How AI can automate UI UX design tasks is no longer a futuristic fantasy; it’s a rapidly evolving reality. This exploration delves into the current capabilities and future potential of artificial intelligence in streamlining the UI/UX design process, examining both the significant advantages and inherent limitations. We’ll uncover how AI assists in various stages, from initial wireframing and prototyping to sophisticated A/B testing and user feedback analysis, ultimately impacting the speed, efficiency, and inclusivity of design workflows.
Prepare to discover how this technological leap is reshaping the landscape of user experience design.
This journey will cover specific AI tools and techniques currently employed, addressing the ethical considerations and potential biases that may arise. We will also look ahead, speculating on emerging trends and the transformative impact AI will have on the role of human designers in the years to come. The goal is to provide a comprehensive understanding of AI’s current contribution and its projected influence on the future of UI/UX.
AI-Powered UI/UX Design Automation
The integration of artificial intelligence (AI) into UI/UX design workflows is rapidly evolving, transitioning from a niche exploration to a progressively impactful toolset. While complete automation remains a distant prospect, AI is already streamlining numerous aspects of the design process, offering significant potential for increased efficiency and improved design outcomes. This exploration delves into the current capabilities, advantages, and limitations of AI in UI/UX design automation.
AI-Powered UI/UX Design Automation: Current State
Current AI applications in UI/UX design primarily focus on automating repetitive tasks and augmenting human creativity. Tools leveraging machine learning algorithms can generate design variations based on input parameters, analyze user data to inform design decisions, and even automate aspects of prototyping and testing. Examples include AI-powered tools that suggest color palettes, generate layouts based on specified content, and provide automated feedback on design usability.
However, these tools are generally best utilized as assistive technologies, enhancing the designer’s capabilities rather than replacing them entirely. The level of sophistication varies widely across available tools, with some offering highly specialized functionalities while others provide a more generalized approach to design assistance.
Benefits of AI in UI/UX Design
The potential benefits of incorporating AI into UI/UX workflows are substantial. Firstly, AI can significantly accelerate the design process by automating time-consuming tasks such as generating design variations, creating mockups, and performing initial usability testing. This allows designers to focus on higher-level creative and strategic decisions. Secondly, AI can enhance design quality by providing data-driven insights into user preferences and behavior.
By analyzing user data, AI can identify patterns and trends that inform design choices, leading to more user-centered and effective designs. Thirdly, AI can improve design consistency across multiple platforms and devices by automating the adaptation of designs to different screen sizes and resolutions. This ensures a seamless user experience across all touchpoints.
Limitations and Challenges of AI-Driven UI/UX Tools
Despite the considerable potential, AI-driven UI/UX tools face several limitations. Current AI models often lack the nuanced understanding of human creativity and aesthetics necessary to produce truly innovative and groundbreaking designs. Over-reliance on AI can stifle the designer’s own creative process and lead to homogenized designs. Furthermore, the quality of AI-generated designs is heavily dependent on the quality and quantity of the input data.
Biased or incomplete data can lead to biased or flawed design outcomes. Finally, ethical considerations surrounding data privacy and algorithmic bias must be carefully addressed when utilizing AI in UI/UX design. Ensuring transparency and accountability in AI-driven design processes is crucial.
Comparison of Traditional and AI-Assisted UI/UX Design Processes
The following table highlights the differences between traditional and AI-assisted UI/UX design processes:
Task | Traditional Method | AI-Assisted Method | Time Savings (Estimate) |
---|---|---|---|
Logo Design | Manual sketching, refinement, and digitalization. Multiple iterations and client feedback loops. | AI-powered generation of logo variations based on input parameters (e.g., s, style preferences). Human refinement still required. | 20-40% |
Wireframing | Manual creation of wireframes using design software. | AI-powered generation of wireframes based on content and specified requirements. Human review and adjustment necessary. | 30-50% |
Usability Testing | Manual testing with users, analysis of feedback, and iterative design adjustments. | AI-powered analysis of user behavior during testing, providing automated feedback on usability issues. | 15-30% |
Color Palette Selection | Manual selection based on design principles and aesthetic considerations. | AI-powered suggestion of color palettes based on design style and target audience. | 10-20% |
AI for Automating Specific UI/UX Tasks
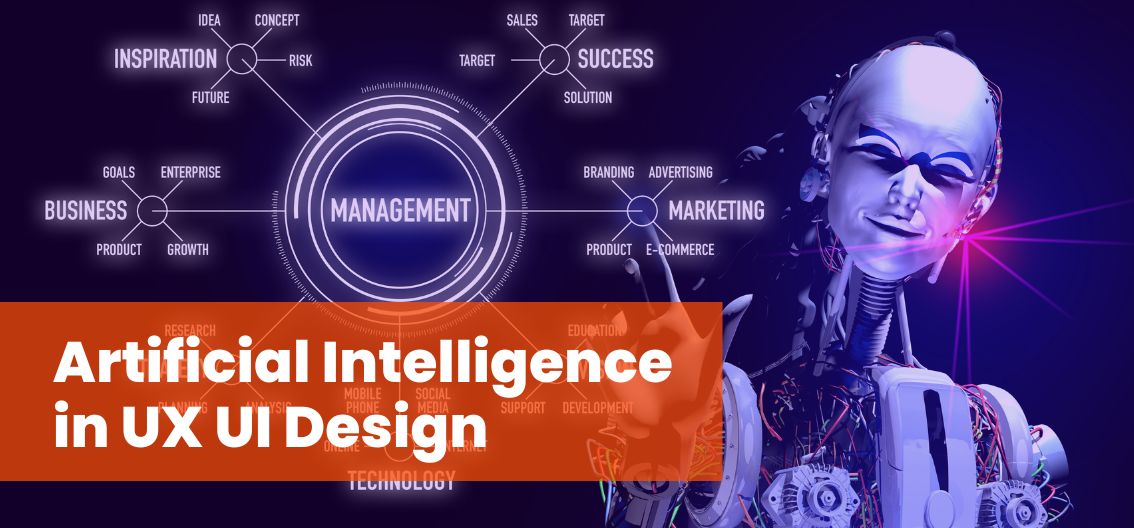
Artificial intelligence is rapidly transforming the UI/UX design landscape, automating previously time-consuming and labor-intensive tasks. This allows designers to focus on higher-level strategic thinking and creative problem-solving, ultimately leading to faster iteration cycles and improved design quality. The automation of specific tasks, powered by AI, is not about replacing designers, but rather augmenting their capabilities and efficiency.
AI-Driven Automation of Wireframing and Prototyping
AI significantly streamlines the wireframing and prototyping stages. Tools leverage machine learning to generate initial wireframes based on user input, such as descriptions of the desired functionality or even hand-drawn sketches. These AI-generated wireframes serve as a starting point, allowing designers to quickly iterate and refine the design. Furthermore, AI can assist in the creation of interactive prototypes, automatically generating transitions and animations based on pre-defined rules or learned patterns from existing successful designs.
This accelerates the prototyping process, enabling faster testing and feedback incorporation. For example, an AI-powered tool might automatically generate a navigation menu based on the defined sections of a website, saving designers considerable time.
Examples of AI Tools for Generating Design Variations
Several AI tools are available that generate design variations based on input parameters or existing designs. These tools utilize generative design algorithms to explore a wide range of design options, offering designers a diverse palette of choices to select from. For instance, tools can automatically generate multiple versions of a button design, varying color schemes, shapes, and text styles.
Another example would be generating multiple layouts for a product page, each with a different arrangement of images and text, all based on user-provided constraints and preferences. These tools effectively accelerate the exploration of design space, leading to more innovative and effective designs. One could imagine a tool generating variations of a mobile app’s home screen, automatically adjusting the placement of icons and information based on established best practices and user experience principles.
AI’s Role in A/B Testing and User Feedback Analysis, How AI can automate UI UX design tasks
AI plays a crucial role in optimizing designs through A/B testing and user feedback analysis. AI-powered platforms can automatically manage A/B tests, analyzing the performance of different design variations and identifying the most effective options based on key metrics such as click-through rates and conversion rates. Beyond A/B testing, AI can analyze qualitative user feedback from surveys, user interviews, and usability testing sessions, identifying recurring themes and pain points.
This automated analysis helps designers understand user preferences and frustrations, leading to more informed design decisions. For example, an AI system could analyze user comments on a website’s search functionality, highlighting recurring issues such as slow loading times or unclear search results. This data would then inform design improvements.
Hypothetical Workflow Showcasing AI’s Role in a UI/UX Project
A hypothetical UI/UX project for a new e-commerce app could utilize AI in the following workflow: The project begins with the designer outlining the app’s core functionalities and target audience. An AI-powered tool then generates initial wireframes based on this input. The designer refines these wireframes, and the AI tool automatically generates interactive prototypes. A/B testing is then conducted using an AI-powered platform, comparing different versions of the app’s home screen.
Finally, user feedback is collected and analyzed using AI, providing insights for further design iterations. This streamlined process significantly reduces development time and improves the overall quality of the final design. The iterative process, enhanced by AI-driven analysis, ensures a user-centric approach throughout the design process.
AI and User Research in UI/UX Design
Integrating artificial intelligence into user research dramatically accelerates the process and provides deeper insights than traditional methods. AI’s ability to process vast datasets allows for a more nuanced understanding of user behavior, preferences, and pain points, ultimately leading to more effective and user-centered designs. This section explores the applications of AI in user research, ethical considerations, and its potential for personalization.AI offers several powerful methods for analyzing user data and feedback, moving beyond simple trend identification to uncover complex patterns and relationships.
AI-Driven User Data Analysis Methods
AI algorithms, particularly machine learning models, can analyze diverse data sources such as user surveys, website analytics, app usage data, and social media comments. Natural Language Processing (NLP) techniques allow AI to understand and interpret textual feedback, identifying sentiment, key themes, and recurring issues. For example, sentiment analysis can gauge the overall positivity or negativity of user reviews, while topic modeling can identify the most frequently discussed aspects of a product or service.
This granular level of analysis provides a richer understanding of user experiences than manual analysis could achieve. Furthermore, AI can correlate different data sources to reveal hidden connections. For instance, linking website analytics showing high bounce rates on a specific page with negative sentiment expressed in user reviews on that same page points to a clear area for improvement.
Identifying User Pain Points and Preferences with AI
By analyzing user data, AI can pinpoint specific areas of friction within the user experience. For instance, analyzing heatmaps generated from user interaction data can highlight sections of a webpage or app that users consistently struggle with or ignore. Similarly, AI can identify patterns in user behavior that indicate frustration or confusion. By analyzing user journeys, AI can reveal bottlenecks and inefficiencies.
This allows designers to focus their efforts on addressing the most pressing issues and improving the overall user experience. Moreover, AI can identify user preferences by analyzing choices and behaviors. For instance, A/B testing powered by AI can determine which design elements or features resonate most strongly with users, guiding designers towards more effective design decisions.
Ethical Considerations of AI in User Research
The use of AI in user research raises several ethical concerns. Data privacy is paramount. Ensuring user data is collected, stored, and used responsibly and in compliance with relevant regulations (like GDPR and CCPA) is critical. Bias in algorithms is another major concern. AI models are trained on data, and if that data reflects existing societal biases, the AI will likely perpetuate and even amplify those biases.
This can lead to unfair or discriminatory outcomes. For example, an AI model trained on data predominantly from one demographic might produce insights that are not representative of the broader user base. Transparency and explainability are essential. Users should understand how their data is being used and how AI is informing design decisions. The potential for manipulation is another ethical consideration.
AI can be used to personalize user experiences to such an extent that it influences user behavior in ways that are not fully transparent or in the user’s best interest.
AI-Powered Personalization of User Experiences
AI facilitates highly personalized user experiences by tailoring content, features, and interactions to individual users based on their data profiles. For example, AI can recommend products or services relevant to a user’s past purchases or browsing history, or it can adjust the interface layout based on user preferences. This personalization enhances user engagement and satisfaction. Netflix’s recommendation engine is a prime example; it uses AI to analyze user viewing habits and suggest shows and movies they are likely to enjoy.
Similarly, many e-commerce platforms use AI to personalize product recommendations and shopping experiences.
Potential Biases in AI-Driven User Research
Several potential biases can be introduced by AI algorithms in user research.
- Sampling Bias: If the data used to train the AI model is not representative of the target user population, the resulting insights will be skewed.
- Algorithmic Bias: Pre-existing biases in the data used to train the AI can be amplified, leading to unfair or discriminatory outcomes.
- Confirmation Bias: AI might inadvertently reinforce existing assumptions or hypotheses, potentially overlooking alternative explanations.
- Measurement Bias: The way data is collected and measured can introduce bias into the results.
- Interpretation Bias: The way AI-generated insights are interpreted by human researchers can introduce bias.
Addressing these biases requires careful data selection, rigorous algorithm testing, and critical interpretation of AI-generated insights. Human oversight remains crucial to ensure ethical and responsible use of AI in user research.
AI in Accessibility and Inclusive Design
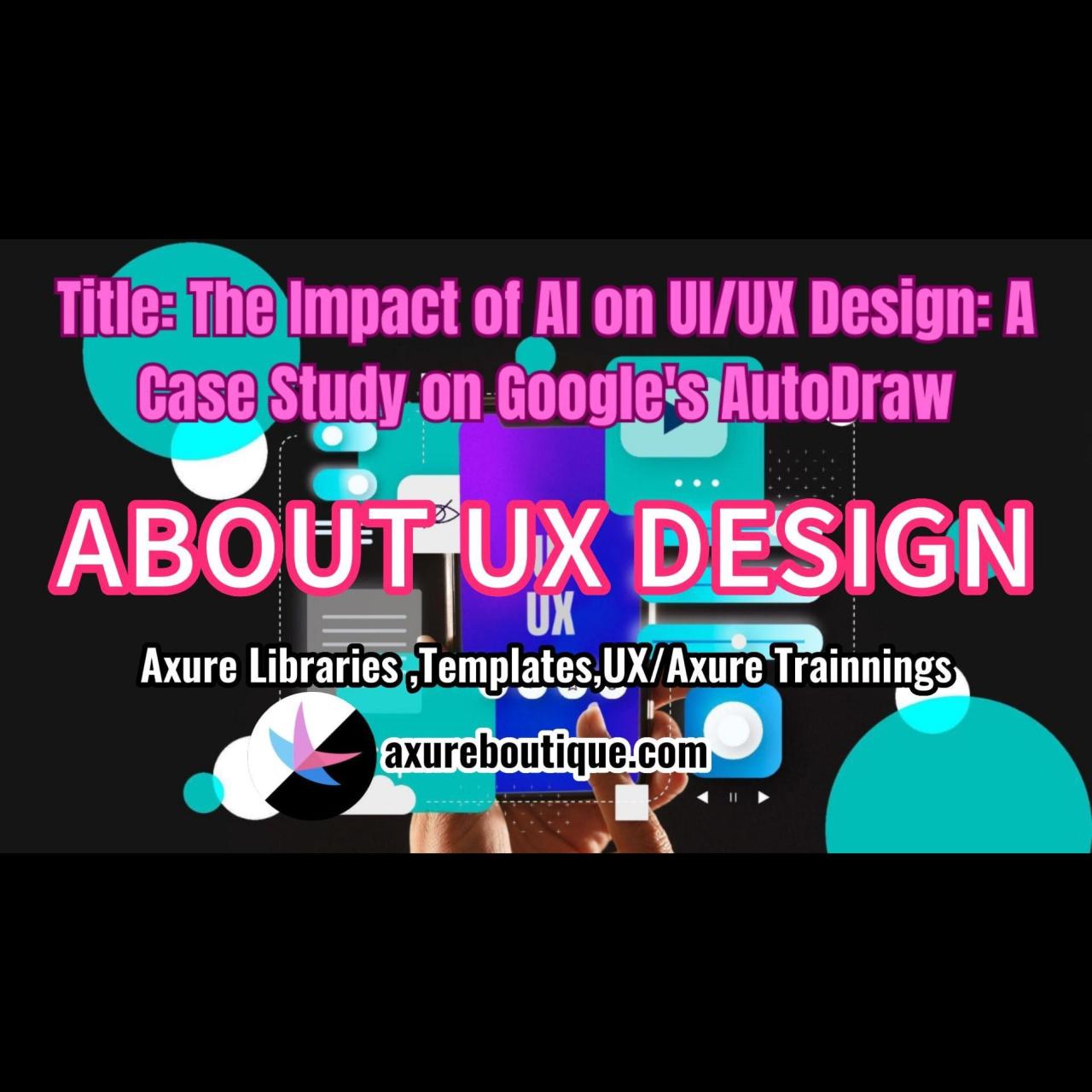
AI is rapidly transforming UI/UX design, and its impact on accessibility and inclusive design is particularly significant. By automating tasks, analyzing data, and offering personalized recommendations, AI empowers designers to create interfaces usable by individuals with diverse abilities and needs, fostering a more equitable digital experience for everyone. This section explores the multifaceted role of AI in promoting accessibility and inclusivity.AI’s contribution to accessible design goes beyond simply automating existing processes.
It enables the creation of genuinely inclusive interfaces by identifying and mitigating accessibility barriers proactively. This proactive approach shifts the focus from reacting to accessibility issues to preventing them in the first place, leading to more user-friendly and inclusive products from the outset. This is achieved through sophisticated algorithms that analyze designs for compliance with accessibility guidelines and identify potential usability problems for users with various disabilities.
AI-Driven Accessibility Testing Automation
AI significantly accelerates and enhances accessibility testing. Traditional methods are often time-consuming and labor-intensive, relying on manual checks against accessibility guidelines like WCAG. AI-powered tools, however, can automate many of these checks, analyzing website code and visual elements to identify potential accessibility issues such as missing alt text for images, insufficient color contrast, or keyboard navigation problems. This automated testing process allows designers to quickly identify and rectify accessibility flaws early in the design process, reducing development costs and improving the overall user experience.
For example, tools can analyze screen reader compatibility, ensuring that visually impaired users can navigate and understand the interface effectively. Automated testing also ensures consistent accessibility across different platforms and devices.
Examples of AI Tools for Inclusive Design
Several AI-powered tools are emerging to assist designers in creating more inclusive interfaces. These tools leverage machine learning to analyze design elements, identify potential accessibility issues, and suggest improvements. For instance, some tools automatically generate alt text for images based on their content, while others analyze color contrast ratios to ensure sufficient readability for users with low vision.
Other tools can automatically generate captions and transcripts for videos, making them accessible to deaf and hard-of-hearing users. These AI-driven solutions not only improve efficiency but also increase the accuracy and consistency of accessibility implementation. These tools often integrate directly into existing design workflows, minimizing disruption and maximizing designer productivity.
Improving User Experience for People with Disabilities
AI can significantly enhance the user experience for people with disabilities. For example, AI-powered personalization features can adapt the interface to individual user needs and preferences. This might involve adjusting font sizes, colors, and layouts based on user-reported visual impairments or cognitive limitations. AI-driven speech recognition and text-to-speech technologies can provide alternative input and output methods for users with motor impairments or visual impairments.
Furthermore, AI can analyze user behavior to identify areas of difficulty and suggest improvements to the interface, leading to a more intuitive and accessible experience for all users. AI-powered predictive text and auto-completion features can also assist users with cognitive or motor impairments in completing tasks more efficiently.
Best Practices for Incorporating AI in Accessible Design
It’s crucial to adopt best practices when integrating AI into accessible design. AI should augment, not replace, human expertise in accessibility.
- Prioritize human oversight: AI tools should be used as aids, not as replacements for human judgment and expertise in accessibility. Designers must review and validate AI-generated suggestions.
- Focus on user feedback: Regularly collect user feedback from people with disabilities to ensure that AI-driven design improvements are effective and meet their needs.
- Maintain transparency: Clearly communicate to users how AI is being used to personalize or improve their experience.
- Ensure data privacy: Handle user data responsibly and ethically, complying with all relevant privacy regulations.
- Stay updated: The field of AI and accessibility is constantly evolving. Stay informed about the latest tools and best practices.
The Future of AI in UI/UX Design
The integration of artificial intelligence into UI/UX design is still in its nascent stages, yet its potential to revolutionize the field is undeniable. Current applications focus on automating specific tasks, but the coming years promise a far more profound transformation, impacting not only the design process but also the very nature of user interfaces themselves. We can expect AI to move beyond assisting designers to becoming a collaborative partner, and even a primary creative force in certain aspects of design.
AI’s increasing sophistication will lead to significant advancements in UI/UX design. This evolution will be characterized by a shift from task automation to more strategic and creative applications, resulting in more efficient and user-centered designs. The human designer’s role will adapt, focusing on higher-level strategic decisions, creative direction, and ethical considerations, while AI handles the more repetitive and computationally intensive tasks.
AI-Driven Hyper-Personalization
AI will enable unprecedented levels of user interface personalization. Imagine a website or app that dynamically adjusts its layout, content, and even visual style based on a user’s real-time behavior, preferences, and context. This goes beyond simple A/B testing; AI will analyze vast datasets of user interactions to predict optimal design choices for each individual, leading to significantly improved user engagement and satisfaction.
This level of personalization could be seen in e-commerce platforms tailoring product recommendations and layouts in real-time, or in educational apps adapting difficulty and content based on individual learning styles.
AI-Generated Design Exploration
Current AI tools can generate design variations based on input parameters. The future will see a significant leap in their creative capabilities. AI could explore a far wider range of design possibilities than a human designer could manually, identifying novel solutions and pushing the boundaries of conventional design thinking. This could involve AI generating completely new UI patterns or interaction models, leading to more intuitive and efficient interfaces.
For example, an AI could explore hundreds of different navigation structures for a complex application, identifying the most efficient and user-friendly option based on simulated user testing.
AI’s Impact on the Designer’s Role
The integration of AI will not replace human designers but will significantly alter their roles. Designers will become strategic thinkers, focusing on defining design goals, understanding user needs, and ensuring ethical considerations are addressed. AI will handle the tedious aspects of design, freeing up designers to focus on higher-level creative and strategic tasks. This shift will necessitate upskilling and reskilling for designers, emphasizing critical thinking, creative problem-solving, and ethical AI implementation.
Designers will need to learn to collaborate effectively with AI tools, guiding them, interpreting their output, and ensuring the final product aligns with overall design vision and ethical standards.
Emergence of Novel Design Paradigms
AI’s influence may lead to entirely new design paradigms. For example, AI could generate adaptive interfaces that seamlessly change based on the user’s emotional state, detected through biometric data. This could lead to more empathetic and supportive user experiences. Similarly, AI could facilitate the creation of interfaces that transcend traditional screen-based interactions, using augmented reality or brain-computer interfaces to create immersive and intuitive experiences.
The potential for AI to create entirely new interaction models, perhaps even those we cannot currently conceive, is immense.
Visual Representation of AI’s Evolution in UI/UX
The visual representation would be a graph charting the evolution of AI’s role in UI/UX over the next 5-10 years. The X-axis would represent time, and the Y-axis would represent the level of AI involvement, ranging from “Task Automation” at the bottom to “Collaborative Partner/Primary Creative Force” at the top. The graph would show a gradual upward trend, initially a slow incline representing the current phase of task automation, then a steeper incline representing the increasing involvement of AI in more complex design decisions and creative processes.
The line would not be perfectly smooth, reflecting the iterative and sometimes unpredictable nature of technological development. Specific milestones (e.g., widespread adoption of AI-powered design tools, emergence of AI-generated design paradigms) could be marked on the graph. The overall message would be one of increasing AI involvement and collaboration, not replacement, of human designers.
Final Conclusion: How AI Can Automate UI UX Design Tasks
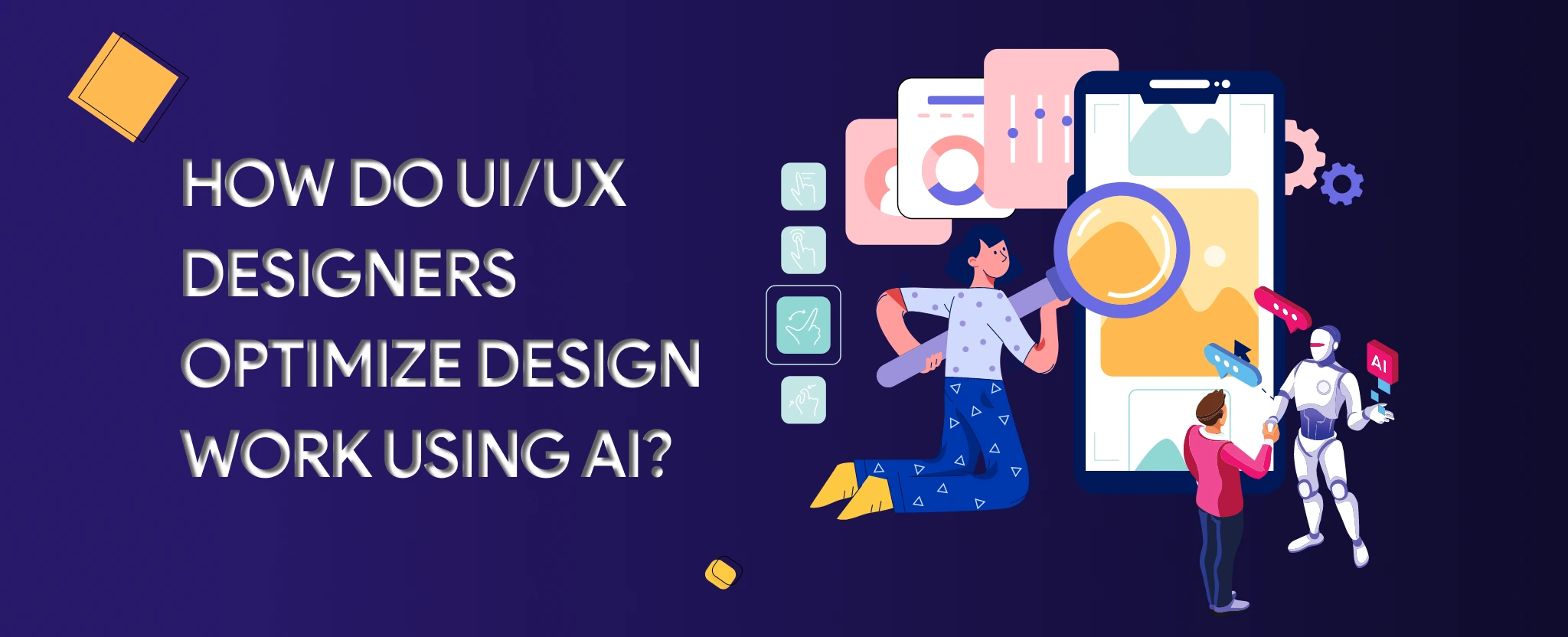
The integration of AI in UI/UX design isn’t about replacing human creativity; it’s about augmenting it. By automating repetitive tasks and providing data-driven insights, AI empowers designers to focus on higher-level strategic thinking, innovation, and the creation of truly exceptional user experiences. While challenges remain, the future trajectory points towards a collaborative partnership between human ingenuity and artificial intelligence, leading to a more efficient, inclusive, and user-centric design process than ever before imagined.
The potential for innovation is vast, and the journey is just beginning.