positive and negative, is crucial for navigating this transformative period.The introduction of AI design tools necessitates a careful examination of their impact on the employment landscape for human designers.
The potential for job displacement exists, particularly for roles involving repetitive or easily automatable tasks. However, concurrently, AI is also fostering the creation of new roles and specializations within the design field.
Job Displacement and Creation in the Design Field, The ethical implications of AI-generated design assets
AI’s ability to rapidly generate design variations and automate certain processes undoubtedly raises concerns about job displacement for entry-level designers or those specializing in tasks readily handled by algorithms. For instance, AI can quickly generate multiple logo variations based on specified parameters, potentially reducing the need for human designers in this specific area. However, this automation frees up human designers to focus on higher-level tasks such as strategic design thinking, user experience (UX) research, and client interaction.
The demand for designers skilled in guiding AI tools, interpreting their output, and ensuring ethical and creative integrity is likely to increase. This shift mirrors historical technological advancements, where automation has altered but not eliminated job markets. The advent of desktop publishing software, for example, did not eliminate graphic designers; instead, it shifted their roles and responsibilities. Similarly, AI is poised to reshape, not necessarily replace, design professions.
Evolving Roles and Skills for Designers
The integration of AI demands a shift in the skillset required for success in the design industry. Human designers will increasingly need to become proficient in AI tool utilization, prompt engineering, and data analysis to effectively collaborate with AI. Critical thinking, problem-solving, and creative direction will become even more paramount, as designers will need to oversee the AI’s output, refine its suggestions, and infuse designs with uniquely human creativity and emotional intelligence.
Skills in areas such as human-computer interaction (HCI), ethical considerations in AI, and project management will also become highly valuable. The ability to leverage AI to enhance efficiency and explore innovative design solutions, rather than fearing its replacement capabilities, will be crucial for designers’ future success.
Collaboration Between Human Designers and AI Tools
Effective collaboration between human designers and AI tools is key to maximizing the benefits of this technological advancement. Designers can use AI tools to automate repetitive tasks like generating color palettes, creating initial mockups, or exploring various design iterations. This frees up time and mental energy to focus on the strategic aspects of the design process, such as defining project goals, understanding user needs, and crafting a compelling narrative.
For example, a designer might use an AI tool to generate several options for a website layout based on specific input parameters, then select the most promising options and refine them based on their creative vision and user research findings. The AI acts as a powerful assistant, augmenting the designer’s capabilities, rather than replacing them. Human oversight and judgment remain essential to ensure the final product aligns with the design brief and reflects the intended aesthetic and emotional impact.
A Hypothetical Training Program for Designers Adapting to AI
A comprehensive training program for designers adapting to the AI-driven design landscape should incorporate several key elements. The program would begin with foundational modules on AI fundamentals, covering basic concepts, common AI design tools, and responsible AI practices. Subsequent modules would delve into advanced techniques such as prompt engineering, data interpretation, and AI-assisted design workflows. Practical workshops would focus on hands-on experience with various AI tools, emphasizing collaborative workflows and ethical considerations.
The curriculum would also integrate modules on emerging design trends, user experience (UX) principles, and the business aspects of leveraging AI in design projects. Finally, a capstone project would allow participants to apply their newly acquired skills to a real-world design challenge, solidifying their understanding and practical abilities. This multifaceted approach ensures designers are well-equipped to thrive in the evolving creative landscape.
Transparency and Disclosure of AI-Generated Content
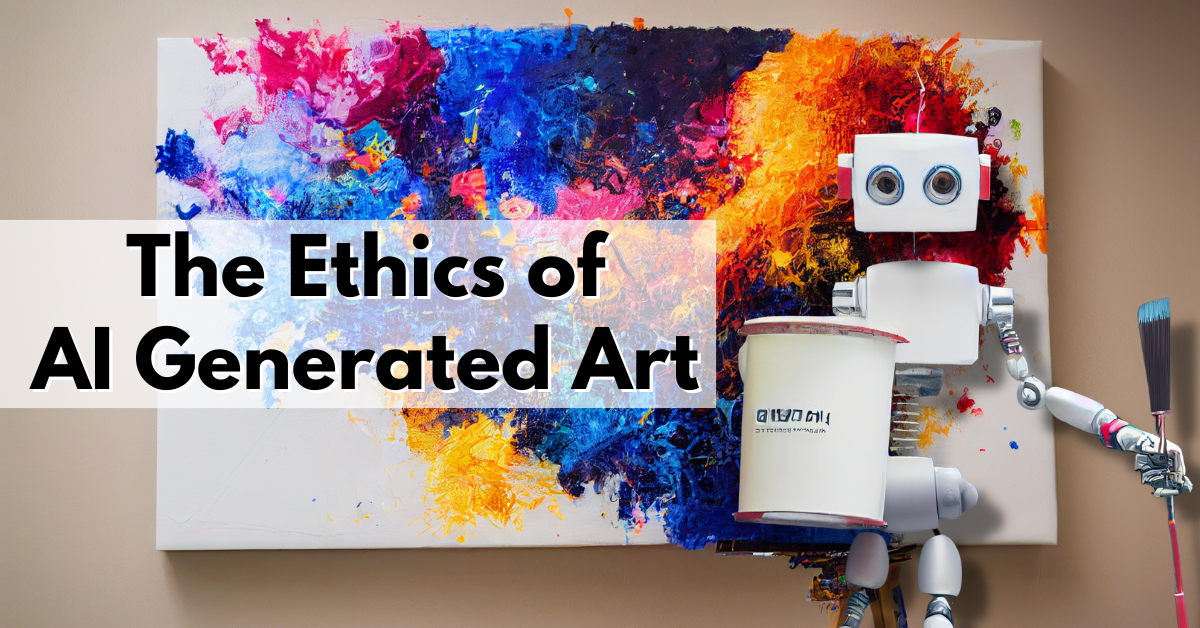
The ethical use of AI in design necessitates transparency. Failing to disclose the use of AI in creating design assets raises significant ethical concerns, impacting consumer trust, business reputation, and the overall creative landscape. Openness regarding AI’s role is not merely a matter of good practice; it’s a crucial element of responsible innovation.Transparency in the use of AI-generated design assets is paramount for building consumer trust and maintaining ethical standards within the creative industry.
Consumers have a right to know the origin of the designs they encounter, especially as AI-generated content becomes increasingly prevalent. This knowledge allows for informed decision-making and prevents potential deception or misrepresentation. Furthermore, transparency fosters accountability, allowing for scrutiny and critique of the AI’s output and its potential biases. Stakeholders, including designers, businesses, and regulators, need clear information to navigate the evolving legal and ethical landscape surrounding AI-generated content.
Consequences of Non-Disclosure
Non-disclosure of AI involvement in design carries significant risks for businesses. Failure to be transparent can erode consumer trust, leading to reputational damage and potential boycotts. Legally, undisclosed use of AI-generated content may infringe on copyright or intellectual property laws, depending on the specific circumstances and the underlying algorithms used. This can result in costly legal battles and penalties.
Furthermore, lack of transparency hinders the ability to address potential biases embedded within the AI algorithms, which could perpetuate harmful stereotypes or discriminatory practices. For example, a fashion company using AI to generate images without disclosing its use might inadvertently perpetuate unrealistic beauty standards, leading to negative social consequences. This lack of transparency also prevents effective oversight and regulation of AI in the design industry, potentially hindering the development of responsible AI practices.
A lack of transparency can stifle innovation by creating an environment of distrust and uncertainty.
Sample Disclosure Statement
A clear and concise disclosure statement is essential for communicating the use of AI in design. The statement should be easily accessible and understandable to the average consumer. Here’s an example of a suitable disclosure statement for a website showcasing AI-generated design work:
“The designs featured on this website were created with the assistance of artificial intelligence. While AI tools aided in the generation of these visuals, human designers were involved in the selection, refinement, and final approval of the designs. We believe in transparency and want to ensure you understand the role of technology in our creative process.”
This statement provides necessary context without overly complex technical jargon. It acknowledges the AI’s role while also emphasizing the human element in the creative process. Variations of this statement can be adapted to suit specific contexts and levels of AI involvement. The key is to be honest, straightforward, and readily accessible to the audience.
Environmental Impact of AI-Generated Design
The burgeoning field of AI-generated design, while offering unprecedented creative possibilities, raises significant concerns regarding its environmental footprint. The computational intensity of training and deploying these sophisticated algorithms necessitates substantial energy consumption, contributing to greenhouse gas emissions and overall environmental degradation. Understanding this impact is crucial for responsible development and implementation of AI design tools.The energy consumption associated with AI design tools stems primarily from two sources: the training phase and the operational phase.
Training large language models (LLMs) and generative adversarial networks (GANs) requires immense computational power, often involving thousands of powerful GPUs running for extended periods. This process consumes vast amounts of electricity, translating directly into a significant carbon footprint. The operational phase, while less energy-intensive than training, still involves significant computing resources to generate designs based on user prompts and parameters.
The cumulative effect of these energy demands across numerous users and applications poses a substantial environmental challenge.
Energy Consumption and Carbon Footprint of AI Design Models
The environmental impact of AI design models varies significantly depending on factors such as the model’s size and complexity, the training data used, the hardware employed, and the frequency of use. Studies have shown that training a single large language model can consume as much energy as a small city for several days, resulting in a considerable carbon emission equivalent to thousands of tons of CO2.
While precise figures remain challenging to obtain due to the proprietary nature of many AI models and the variability in hardware and training methodologies, the sheer scale of computational resources involved makes it clear that the energy consumption is substantial. For example, a study by researchers at the University of Massachusetts Amherst estimated that training a large language model can emit up to 5 times more CO2 than a typical car over its lifetime.
Comparison with Traditional Design Methods
Compared to traditional design methods, the environmental impact of AI-generated designs is a complex issue without a simple answer. Traditional design often involves material consumption, transportation, and waste generation. However, AI design, particularly in its current form, relies heavily on computational power, making its energy consumption a significant environmental factor. A direct comparison is difficult because the environmental impacts are expressed in different units (energy vs.
materials). However, the increasing prevalence of AI-driven design necessitates a comprehensive life-cycle assessment to accurately compare the overall environmental burdens of both approaches. This assessment would need to consider the energy consumed in AI training and operation, alongside the material use and waste generation associated with the final product design.
Strategies for Reducing the Environmental Impact of AI Design Tools
Several strategies can mitigate the environmental impact of AI design tools. One crucial aspect is improving the energy efficiency of the algorithms themselves. Research into more efficient training techniques and model architectures can significantly reduce energy consumption. Another approach involves optimizing the hardware used for training and deployment, leveraging more energy-efficient processors and data centers. Furthermore, employing renewable energy sources to power data centers and computational infrastructure is vital in reducing the carbon footprint.
The development and use of more efficient cooling systems for data centers can also contribute to energy savings.
Best Practices for Minimizing the Environmental Footprint of AI-Generated Design Workflows
To minimize the environmental footprint of AI-generated design workflows, several best practices can be adopted. These include:
- Selecting energy-efficient hardware for AI design tasks.
- Optimizing design workflows to minimize computational demands.
- Utilizing cloud computing services that prioritize renewable energy sources.
- Employing model compression techniques to reduce model size and computational requirements.
- Prioritizing the use of pre-trained models whenever possible, reducing the need for extensive retraining.
- Promoting the development and adoption of more energy-efficient AI algorithms.
Implementing these best practices will not only reduce the environmental impact of AI-generated design but also contribute to a more sustainable future for the creative industry as a whole.
Authenticity and Originality in AI-Generated Design
The rise of AI-powered design tools presents a fundamental challenge to our understanding of authenticity and originality in creative work. While human designers imbue their creations with personal experiences, intentions, and unique perspectives, AI algorithms generate designs based on learned patterns and data sets. This raises questions about the nature of creativity itself and the value we ascribe to artistic expression.
Determining the originality of an AI-generated design requires a nuanced approach, considering both the algorithm’s capabilities and the user’s input and direction.AI-generated designs differ significantly from human-created designs in their process of creation and the resulting expression of originality. Human designers typically undergo a process of ideation, experimentation, and refinement, guided by their individual artistic vision and emotional responses.
AI, on the other hand, generates designs through complex algorithms that analyze vast datasets, identifying trends and patterns to create novel combinations. While the output might be visually striking and innovative, the underlying process lacks the same level of conscious intentionality and emotional investment found in human-created work. This difference raises questions about whether AI can truly be considered “creative” in the same way a human artist is.
AI-Generated Designs Blurring the Lines Between Imitation and Innovation
Several examples demonstrate the ambiguity surrounding originality in AI-generated design. Consider an AI trained on a vast dataset of Renaissance paintings. It could generate a new artwork that incorporates stylistic elements reminiscent of Michelangelo or Raphael, but with novel color palettes or compositional arrangements. Is this a derivative work, a mere imitation, or an innovative synthesis of existing styles?
Similarly, AI tools can generate logos or website layouts based on user-defined parameters, resulting in designs that may resemble existing work but possess subtle, yet crucial, differences. The line between inspiration and outright copying becomes increasingly blurred, forcing us to reconsider traditional notions of copyright and originality. Another example would be an AI trained on architectural styles generating a design that incorporates elements of both gothic and modern architecture in a previously unseen combination.
While drawing from existing styles, the final product exhibits a unique aesthetic not explicitly present in its training data.
Assessing the Originality of AI-Generated Design Assets
Assessing the originality of an AI-generated design requires a multi-faceted approach. First, it’s crucial to understand the AI’s training data and the parameters used to generate the design. A design generated with highly specific constraints and minimal algorithmic freedom may exhibit less originality than one created with more open-ended parameters. Second, the novelty of the design should be evaluated.
Does it introduce new visual elements, compositional techniques, or stylistic approaches? Third, the design’s aesthetic impact should be considered. Does it evoke a unique emotional response or possess a distinctive visual character? Finally, the context of the design’s creation and intended use should be taken into account. A design intended for a specific commercial purpose may be deemed less original than a design created purely for artistic exploration.
A thorough analysis of these factors provides a more comprehensive assessment of the design’s originality and its potential contribution to the creative landscape.
End of Discussion
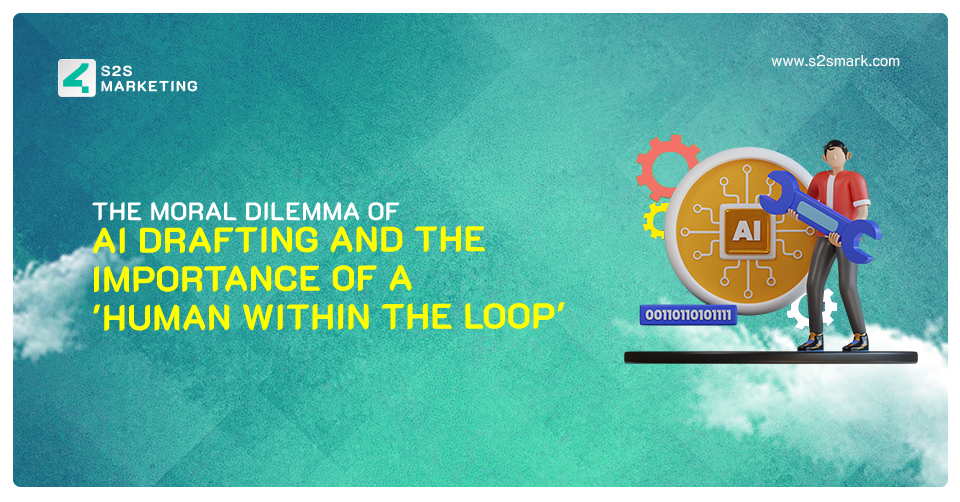
The rise of AI in design presents a paradigm shift, forcing a re-evaluation of established creative practices and legal frameworks. While AI offers unprecedented opportunities for efficiency and innovation, navigating its ethical complexities is paramount. By fostering transparency, addressing algorithmic bias, and proactively mitigating environmental concerns, we can harness the power of AI for good while safeguarding the integrity and inclusivity of the design industry.
The future of design hinges on responsible innovation and a thoughtful approach to the ethical implications of this powerful new technology.